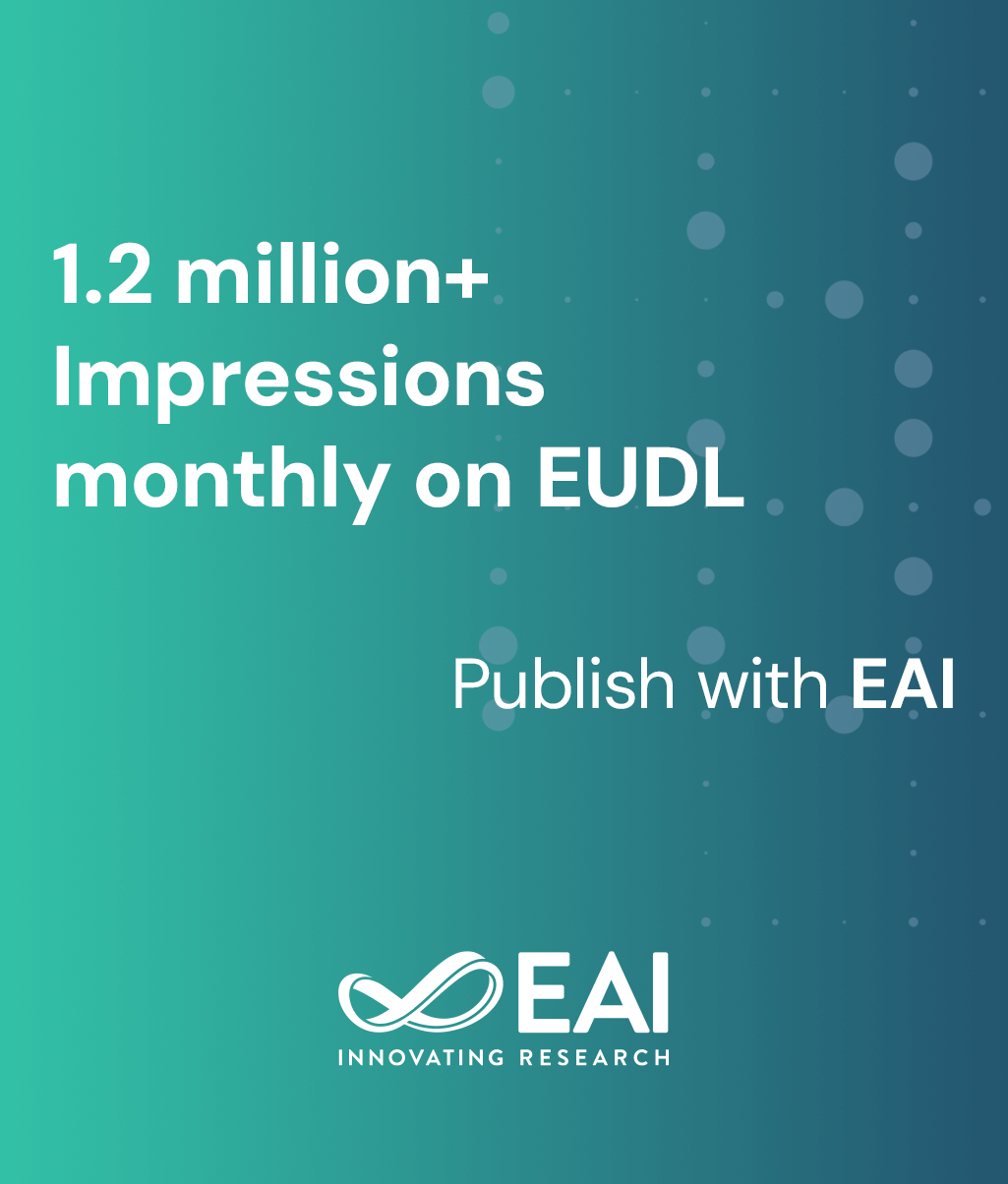
Research Article
Classification of Skin Lesions Based on Data Collaboration Under Imbalance Dataset
@INPROCEEDINGS{10.1007/978-3-030-30146-0_20, author={Weijia Ji and Lizhi Cai and Mingang Chen and Naiqi Wang}, title={Classification of Skin Lesions Based on Data Collaboration Under Imbalance Dataset}, proceedings={Collaborative Computing: Networking, Applications and Worksharing. 15th EAI International Conference, CollaborateCom 2019, London, UK, August 19-22, 2019, Proceedings}, proceedings_a={COLLABORATECOM}, year={2019}, month={8}, keywords={Imbalance data Generative adversarial network Data collaboration Skin image classification}, doi={10.1007/978-3-030-30146-0_20} }
- Weijia Ji
Lizhi Cai
Mingang Chen
Naiqi Wang
Year: 2019
Classification of Skin Lesions Based on Data Collaboration Under Imbalance Dataset
COLLABORATECOM
Springer
DOI: 10.1007/978-3-030-30146-0_20
Abstract
Imbalance data is a common problem in machine learning task, which often impacts the accuracy of models. An effective way to solve it is to increase the number of minority class samples in the dataset. Many methods are put forward to solve the problem of imbalance data in machine learning. But these are all for low-dimensional data. For high-dimensional data, such as images, these methods are not well applicable. In this paper, an image generation method based on generative adversarial network is introduced to do pattern learning for samples of minority class in the dataset, so as to realize the expansion of data for minority class. And finally the classification networks for skin lesions are trained by data collaboration which consist of real images and generated images. The experimental results indicate that the accuracy of networks are further improved by the addition of generated images while alleviating the imbalance problem to some extent.