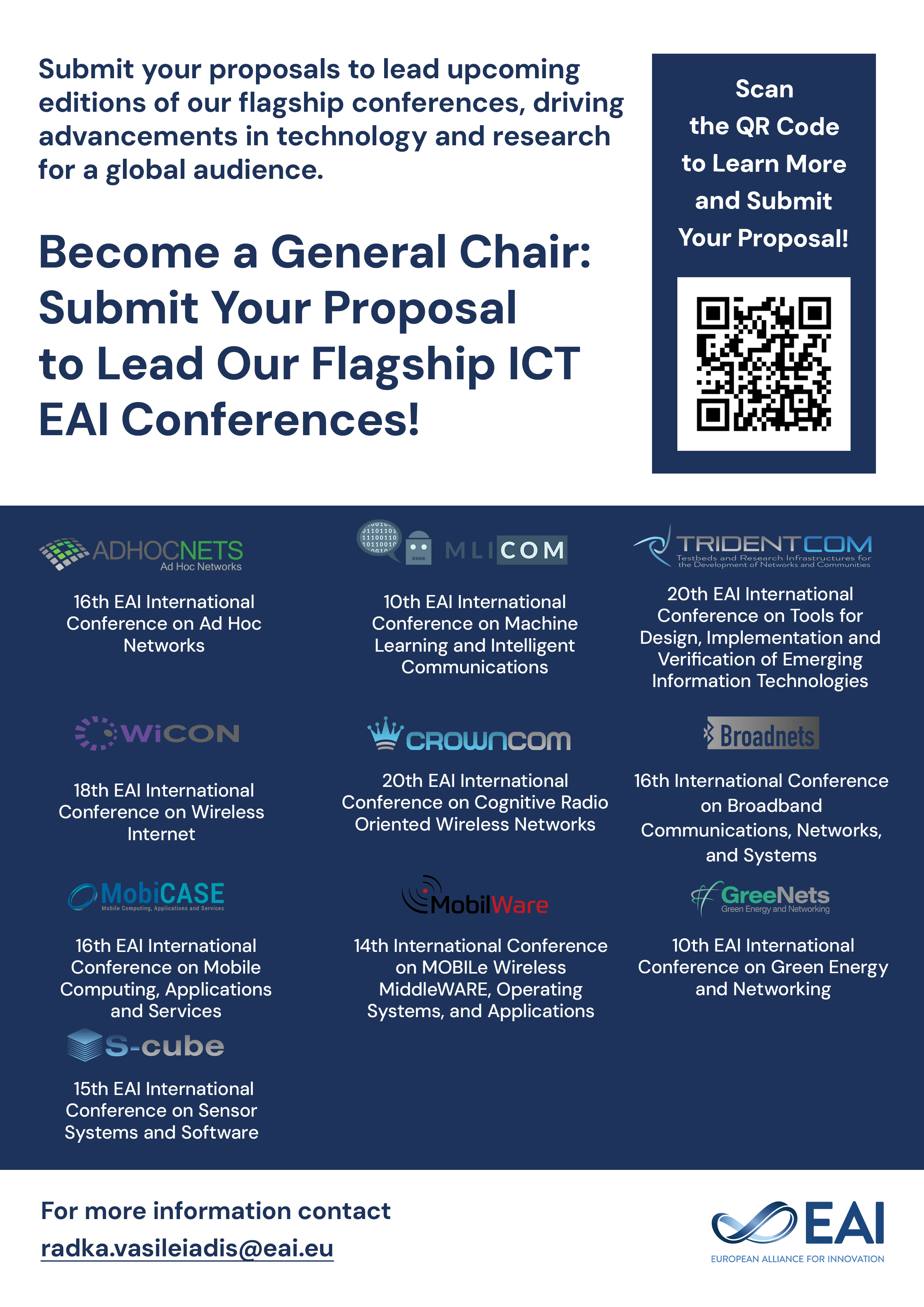
Research Article
A Dynamic Difficulty-Sensitive Worker Distribution Model for Crowdsourcing Quality Management
@INPROCEEDINGS{10.1007/978-3-030-30146-0_2, author={Miao Zheng and Lizhen Cui and Wei He and Wei Guo and Xudong Lu}, title={A Dynamic Difficulty-Sensitive Worker Distribution Model for Crowdsourcing Quality Management}, proceedings={Collaborative Computing: Networking, Applications and Worksharing. 15th EAI International Conference, CollaborateCom 2019, London, UK, August 19-22, 2019, Proceedings}, proceedings_a={COLLABORATECOM}, year={2019}, month={8}, keywords={Crowdsourcing quality management Worker quality distribution model Maximum likelihood estimation}, doi={10.1007/978-3-030-30146-0_2} }
- Miao Zheng
Lizhen Cui
Wei He
Wei Guo
Xudong Lu
Year: 2019
A Dynamic Difficulty-Sensitive Worker Distribution Model for Crowdsourcing Quality Management
COLLABORATECOM
Springer
DOI: 10.1007/978-3-030-30146-0_2
Abstract
Crowdsourcing utilizes the intelligence of people to solve problems that are difficult for machines such as entity resolution, sentiment analysis and image recognition. In crowdsourcing systems, requesters publish tasks that are answered by workers. However, the responses collected from the crowd are ambiguous as the workers on internet with unknown and very diverse abilities, skills, interests and knowledge background. In order to ensure the quality of crowdsourcing results, it is important to characterize worker quality accurately. Many previous works model the worker quality by a fixed value (such as probability value or confusion matrix). But even when workers complete the same type of tasks, the quality is affected by some factors (task difficulty) to varying degrees. Here we propose a dynamic difficulty-sensitive worker quality distribution model. In our model, the worker’s ability is affected by task difficulty and fits a functional distribution. This model reflects the relationship between worker reliability and task difficulty. In addition, we utilize Expectation-Maximization approach (EM) to obtain maximum likelihood estimates of the parameters of worker quality distribution model and the true answers to the tasks. We conduct extensive experiments with synthetic data and real-world data. The experimental results show that our method significantly outperforms other state-of-the-art approaches.