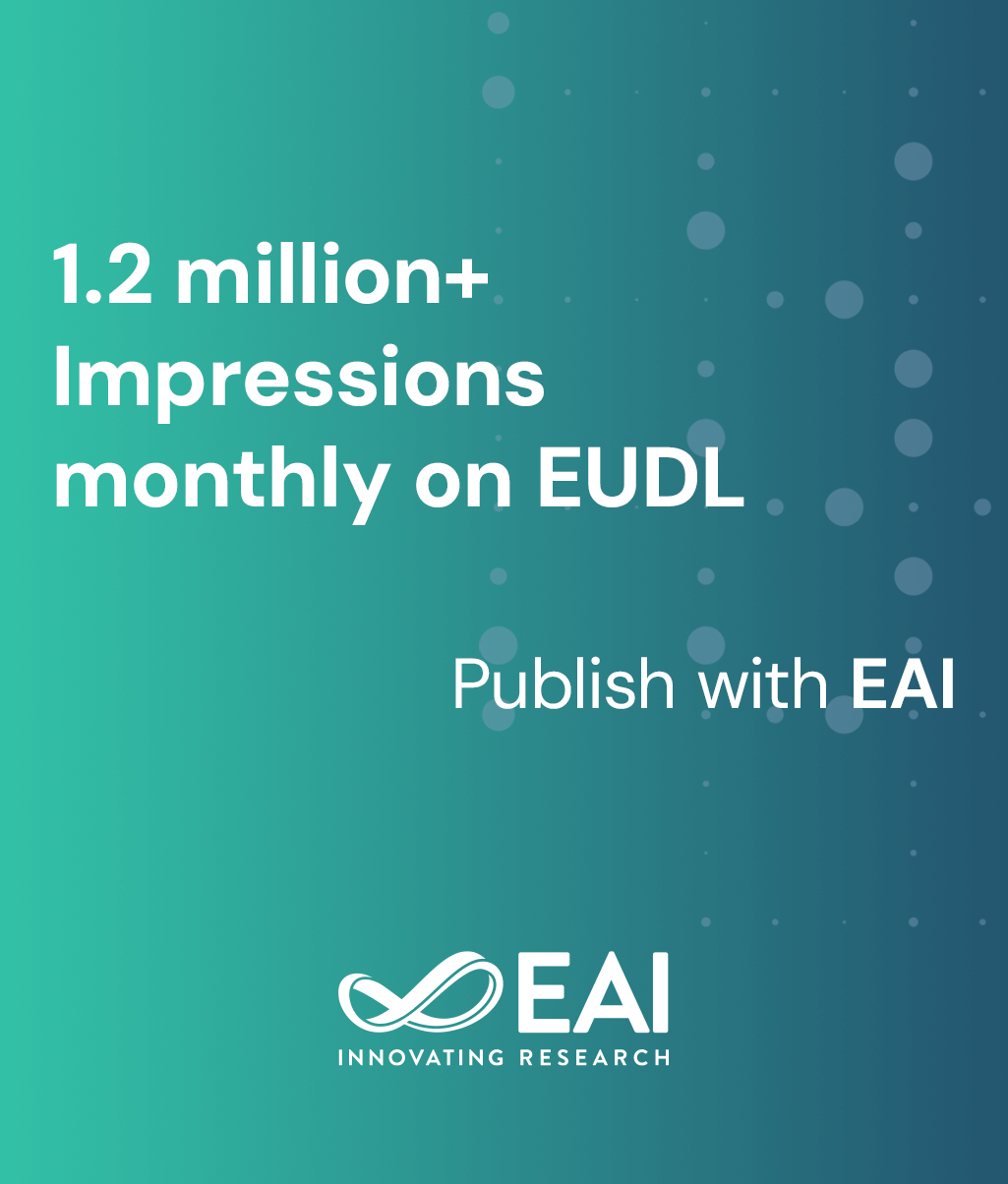
Research Article
Attention-Based Bilinear Joint Learning Framework for Entity Linking
@INPROCEEDINGS{10.1007/978-3-030-30146-0_17, author={Min Cao and Penglong Wang and Honghao Gao and Jiangang Shi and Yuan Tao and Weilin Zhang}, title={Attention-Based Bilinear Joint Learning Framework for Entity Linking}, proceedings={Collaborative Computing: Networking, Applications and Worksharing. 15th EAI International Conference, CollaborateCom 2019, London, UK, August 19-22, 2019, Proceedings}, proceedings_a={COLLABORATECOM}, year={2019}, month={8}, keywords={Entity linking Embedding model Modeling context Modeling coherence Entity disambiguation}, doi={10.1007/978-3-030-30146-0_17} }
- Min Cao
Penglong Wang
Honghao Gao
Jiangang Shi
Yuan Tao
Weilin Zhang
Year: 2019
Attention-Based Bilinear Joint Learning Framework for Entity Linking
COLLABORATECOM
Springer
DOI: 10.1007/978-3-030-30146-0_17
Abstract
Entity Linking (EL) is a task that links entity mentions in the text to corresponding entities in a knowledge base. The key to building a high-quality EL system involves accurate representations of word and entity. In this paper, we propose an attention-based bilinear joint learning framework for entity linking. First, a novel encoding method is employed for coding EL. This method jointly learns words and entities using an attention mechanism. Next, for ranking features, a weighted summation model is introduced to model the textual context and coherence. Then, we employ a pairwise boosting regression tree (PBRT) to rank candidate entities. As input, PBRT takes both features constructed with a weighted summation model and conventional EL features. Finally, through the experiment, we demonstrate that the proposed model learns embedding efficiently and improves the EL performance compared with other state-of-the-art methods. Our approach achieves superior result on two standard EL datasets: CoNLL and TAC 2010.