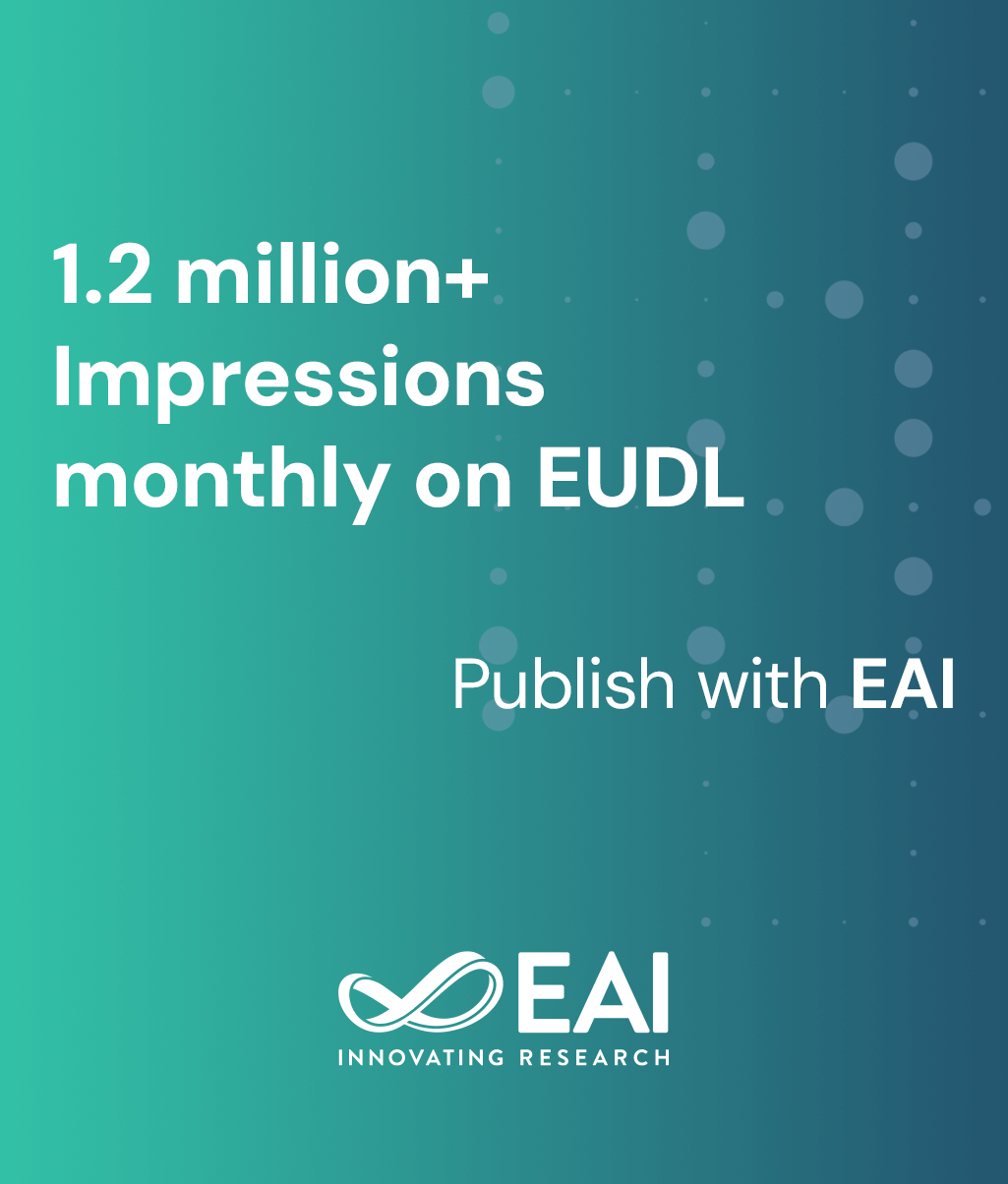
Research Article
Noise Sensing Calibration Under Different Phone Context
@INPROCEEDINGS{10.1007/978-3-030-28468-8_2, author={Min Huang and Lina Chen}, title={Noise Sensing Calibration Under Different Phone Context}, proceedings={Mobile Computing, Applications, and Services. 10th EAI International Conference, MobiCASE 2019, Hangzhou, China, June 14--15, 2019, Proceedings}, proceedings_a={MOBICASE}, year={2019}, month={9}, keywords={Mobile sensors Noise sensing Phone context}, doi={10.1007/978-3-030-28468-8_2} }
- Min Huang
Lina Chen
Year: 2019
Noise Sensing Calibration Under Different Phone Context
MOBICASE
Springer
DOI: 10.1007/978-3-030-28468-8_2
Abstract
Noise pollution severely threatens human well-being. Constructing a noise map based on crowd-sensing can help city planners better understand environmental noise at lower cost. Based on the strict sampling method limitation of state-of-the-art techniques, we build a new calibration model aimed at the pocket situations and the scenarios happened more frequently in actual life. The proposed model consists of a Activity Recognition Model (ARM) and a Signal Processing Model (SPM). Three types of data are taken into consideration, which are sitting, standing, and walking. In ARM, we collect 3-axis accelerometer data to identify current sampling context based on the convolutional neural network. SPM mainly implements noise level measurement and calibration according to the corresponding output of ARM under different phone context. The average errors after calibration are controlled to be within ±3 dB(A), and the classification precision reaches 99.2%. Finally, we display the noise map adopting different criteria based on the building types, which is more scientific and meaningful. The final results show that our proposed calibration model is feasible and can improve the data quality under different situations.