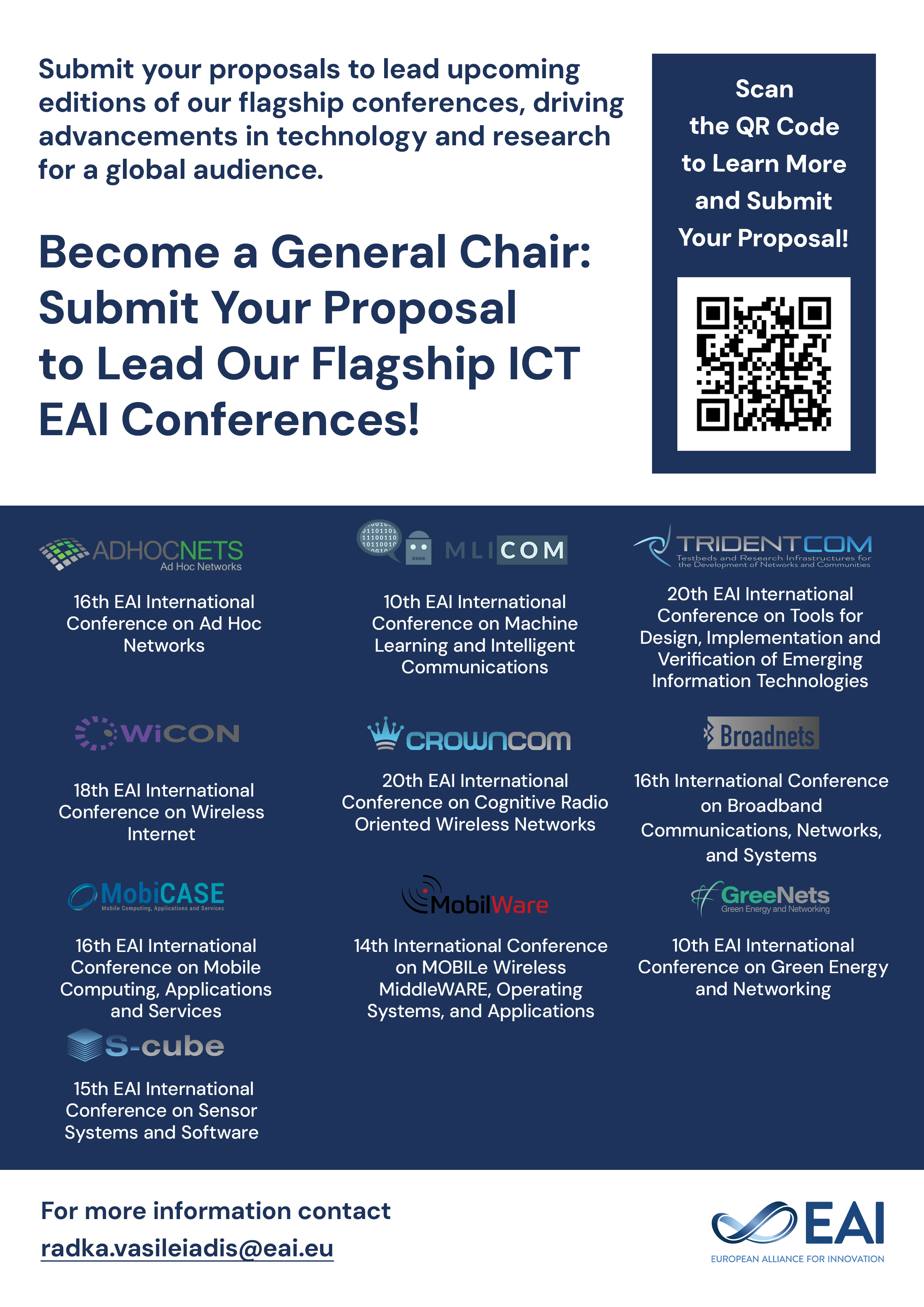
Research Article
A Pricing Incentive Mechanism for Mobile Crowd Sensing in Edge Computing
@INPROCEEDINGS{10.1007/978-3-030-28468-8_14, author={Xin Chen and Zhuo Li and Lianyong Qi and Ying Chen and Yuzhe Zhao and Shuang Chen}, title={A Pricing Incentive Mechanism for Mobile Crowd Sensing in Edge Computing}, proceedings={Mobile Computing, Applications, and Services. 10th EAI International Conference, MobiCASE 2019, Hangzhou, China, June 14--15, 2019, Proceedings}, proceedings_a={MOBICASE}, year={2019}, month={9}, keywords={Mobile crowd sensing Pricing Social welfare Incentive mechanism Convex optimization}, doi={10.1007/978-3-030-28468-8_14} }
- Xin Chen
Zhuo Li
Lianyong Qi
Ying Chen
Yuzhe Zhao
Shuang Chen
Year: 2019
A Pricing Incentive Mechanism for Mobile Crowd Sensing in Edge Computing
MOBICASE
Springer
DOI: 10.1007/978-3-030-28468-8_14
Abstract
Mobile crowd sensing (MCS) has been recognized as a promising method to acquire massive volume of data. Stimulating the enthusiasm of participants could be challenging at the same time. In this paper, we first propose a three-layer mobile crowd sensing architecture and introduce edge servers into it. The edge servers are used to process raw data and improve response time. Our goal is to maximize social welfare. Specifically, we model the social welfare maximization problem by Markov decision process and study a convex optimization pricing problem in the proposed three-layer architecture. The size of the tasks the edge servers assign is adjustable in this system. Then Lagrange multiplier method is leveraged to solve the problem. We derive the experimental data from real-world dataset and extensive simulations demonstrate the performance of our proposed method.