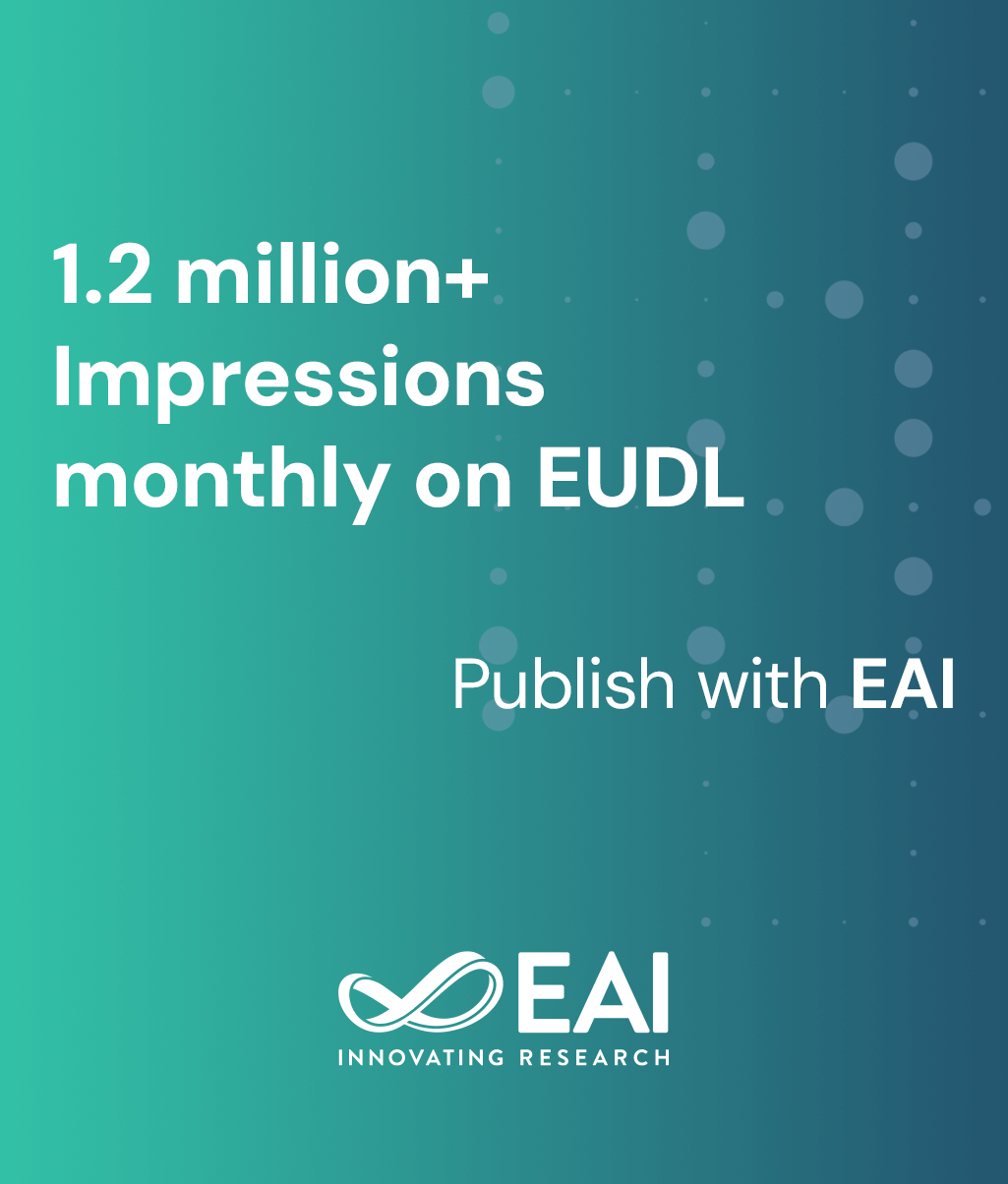
Research Article
Progress in Interpretability Research of Convolutional Neural Networks
@INPROCEEDINGS{10.1007/978-3-030-28468-8_12, author={Wei Zhang and Lizhi Cai and Mingang Chen and Naiqi Wang}, title={Progress in Interpretability Research of Convolutional Neural Networks}, proceedings={Mobile Computing, Applications, and Services. 10th EAI International Conference, MobiCASE 2019, Hangzhou, China, June 14--15, 2019, Proceedings}, proceedings_a={MOBICASE}, year={2019}, month={9}, keywords={Convolutional neural networks “black box” Interpretability}, doi={10.1007/978-3-030-28468-8_12} }
- Wei Zhang
Lizhi Cai
Mingang Chen
Naiqi Wang
Year: 2019
Progress in Interpretability Research of Convolutional Neural Networks
MOBICASE
Springer
DOI: 10.1007/978-3-030-28468-8_12
Abstract
Convolutional neural networks have made unprecedented breakthroughs in various tasks of computer vision. Due to its complex nonlinear model structure and the high latitude and complexity of data distribution, it has been criticized as an unexplained “black box”. Therefore, explaining the neural network model and uncovering the veil of the neural network have become the focus of attention. This paper starts with the term “interpretability”, summarizes the results of the interpretability of convolutional neural networks in the past three years (2016–2018), and analyses them with interpretable methods. Firstly, the concept of “interpretability” is introduced. Then the existing research achievements are classified and compared from four aspects, data characteristics and rule processing, model internal spatial analysis, interpretation and prediction, and model interpretation. Finally pointed out the possible research directions.