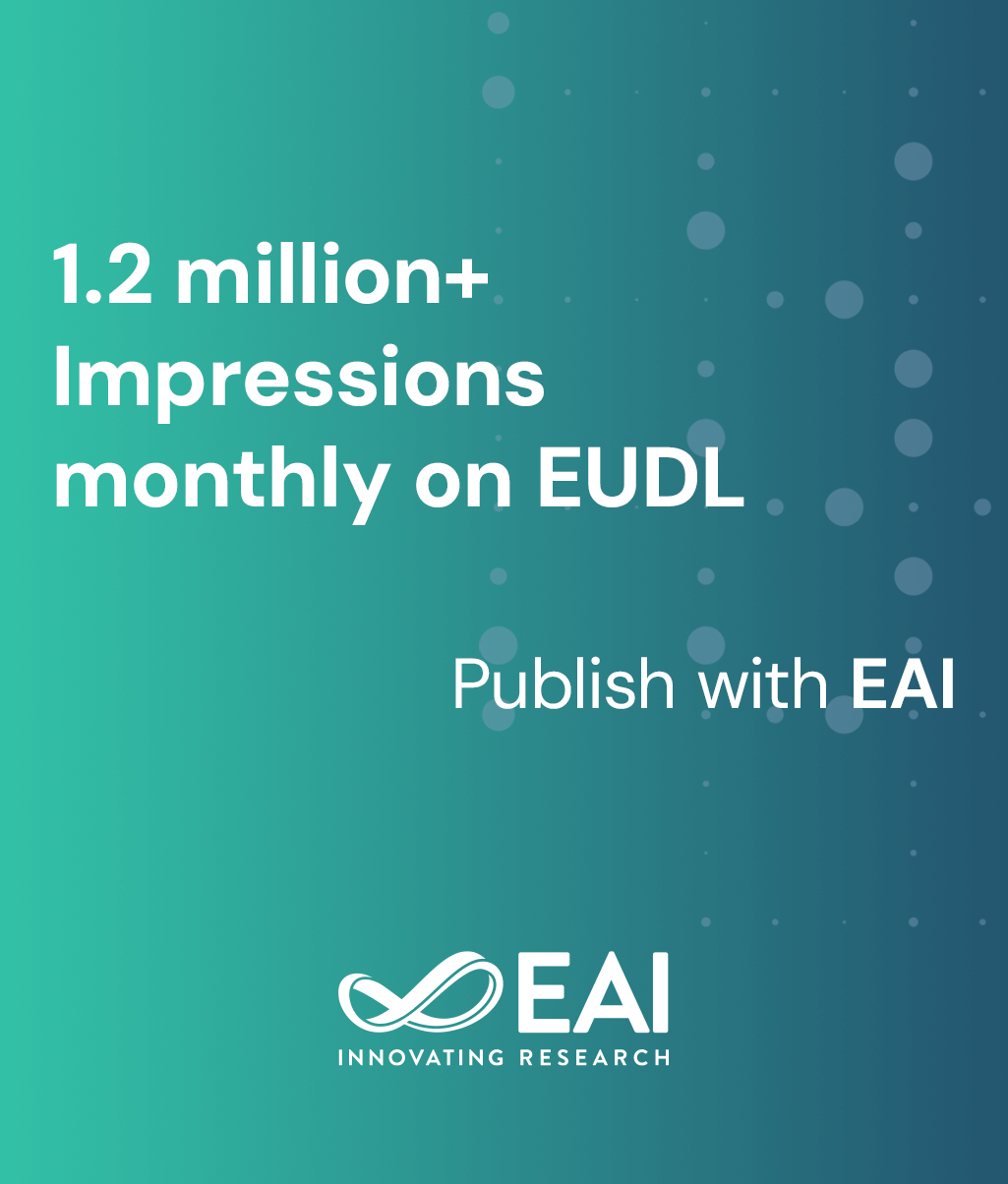
Research Article
A Location and Intention Oriented Recommendation Method for Accuracy Enhancement over Big Data
@INPROCEEDINGS{10.1007/978-3-030-28468-8_1, author={Wajid Rafique and Lianyong Qi and Zhili Zhou and Xuan Zhao and Wenda Tang and Wanchun Dou}, title={A Location and Intention Oriented Recommendation Method for Accuracy Enhancement over Big Data}, proceedings={Mobile Computing, Applications, and Services. 10th EAI International Conference, MobiCASE 2019, Hangzhou, China, June 14--15, 2019, Proceedings}, proceedings_a={MOBICASE}, year={2019}, month={9}, keywords={Intention-oriented recommendation Location-based clustering Spatial Performance improvement}, doi={10.1007/978-3-030-28468-8_1} }
- Wajid Rafique
Lianyong Qi
Zhili Zhou
Xuan Zhao
Wenda Tang
Wanchun Dou
Year: 2019
A Location and Intention Oriented Recommendation Method for Accuracy Enhancement over Big Data
MOBICASE
Springer
DOI: 10.1007/978-3-030-28468-8_1
Abstract
Big data recommendation systems provide recommendations based on user history and optimize this process using feedback information. Recent developments in location-based social networks reveal that spatial properties of users greatly affect their opinion. Traditional location-aware recommendation systems do not consider user intentions to produce personalized recommendations. This paper proposes LIOR, a Location and Intention Oriented Recommendation method that uses spatial properties of users and their intentions to produce personalized recommendations. LIOR hierarchically employs user location and rating information to generate location-aware predictions, it then integrates user intentions to produce highly accurate recommendations. Extensive experimental evaluation performed on a real-world location-aware Movielens dataset demonstrates that LIOR provides exceptional performance on producing recommendations, it is highly scalable, and efficiently reduces the sparsity problem.