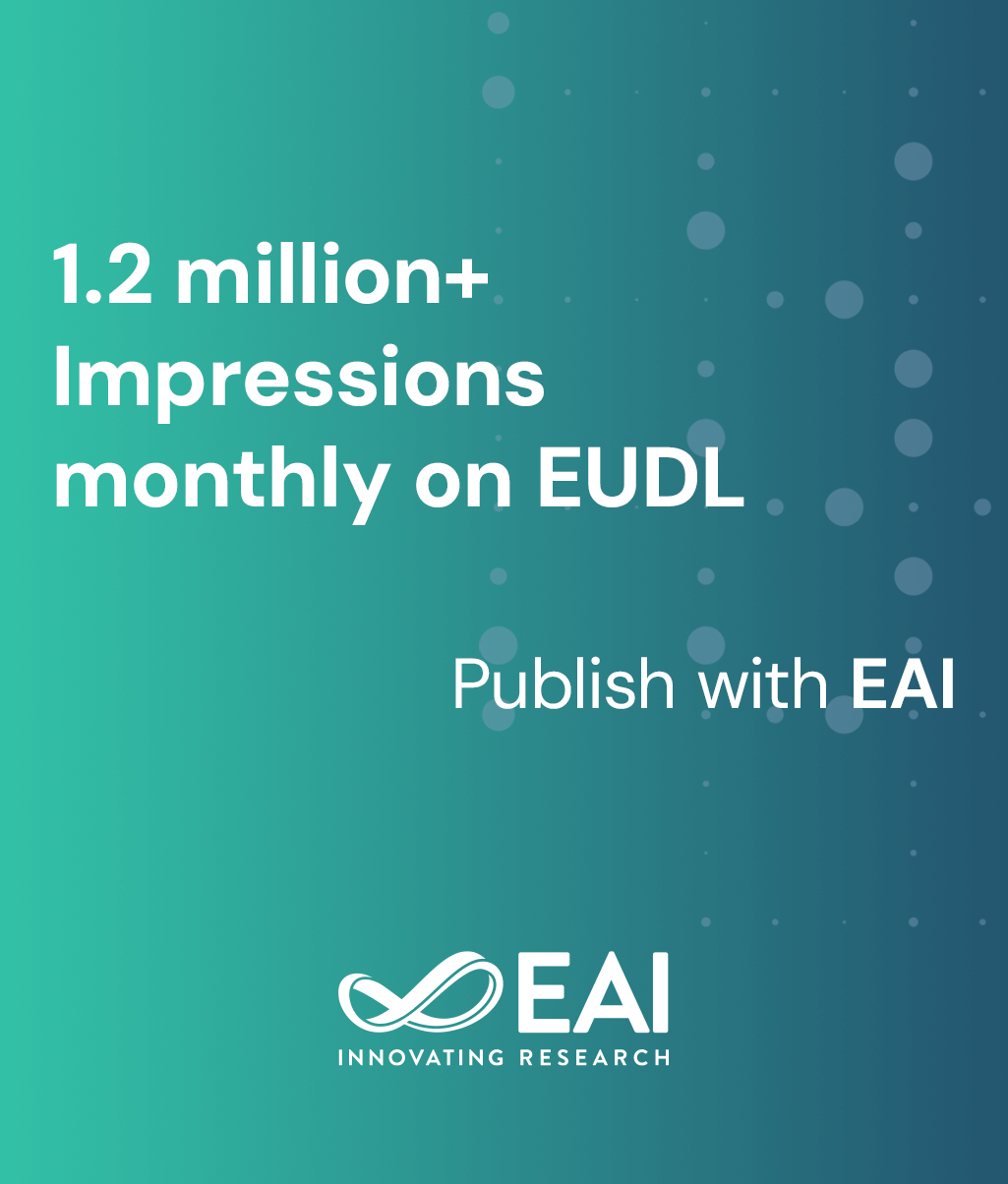
Research Article
Evolutionary Multi-objective Optimization for Evolving Soft Robots in Different Environments
@INPROCEEDINGS{10.1007/978-3-030-24202-2_9, author={Jun Ogawa}, title={Evolutionary Multi-objective Optimization for Evolving Soft Robots in Different Environments}, proceedings={Bio-inspired Information and Communication Technologies. 11th EAI International Conference, BICT 2019, Pittsburgh, PA, USA, March 13--14, 2019, Proceedings}, proceedings_a={BICT}, year={2019}, month={7}, keywords={Evolutionary robotics Soft robotics Neural network Pareto optimization Artificial life}, doi={10.1007/978-3-030-24202-2_9} }
- Jun Ogawa
Year: 2019
Evolutionary Multi-objective Optimization for Evolving Soft Robots in Different Environments
BICT
Springer
DOI: 10.1007/978-3-030-24202-2_9
Abstract
Evolutionary robotics is an approach for optimizing a robotic control system and structure based on the bio-inspired mechanism of adaptiogenesis. Conventional evolutionary robotics assigns a task and an evaluation to a virtual robot and acquires an optimal control system. In many cases, however, the robot is composed of a few rigid primitives and the morphology imitates that of real animals, insects, and artifacts. This paper proposes a novel approach to evolutionary robotics combining morphological evolution and soft robotics to optimize the control system of a soft robot. Our method calculates the relational dynamics among morphological changes and autonomous behavior for neuro-evolution (NE) with the development of a complex soft-bodied robot and the accomplishment of multiple tasks. We develop a soft-bodied robot composed of heterogeneous materials in two stages: a development stage and a locomotion stage, and we optimize these robotic structures by combining an artificial neural network (ANN) and age-fitness pareto optimization (AFP). These body structures of the robot are determined depending on three genetic rules and some voxels for evolving the ANN. In terms of our experimental results, our approach enabled us to develop some adaptive structural robots that simultaneously acquire behavior for crawling both on the ground and underwater. Subsequently, we discovered an unintentional morphology and behavior (e.g., walking, swimming, and crawling) of the soft robot through the evolutionary process. Some of the robots have high generalization ability with the ability to crawl to any target in any direction by only learning a one-directional crawling task.