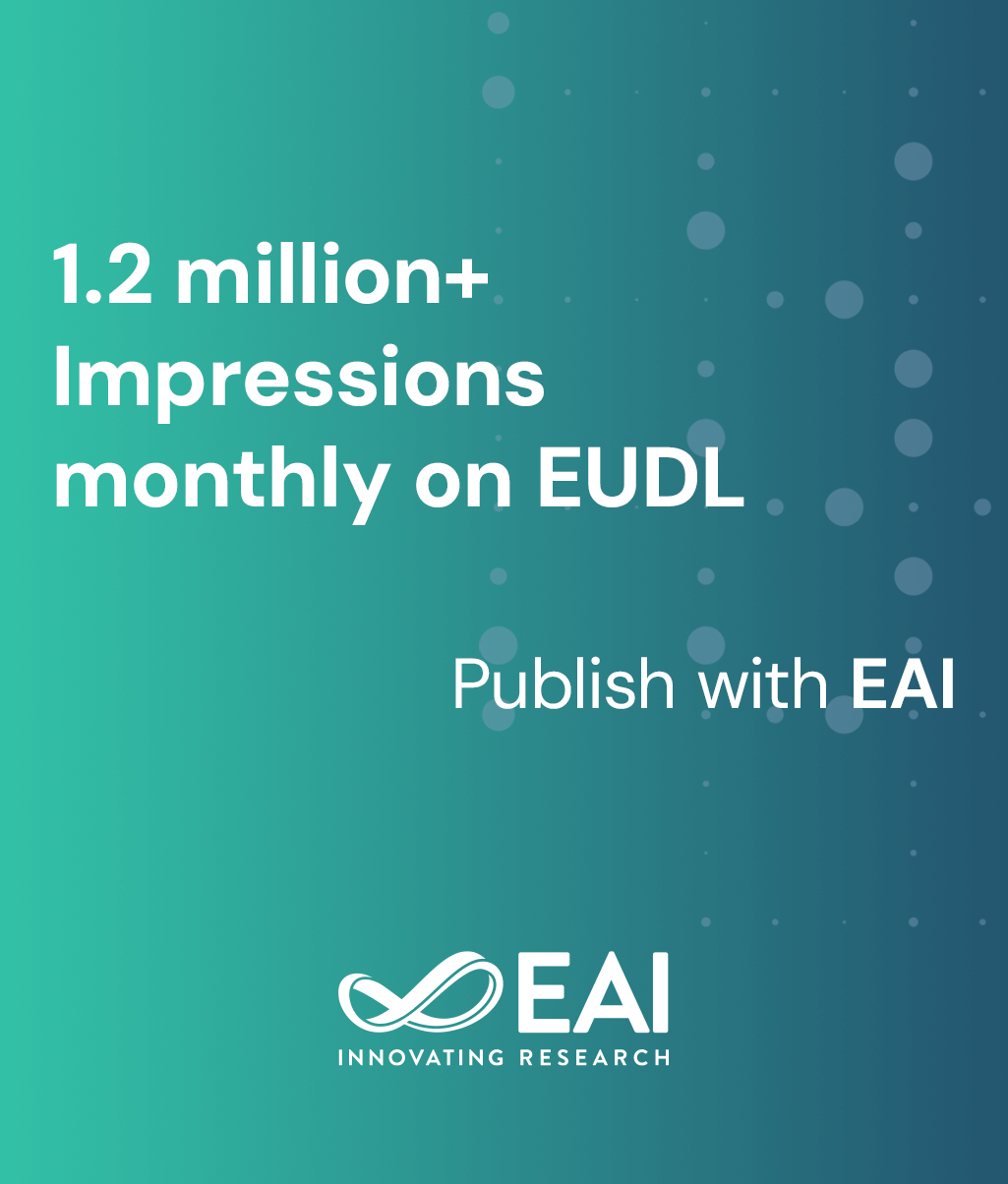
Research Article
Ground Sky Imager Based Short Term Cloud Coverage Prediction
@INPROCEEDINGS{10.1007/978-3-030-23976-3_33, author={Stefan Hensel and Marin Marinov and Raphael Schwarz and Ivan Topalov}, title={Ground Sky Imager Based Short Term Cloud Coverage Prediction}, proceedings={Future Access Enablers for Ubiquitous and Intelligent Infrastructures. 4th EAI International Conference, FABULOUS 2019, Sofia, Bulgaria, March 28-29, 2019, Proceedings}, proceedings_a={FABULOUS}, year={2019}, month={9}, keywords={Cloud coverage High dynamic range images Prediction algorithms Short term irradiance prediction}, doi={10.1007/978-3-030-23976-3_33} }
- Stefan Hensel
Marin Marinov
Raphael Schwarz
Ivan Topalov
Year: 2019
Ground Sky Imager Based Short Term Cloud Coverage Prediction
FABULOUS
Springer
DOI: 10.1007/978-3-030-23976-3_33
Abstract
The paper describes a systematic approach for a precise short-time cloud coverage prediction based on an optical system. We present a distinct pre-processing stage that uses a model based clear sky simulation to enhance the cloud segmentation in the images. The images are based on a sky imager system with fish-eye lens optic to cover a maximum area. After a calibration step, the image is rectified to enable linear prediction of cloud movement. In a subsequent step, the clear sky model is estimated on actual high dynamic range images and combined with a threshold based approach to segment clouds from sky. In the final stage, a multi hypothesis linear tracking framework estimates cloud movement, velocity and possible coverage of a given photovoltaic power station. We employ a Kalman filter framework that efficiently operates on the rectified images. The evaluation on real world data suggests high coverage prediction accuracy above 75%.