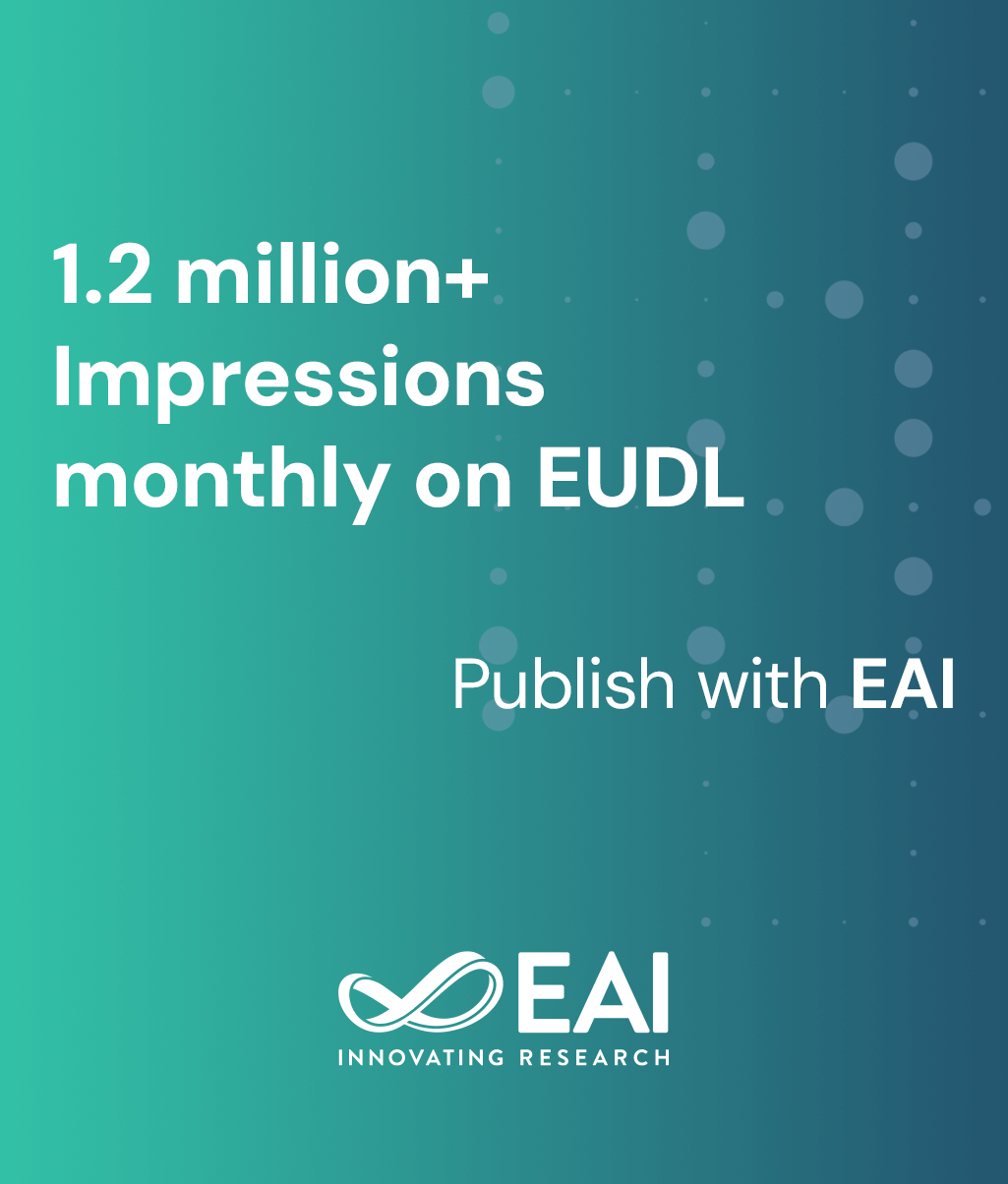
Research Article
Hybrid Noise-Resilient Deep Learning Architecture for Modulation Classification in Cognitive Radio Networks
@INPROCEEDINGS{10.1007/978-3-030-23976-3_20, author={Antoni Ivanov and Krasimir Tonchev and Vladimir Poulkov and Hussein Al-Shatri and Anja Klein}, title={Hybrid Noise-Resilient Deep Learning Architecture for Modulation Classification in Cognitive Radio Networks}, proceedings={Future Access Enablers for Ubiquitous and Intelligent Infrastructures. 4th EAI International Conference, FABULOUS 2019, Sofia, Bulgaria, March 28-29, 2019, Proceedings}, proceedings_a={FABULOUS}, year={2019}, month={9}, keywords={Cognitive Radio Deep Learning Modulation classification Spectrum sensing}, doi={10.1007/978-3-030-23976-3_20} }
- Antoni Ivanov
Krasimir Tonchev
Vladimir Poulkov
Hussein Al-Shatri
Anja Klein
Year: 2019
Hybrid Noise-Resilient Deep Learning Architecture for Modulation Classification in Cognitive Radio Networks
FABULOUS
Springer
DOI: 10.1007/978-3-030-23976-3_20
Abstract
The increasing maturity of the concepts which would allow for the operation of a practical Cognitive Radio (CR) Network require functionalities derived through different methodologies from other fields. One such approach is Deep Learning (DL) which can be applied to diverse problems in CR to enhance its effectiveness by increasing the utilization of the unused radio spectrum. Using DL, the CR device can identify whether the signal comes from the Primary User (PU) transmitter or from an interferer. The method proposed in this paper is a hybrid DL architecture which aims at achieving high recognition rate at low signal-to-noise ratio (SNR) and various channel impairments including fading because such are the relevant conditions of operation of the CR. It consists of an autoencoder and a neural network structure due to the good denoising qualities of the former and the recognition accuracy of the latter. The autoencoder aims to restore the original signal from the corrupted samples which would increase the accuracy of the classifier. Afterwards its output is fed into the NN which learns the characteristics of each modulation type and classifies the restored signal correctly with certain probability. To determine the optimal classification DL model, several types of NN structures are examined and compared for input comprised of the IQ samples of the reconstructed signal. The performance of the proposed DL architecture in comparison to similar models for the relevant parameters in different channel impairments scenarios is also analyzed.