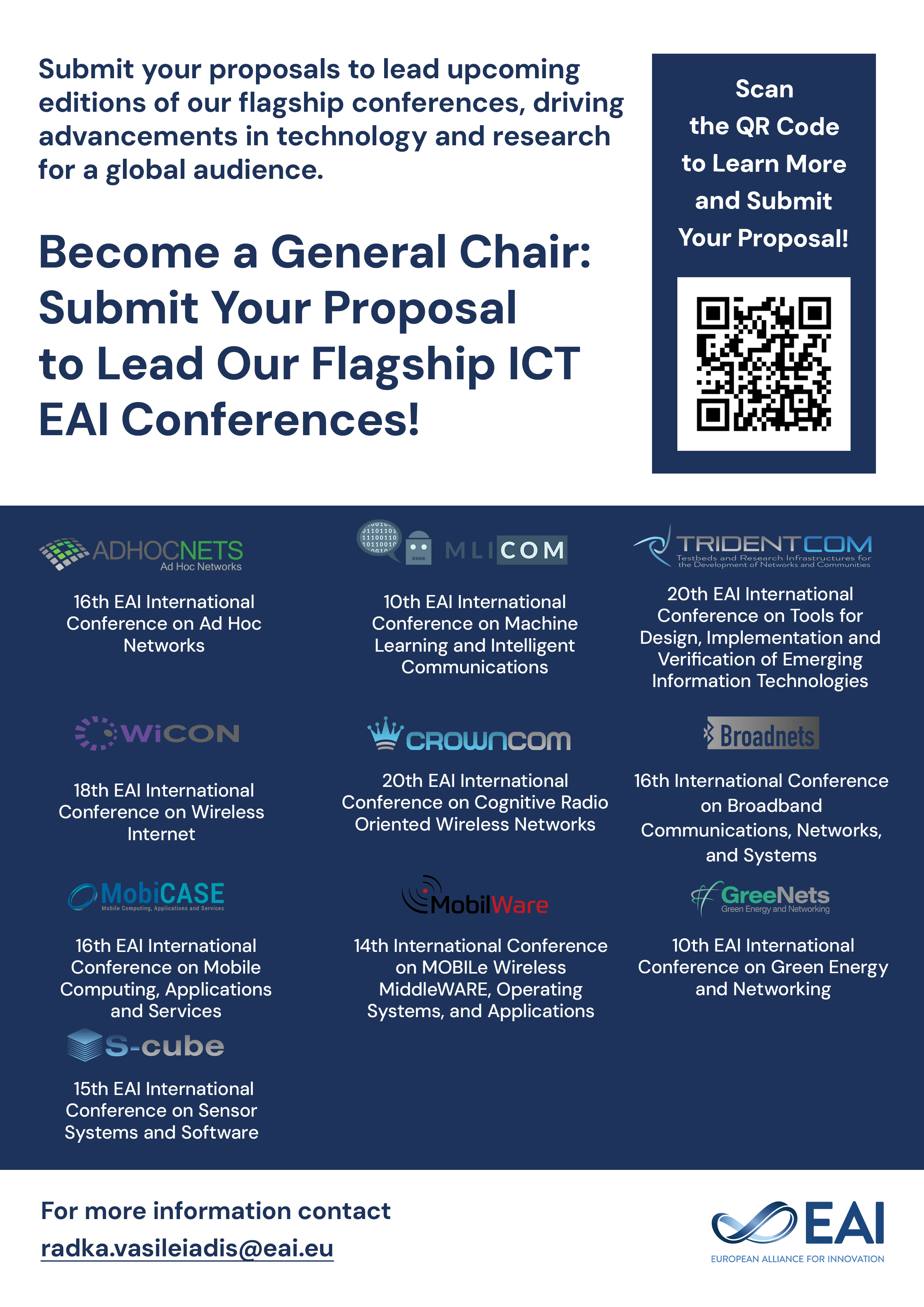
Research Article
A Framework for Classification of Data Stream Application in Vehicular Network Computing
@INPROCEEDINGS{10.1007/978-3-030-21730-3_6, author={Ling Yu and Yang Gao and Yu Zhang and Li Guo}, title={A Framework for Classification of Data Stream Application in Vehicular Network Computing}, proceedings={Green Energy and Networking. 6th EAI International Conference, GreeNets 2019, Dalian, China, May 4, 2019, Proceedings}, proceedings_a={GREENETS}, year={2019}, month={6}, keywords={Data stream classification ESVM Incremental learning MapReduce Vehicular network}, doi={10.1007/978-3-030-21730-3_6} }
- Ling Yu
Yang Gao
Yu Zhang
Li Guo
Year: 2019
A Framework for Classification of Data Stream Application in Vehicular Network Computing
GREENETS
Springer
DOI: 10.1007/978-3-030-21730-3_6
Abstract
Due to the fast developing of intelligent vehicles and the dynamic sensor network, a huge amount of data streams are generated continuously. How to manage these data stream will play a very important role in the development of next-generation intelligent vehicular networks. Data stream classification problem poses many new challenges to the data mining area. One of the most basic challenges is how to make a tradeoff between data stream generating and classifying process. In this paper, we address this problem using a new framework and a new classification method, namely Extreme Support Vector Machine (ESVM), for large-scale data stream classification for specialty vehicle network. Moreover, a new forgetting strategy is designed for the proposed TFS-PESVM model, which employs the residual weight matrix to optimal residual value and improves the effectiveness of new data samples. The experimental results show that the proposed data stream classification model can improve the speed of classification process and less affected by noise interference.