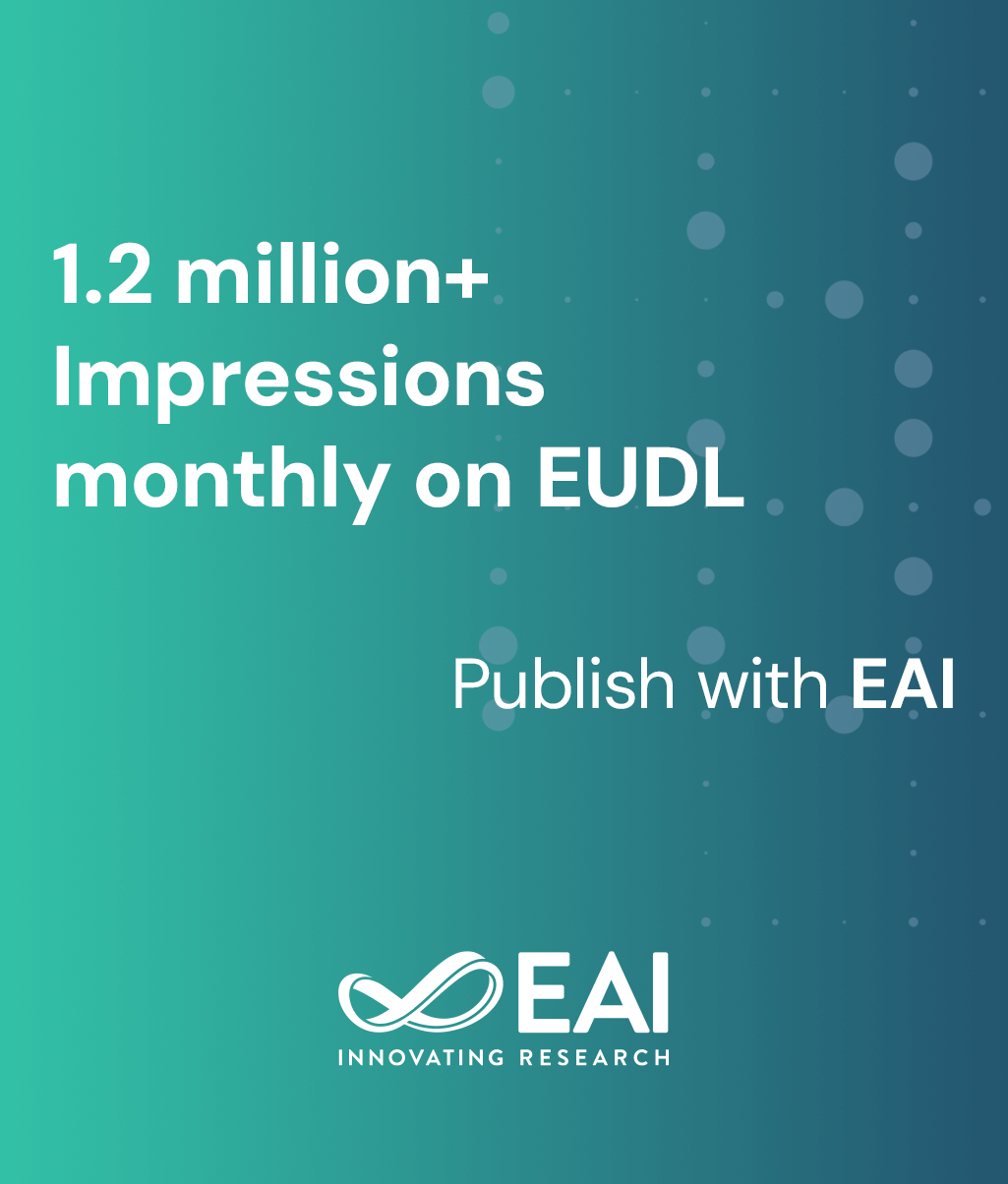
Research Article
The Physical Layer Identification of Communication Devices Based on RF-DNA
@INPROCEEDINGS{10.1007/978-3-030-19086-6_50, author={Ying Li and Xiang Chen and Jie Chang and Yun Lin}, title={The Physical Layer Identification of Communication Devices Based on RF-DNA}, proceedings={Advanced Hybrid Information Processing. Second EAI International Conference, ADHIP 2018, Yiyang, China, October 5-6, 2018, Proceedings}, proceedings_a={ADHIP}, year={2019}, month={5}, keywords={RF fingerprinting Physical layer identification Feature extraction Device classification}, doi={10.1007/978-3-030-19086-6_50} }
- Ying Li
Xiang Chen
Jie Chang
Yun Lin
Year: 2019
The Physical Layer Identification of Communication Devices Based on RF-DNA
ADHIP
Springer
DOI: 10.1007/978-3-030-19086-6_50
Abstract
Traditional methods of improving wireless network security are through software-level device identification, such as IP or MAC addresses. However, these identifiers can be easily changed by software, making wireless network communication a high risk. In response to these risks, radio frequency fingerprinting technology has been proposed. Since the radio frequency fingerprint is an essential feature of the physical layer of the wireless communication device and is difficult to be tampered with, it is widely used to improve the security of the wireless network. Based on the physical layer characteristics of the communication system, this paper has established a relatively complete RF fingerprint identification system to realize the identification and classification of the devices. Two signal starting point detection methods and two RF fingerprint feature extraction methods are studied in this paper. The detailed results are obtained by combining the dimensionality reduction and classification methods. Finally, an optimal identification scheme was found to achieve a classification accuracy of more than 90% when the signal-to-noise ratio is greater than 15 dB.