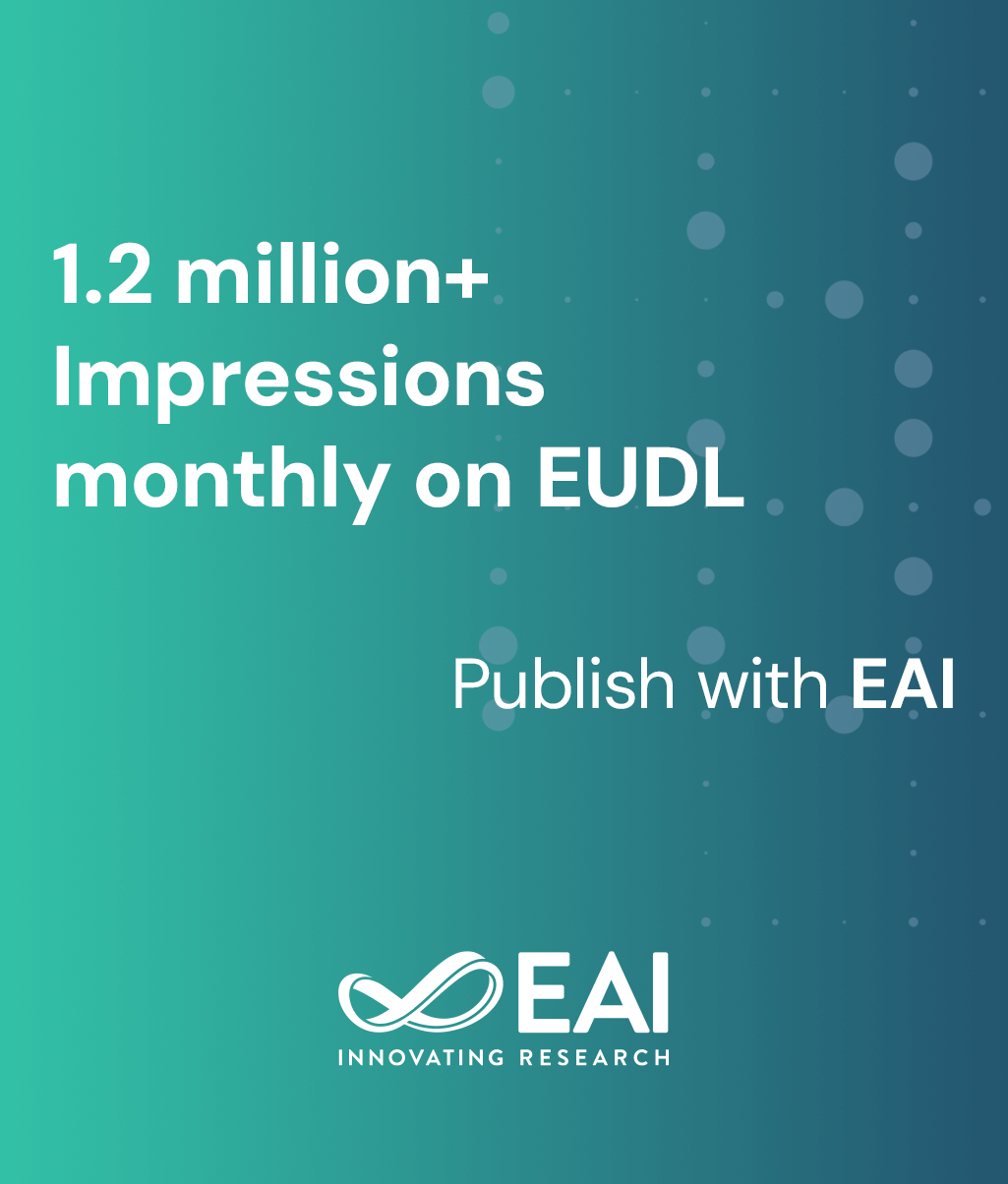
Research Article
Electromagnetic Spectrum Threat Prediction via Deep Learning
@INPROCEEDINGS{10.1007/978-3-030-19086-6_48, author={Chunyan Wei and Lin Qi and Ruowu Wu and Yun Lin}, title={Electromagnetic Spectrum Threat Prediction via Deep Learning}, proceedings={Advanced Hybrid Information Processing. Second EAI International Conference, ADHIP 2018, Yiyang, China, October 5-6, 2018, Proceedings}, proceedings_a={ADHIP}, year={2019}, month={5}, keywords={Electromagnetic spectrum threat Prediction Generative Adversarial Networks}, doi={10.1007/978-3-030-19086-6_48} }
- Chunyan Wei
Lin Qi
Ruowu Wu
Yun Lin
Year: 2019
Electromagnetic Spectrum Threat Prediction via Deep Learning
ADHIP
Springer
DOI: 10.1007/978-3-030-19086-6_48
Abstract
Nowadays, in the complex electromagnetic environment, the detection of foreign satellite, the electronic interferences and the sensing data tampering in the process of consistent spectrum situation fusion and the electronic countermeasures reconnaissance and enforcement implemented by the enemy electronic attacks all pose serious threats to the communication performance of our electronic devices and communication systems. Therefore, how to detect these electromagnetic spectrum threats effectively is very important. The generative adversarial networks was applied in this paper, which is a method in deep learning, and an unsupervised solution for the above-mentioned electromagnetic spectrum threat signal prediction problem was provided, which has achieved good results. To carry out the detection experiments, three common electromagnetic spectrum threat scenarios were simulated. The prediction performance of the model is evaluated based on the prediction accuracy of the model. The experimental results have shown that the generative adversarial networks model used in this paper has a good predictive effect on the electromagnetic spectrum threat signals of a certain intensity.