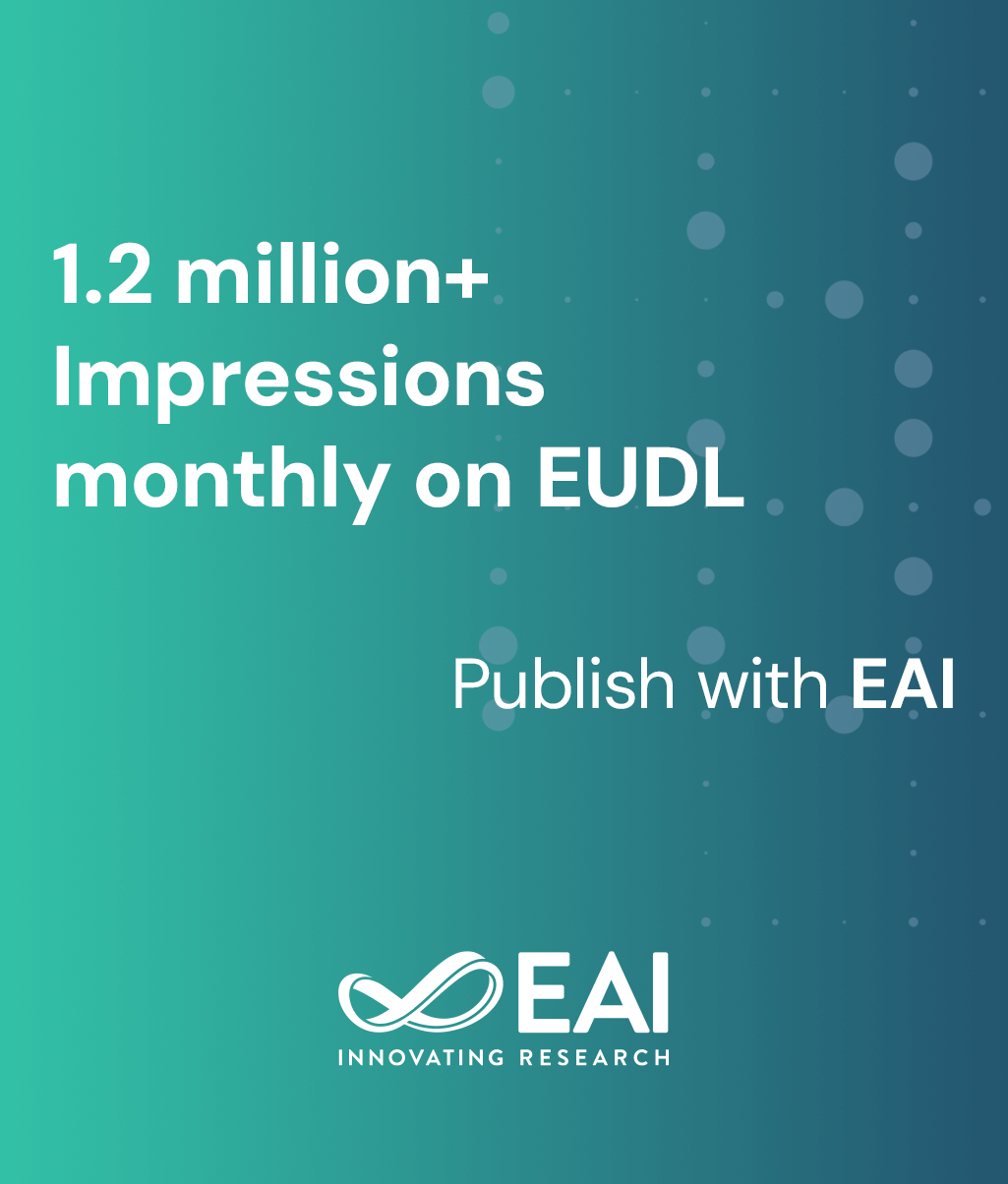
Research Article
Precipitation Prediction Based on KPCA Support Vector Machine Optimization
@INPROCEEDINGS{10.1007/978-3-030-19086-6_42, author={Fangqiong Luo and Guodong Wang and Yu Zhang}, title={Precipitation Prediction Based on KPCA Support Vector Machine Optimization}, proceedings={Advanced Hybrid Information Processing. Second EAI International Conference, ADHIP 2018, Yiyang, China, October 5-6, 2018, Proceedings}, proceedings_a={ADHIP}, year={2019}, month={5}, keywords={Kernel principle component analysis (KPCA) Particle swarm Support vector machine (SVM) Precipitationion}, doi={10.1007/978-3-030-19086-6_42} }
- Fangqiong Luo
Guodong Wang
Yu Zhang
Year: 2019
Precipitation Prediction Based on KPCA Support Vector Machine Optimization
ADHIP
Springer
DOI: 10.1007/978-3-030-19086-6_42
Abstract
In this paper, kernel principle component analysis (KPCA) is employed to extract the features of multiple precipitation factors. The extracted principle components are considered as the characteristic vector of support vector machine (SVM) to build the SVM precipitation forecast model. We calculate the SVM parameters using particle swarm optimization (PSO) algorithm, and build the cooperative model of KPCA and the SVM with PSO to predict the precipitation in Guangxi province. The simulation results show that the prediction outcome, resulting from the combination of KPCA and the SVM with PSO, is consistent with the actual precipitation. Comparisons with other models also demonstrate that our model has advantages in fitting and generalizing in comparison other models.