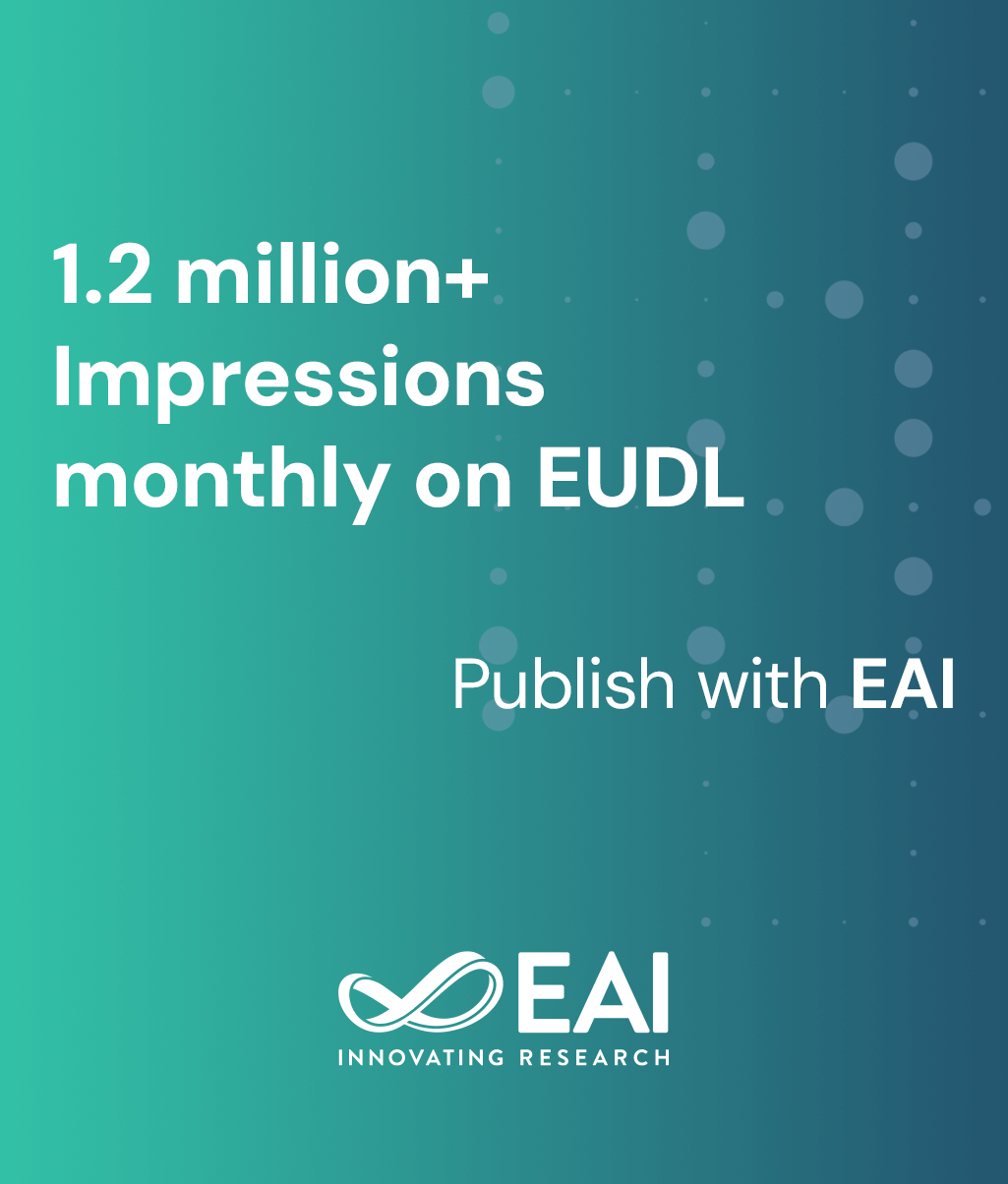
Research Article
Parallel Implementation and Optimization of a Hybrid Data Assimilation Algorithm
@INPROCEEDINGS{10.1007/978-3-030-19086-6_34, author={Jingmeifang Li and Weifei Wu}, title={Parallel Implementation and Optimization of a Hybrid Data Assimilation Algorithm}, proceedings={Advanced Hybrid Information Processing. Second EAI International Conference, ADHIP 2018, Yiyang, China, October 5-6, 2018, Proceedings}, proceedings_a={ADHIP}, year={2019}, month={5}, keywords={Parallel MPI Data assimilation Optimization}, doi={10.1007/978-3-030-19086-6_34} }
- Jingmeifang Li
Weifei Wu
Year: 2019
Parallel Implementation and Optimization of a Hybrid Data Assimilation Algorithm
ADHIP
Springer
DOI: 10.1007/978-3-030-19086-6_34
Abstract
Data assimilation plays a very important role in numerical weather forecasting, and data assimilation algorithms are the core of data assimilation. The objective function of common data assimilation algorithms currently has a large amount of calculation, which takes more time to solve, thereby causing the time cost of the assimilation process to affect the timeliness of the numerical weather forecast. Aiming at an excellent hybrid data assimilation algorithm-dimension reduction projection four-dimensional variational algorithm that has appeared in recent years, the paper uses the MPI parallel programming model for parallel implementation and optimization of the algorithm, and effectively solves the problem of large computational complexity of the objective function. This effectively not only reduces the solution time of the algorithm’s objective function, but also ensures the effect of assimilation. Experiments show that the speedup of the paralleled and optimized algorithm is about 17, 26, and 32 on 32, 64, and 128 processors, and the average speedup is about 26.