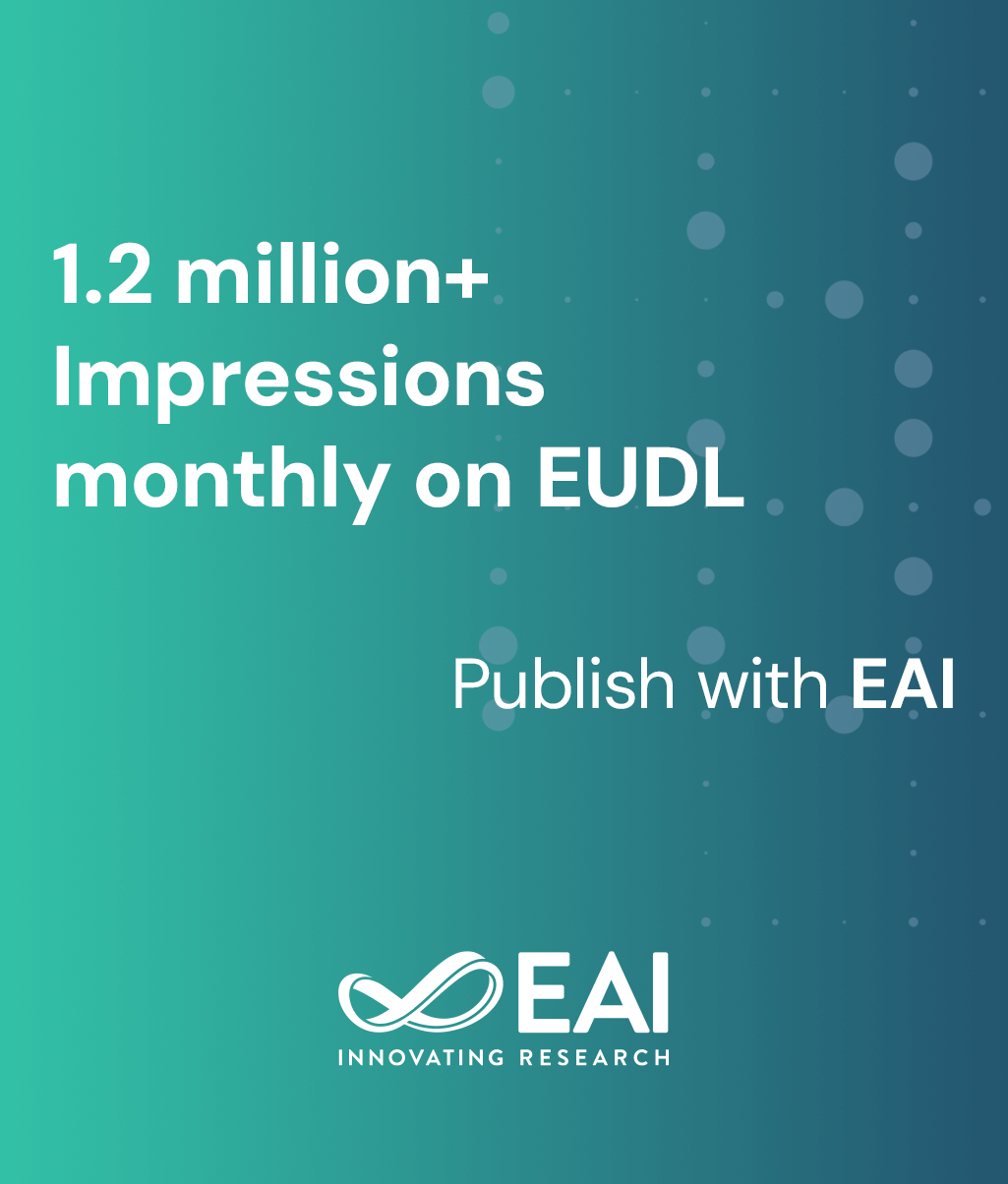
Research Article
A Large-Scale Image Retrieval Method Based on Image Elimination Technology and Supervised Kernel Hash
@INPROCEEDINGS{10.1007/978-3-030-19086-6_26, author={Zhiming Yin and Jianguo Sun and Xingjian Zhang and Liu Sun and Hanqi Yin}, title={A Large-Scale Image Retrieval Method Based on Image Elimination Technology and Supervised Kernel Hash}, proceedings={Advanced Hybrid Information Processing. Second EAI International Conference, ADHIP 2018, Yiyang, China, October 5-6, 2018, Proceedings}, proceedings_a={ADHIP}, year={2019}, month={5}, keywords={Image retrieval Image redundancy Fast matching Supervised kernel hashing Fuzzy logic inference}, doi={10.1007/978-3-030-19086-6_26} }
- Zhiming Yin
Jianguo Sun
Xingjian Zhang
Liu Sun
Hanqi Yin
Year: 2019
A Large-Scale Image Retrieval Method Based on Image Elimination Technology and Supervised Kernel Hash
ADHIP
Springer
DOI: 10.1007/978-3-030-19086-6_26
Abstract
The Internet develops rapidly in the era of big data, which can be shown by the widespread uses of image processing software as well as digital images skills. However, there are a large number of redundant images in the network, which not only occupy the network storage but also slow down image search speed. At the same time, the image hash algorithm has received extensive attention due to its advantages of improving the image retrieval efficiency while reducing storage space. Therefore, this paper aims to propose a large-scale image retrieval method based on image redundancy and hash algorithm for large-scale image retrieval system with a large number of redundant images. I look upon the method into two phases: The first phase is eliminating the redundancy of repetitive images. As usual, image features need to be extracted from search results. Next, I use the K-way, Min-Max algorithm to cluster and sort the returned images and filter out the image classes in the end to improve the speed and accuracy of the image retrieval. Fuzzy logic reasoning comes to the last part. It can help to select the centroid image so as to achieve redundancy. The second phase is image matching. In this stage, the supervised kernel hashing is used to supervise the deep features of high-dimensional images and the high-dimensional features are mapped into low-dimensional Hamming space to generate compact hash codes. Finally, accomplish the efficient retrieval of large-scale image data in low-dimensional Hamming of the space. After texting three common dataset, the preliminary results show that the computational time can be reduced by the search image redundancy technology when filter out the invalid images. This greatly improves the efficiency of large-scale image retrieval and its image retrieval performance is better than the current mainstream method.