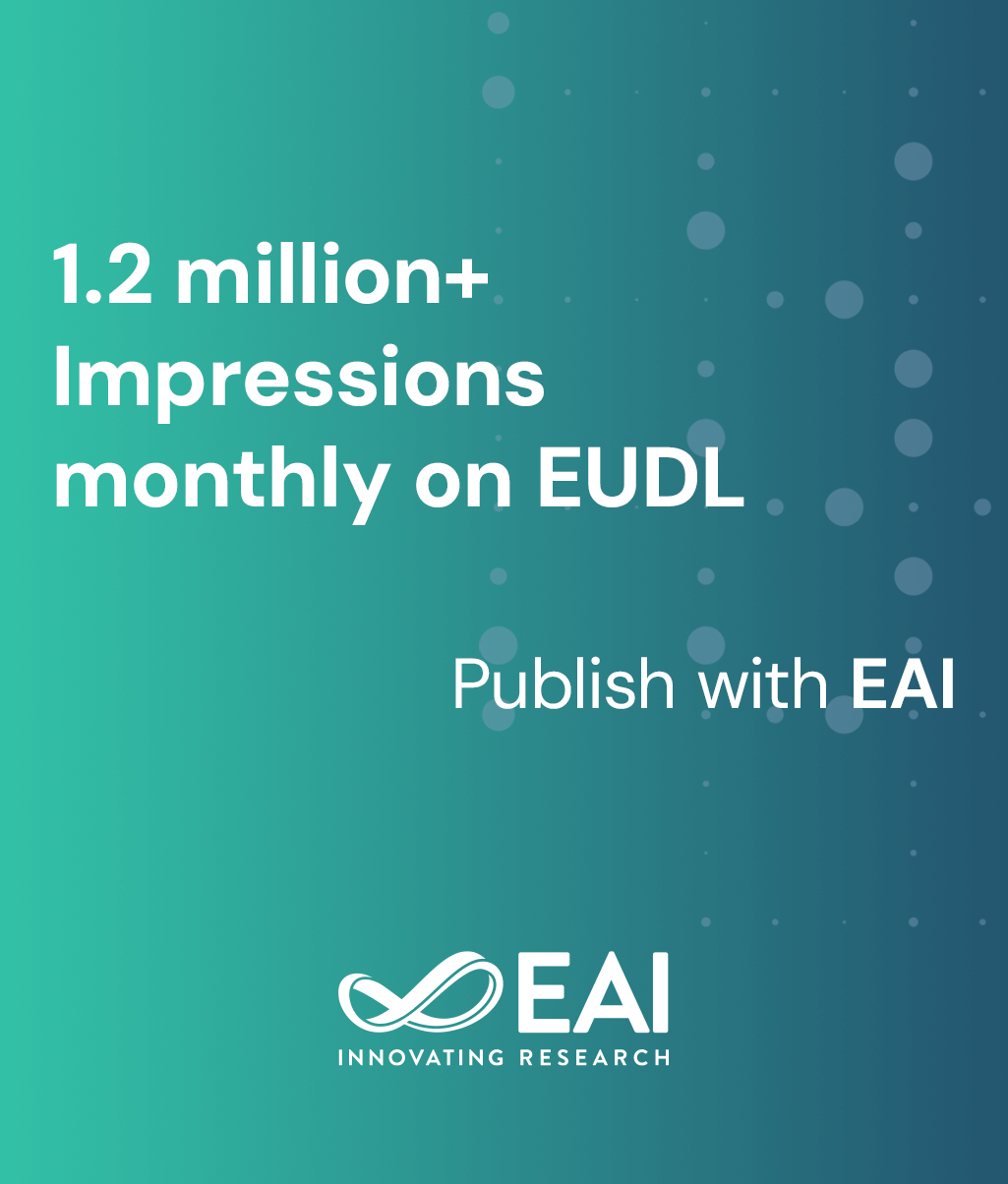
Research Article
-ADMM Algorithm for Sparse Image Recovery Under Impulsive Noise
@INPROCEEDINGS{10.1007/978-3-030-19086-6_1, author={Dongbin Hao and Chunjie Zhang and Yingjun Hao}, title={-ADMM Algorithm for Sparse Image Recovery Under Impulsive Noise}, proceedings={Advanced Hybrid Information Processing. Second EAI International Conference, ADHIP 2018, Yiyang, China, October 5-6, 2018, Proceedings}, proceedings_a={ADHIP}, year={2019}, month={5}, keywords={Alternative direction method of multipliers Augmented Lagrangian methods Compressive sensing Impulsive noise Inpainting images}, doi={10.1007/978-3-030-19086-6_1} }
- Dongbin Hao
Chunjie Zhang
Yingjun Hao
Year: 2019
-ADMM Algorithm for Sparse Image Recovery Under Impulsive Noise
ADHIP
Springer
DOI: 10.1007/978-3-030-19086-6_1
Abstract
The existing compressive sensing recovery algorithm has the problems of poor robustness, low peak signal-to-noise ratio (PSNR) and low applicability in images inpainting polluted by impulsive noise. In this paper, we proposed a robust algorithm for image recovery in the background of impulsive noise, called -ADMM algorithm. The proposed algorithm uses -norm substitute -norm residual term of cost function model to gain more image inpainting capability corrupted by impulsive noise and uses generalized non-convex penalty terms to ensure sparsity. The residual term of -norm is less sensitive to outliers in the observations than -norm. And using the non-convex penalty function can solve the offset problem of the -norm (not differential at zero point), so more accurate recovery can be obtained. The augmented Lagrange method is used to transform the constrained objective function model into an unconstrained model. Meanwhile, the alternating direction method can effectively improve the efficiently of -ADMM algorithm. Through numerical simulation results show that the proposed algorithm has better image inpainting performance in impulse noise environment by comparing with some state-of-the-art robust algorithms. Meanwhile, the proposed algorithm has flexible scalability for large-scale problem, which has better advantages for image progressing.