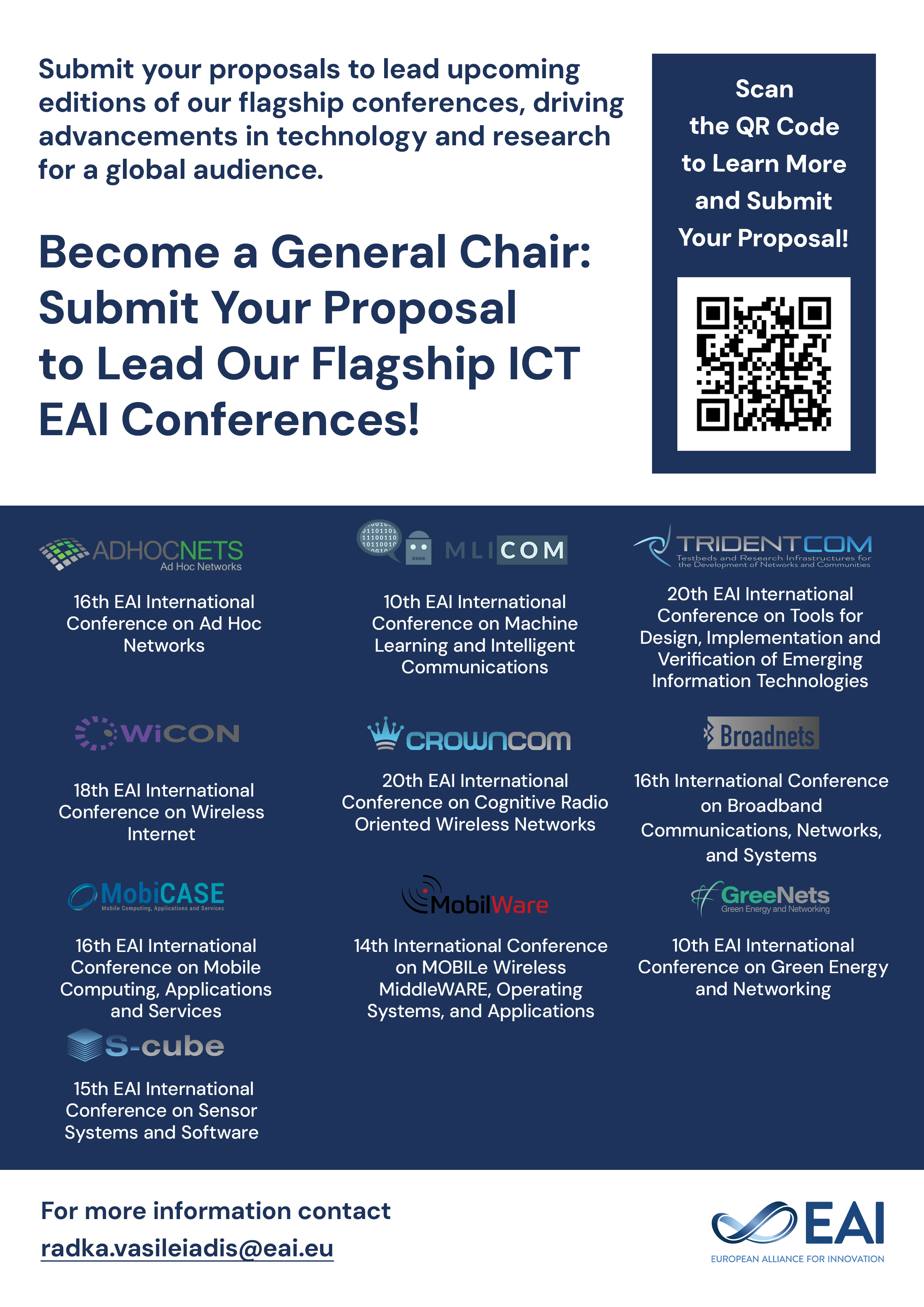
Research Article
Deep Learning Based Antenna Muting and Beamforming Optimization in Distributed Massive MIMO Systems
@INPROCEEDINGS{10.1007/978-3-030-17513-9_2, author={Yu Chen and Kai Zhao and Jing-ya Zhao and Qing-hua Zhu and Yong Liu}, title={Deep Learning Based Antenna Muting and Beamforming Optimization in Distributed Massive MIMO Systems}, proceedings={5G for Future Wireless Networks. Second EAI International Conference, 5GWN 2019, Changsha, China, February 23-24, 2019, Proceedings}, proceedings_a={5GWN}, year={2019}, month={4}, keywords={Deep learning Distributed massive MIMO Deep Neural Network Antenna muting Beamforming}, doi={10.1007/978-3-030-17513-9_2} }
- Yu Chen
Kai Zhao
Jing-ya Zhao
Qing-hua Zhu
Yong Liu
Year: 2019
Deep Learning Based Antenna Muting and Beamforming Optimization in Distributed Massive MIMO Systems
5GWN
Springer
DOI: 10.1007/978-3-030-17513-9_2
Abstract
Inspired by the success of Deep Learning (DL) in solving complex control problems, a new DL-based approximation framework to solve the problems of antenna muting and beamforming optimization in distributed massive MIMO was proposed. The main purpose is to obtain a non-linear mapping from the raw observations of networks to the optimal antenna muting and beamforming pattern, using Deep Neural Network (DNN). Firstly, the antenna muting and beamforming optimization problem is modeled as a non-combination optimization problem, which is NP-hard. Then a DNN based framework is proposed to obtain the optimal solution to this complex optimization problem with low-complexity. Finally, the performance of the DNN-based framework is evaluated in detail. Simulation results show that the proposed DNN framework can achieve a fairly accurate approximation. Moreover, compared with the traditional algorithm, DNN can be reduced the computation time by several orders of magnitude.