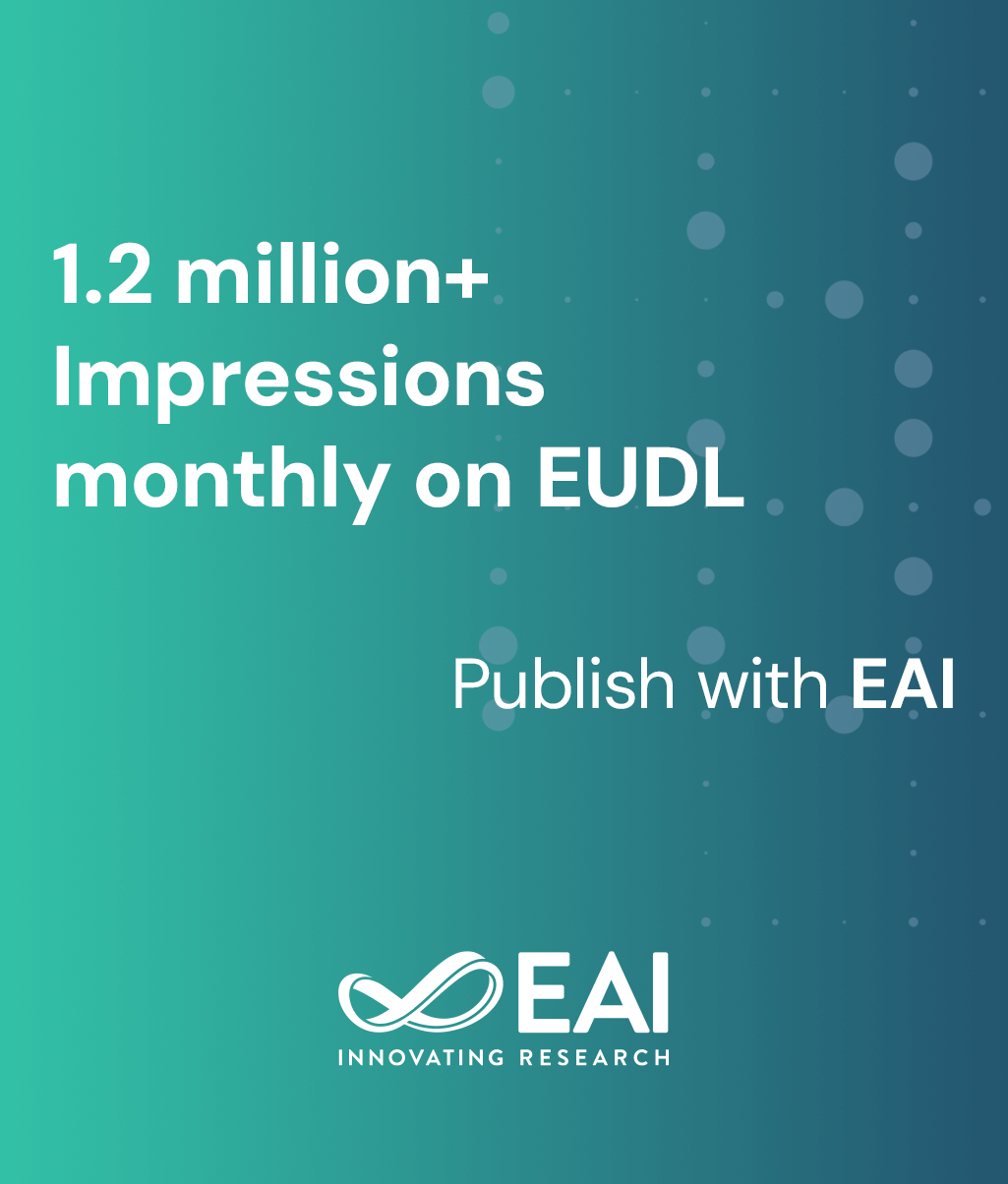
Research Article
Improved Flow Awareness by Intelligent Collaborative Sampling in Software Defined Networks
@INPROCEEDINGS{10.1007/978-3-030-17513-9_13, author={Jun Deng and He Cai and Xiaofei Wang}, title={Improved Flow Awareness by Intelligent Collaborative Sampling in Software Defined Networks}, proceedings={5G for Future Wireless Networks. Second EAI International Conference, 5GWN 2019, Changsha, China, February 23-24, 2019, Proceedings}, proceedings_a={5GWN}, year={2019}, month={4}, keywords={Edge Computing Deep Q-Learning SDN Flow-awareness Resource allocation}, doi={10.1007/978-3-030-17513-9_13} }
- Jun Deng
He Cai
Xiaofei Wang
Year: 2019
Improved Flow Awareness by Intelligent Collaborative Sampling in Software Defined Networks
5GWN
Springer
DOI: 10.1007/978-3-030-17513-9_13
Abstract
To improve the specific quality of service, internal network management and security analysis in the future mobile network, accurate flow-awareness in the global network through packet sampling has been a viable solution. However, the current traffic measurement method with the five tuples cannot recognize the deep information of flows, and the Deep Packet Inspection (DPI) deployed at the gateways or access points is lack of traffic going through the internal nodes (e.g., base station, edge server). In this paper, by means of Deep Q-Network (DQN) and Software-Defined Networking (SDN) technique, we propose a flow-level sampling framework for edge devices in the Mobile Edge Computing (MEC) system. In the framework, an original learning-based sampling strategy considering the iterative influences of nodes is used for maximizing the long-term sampling accuracy of both mice and elephant flows. We present an approach to effectively collect traffic packets generated from base stations and edge servers in two steps: (1) adaptive node selection, and (2) dynamic sampling duration allocation by Deep Q-Learning. The results show that the approach can improve the sampling accuracy, especially for mice flows.