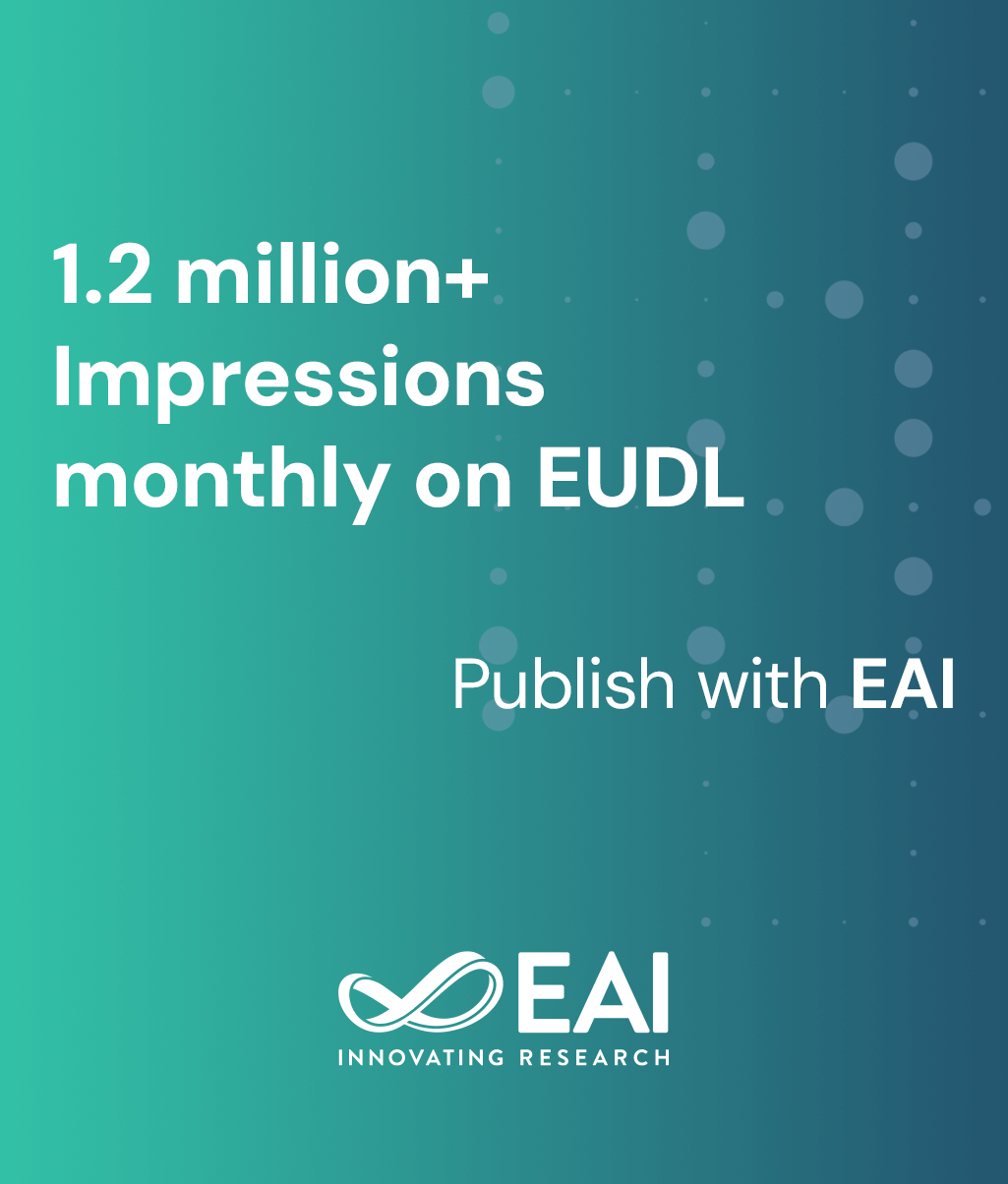
Research Article
A Many-Valued Empirical Machine for Thyroid Dysfunction Assessment
@INPROCEEDINGS{10.1007/978-3-030-16447-8_5, author={Sofia Santos and M. Martins and Henrique Vicente and M. Barroca and Fernando Calisto and C\^{e}sar Gama and Jorge Ribeiro and Joana Machado and Liliana \^{A}vidos and Nuno Ara\^{u}jo and Almeida Dias and Jos\^{e} Neves}, title={A Many-Valued Empirical Machine for Thyroid Dysfunction Assessment}, proceedings={Intelligent Technologies for Interactive Entertainment. 10th EAI International Conference, INTETAIN 2018, Guimar\"{a}es, Portugal, November 21-23, 2018, Proceedings}, proceedings_a={INTETAIN}, year={2019}, month={4}, keywords={Thyroid dysfunction Knowledge Representation and Reasoning Artificial Neural Networks Entropy Logic Programming Many-Valued Empirical Machine}, doi={10.1007/978-3-030-16447-8_5} }
- Sofia Santos
M. Martins
Henrique Vicente
M. Barroca
Fernando Calisto
César Gama
Jorge Ribeiro
Joana Machado
Liliana Ávidos
Nuno Araújo
Almeida Dias
José Neves
Year: 2019
A Many-Valued Empirical Machine for Thyroid Dysfunction Assessment
INTETAIN
Springer
DOI: 10.1007/978-3-030-16447-8_5
Abstract
is a clinical condition that affects thyroid behaviour and is reported to be the most common in all endocrine disorders. It is a multiple factorial pathology condition due to the high incidence of hypothyroidism and hyperthyroidism, which is becoming a serious health problem requiring a detailed study for early diagnosis and monitoring. Understanding the prevalence and risk factors of thyroid disease can be very useful to identify patients for screening and/or follow-up and to minimize their collateral effects. Thus, this paper describes the development of a decision support system that aims to help physicians in the decision-making process regarding thyroid dysfunction assessment. The proposed problem-solving method is based on a symbolic/sub-symbolic line of logical formalisms that have been articulated as an approach to data processing, complemented by an unusual approach to that takes into account the data elements entropic states. The model performs well in the thyroid dysfunction assessment with an accuracy ranging between 93.2% and 96.9%.