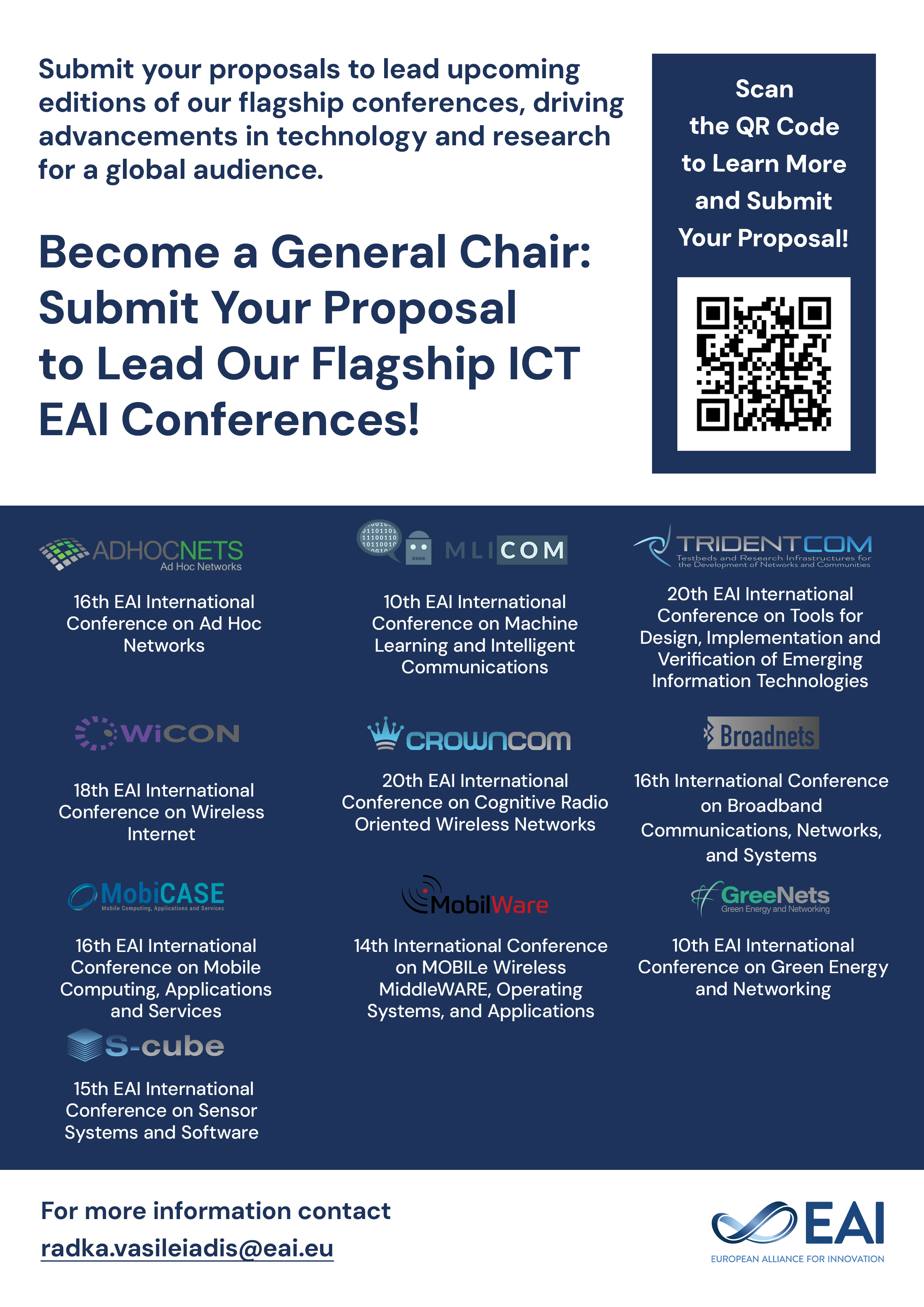
Research Article
Exploring Novel Methodology for Classifying Cognitive Workload
@INPROCEEDINGS{10.1007/978-3-030-16447-8_11, author={Seth Siriya and Martin Lochner and Andreas Duenser and Ronnie Taib}, title={Exploring Novel Methodology for Classifying Cognitive Workload}, proceedings={Intelligent Technologies for Interactive Entertainment. 10th EAI International Conference, INTETAIN 2018, Guimar\"{a}es, Portugal, November 21-23, 2018, Proceedings}, proceedings_a={INTETAIN}, year={2019}, month={4}, keywords={Cognitive load Galvanic Skin Response Electrodermal Activity Psycho-physiology Analytics Machine learning Decision response task}, doi={10.1007/978-3-030-16447-8_11} }
- Seth Siriya
Martin Lochner
Andreas Duenser
Ronnie Taib
Year: 2019
Exploring Novel Methodology for Classifying Cognitive Workload
INTETAIN
Springer
DOI: 10.1007/978-3-030-16447-8_11
Abstract
This paper describes our work in extracting useful cognitive load classification information from a relatively simple and non-invasive physiological measurement technique, with application in a range of Human Factors and Human-Computer Interaction contexts. We employ novel methodologies, including signal processing, machine learning and genetic algorithms, to classify Galvanic Skin Response/Electrodermal Activity (GSR/EDA) signals during performance of a customised game task () in high- and low-workload conditions. Our results reveal that Support Vector Machine Linear was the most successful technique for classifying the level of cognitive load that an operator is undergoing during easy, medium, and difficult operation conditions. This methodology has the advantage of applicability in situations, where other cognitive load measurement methodologies are problematic due to sampling delay (e.g. questionnaires), or difficulty of implementation (e.g. other psych-physiological measures). A proposed cognitive load classification pipeline for real-time implementation and its use in human factors contexts is discussed.