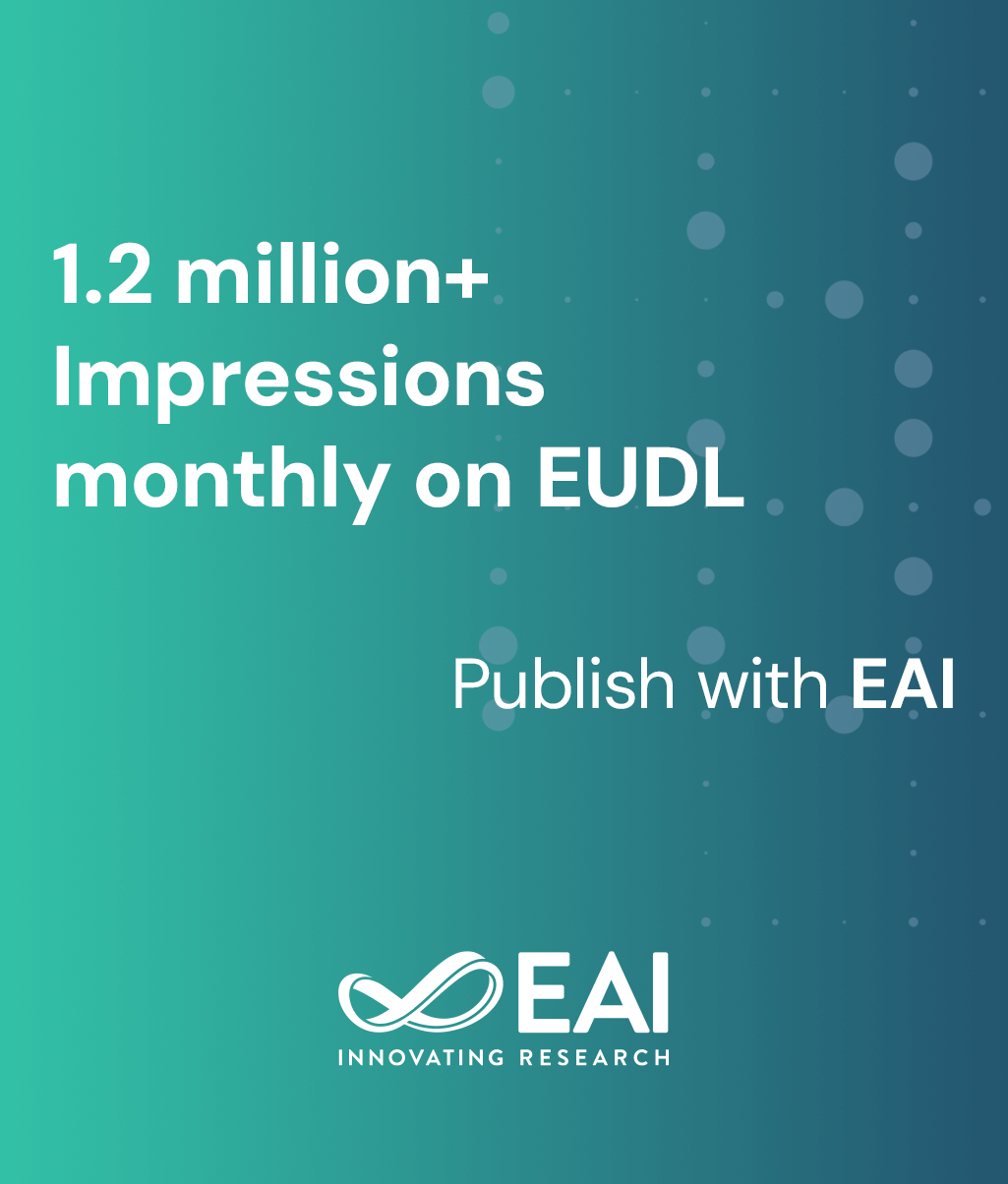
Research Article
Classification and Prediction of Arrhythmias from Electrocardiograms Patterns Based on Empirical Mode Decomposition and Neural Network
@INPROCEEDINGS{10.1007/978-3-030-16042-5_17, author={Abdoul-Dalibou Abdou and Ndeye Ngom and Oumar Niang}, title={Classification and Prediction of Arrhythmias from Electrocardiograms Patterns Based on Empirical Mode Decomposition and Neural Network}, proceedings={e-Infrastructure and e-Services for Developing Countries. 10th EAI International Conference, AFRICOMM 2018, Dakar, Senegal, November 29-30, 2019, Proceedings}, proceedings_a={AFRICOMM}, year={2019}, month={3}, keywords={ECG classification Neural networks Predictive models Empirical mode decomposition Arrhythmia}, doi={10.1007/978-3-030-16042-5_17} }
- Abdoul-Dalibou Abdou
Ndeye Ngom
Oumar Niang
Year: 2019
Classification and Prediction of Arrhythmias from Electrocardiograms Patterns Based on Empirical Mode Decomposition and Neural Network
AFRICOMM
Springer
DOI: 10.1007/978-3-030-16042-5_17
Abstract
Diagnosis of heart disease rests essentially on the analysis of the statistical, morphological, temporal, or frequency properties of ECG. Data analytical techniques are often needed for the identification, the extraction of relevant information, the discovery of meaningful patterns and new threads of knowledge from biomedical data. However for cardio-vascular diseases, despite the rapid increase in the collection of methods proposed, research communities still have difficulties in delivering applications for clinical practice. In this paper we propose hybrid model to advance the understanding of arrhythmias from electrocardiograms patterns. Adaptive analysis based on empirical Mode Decomposition (EMD) is first carried out to perform signal denoising and the detection of main events presented in the electrocardiograms (Ecg). Then, binary classification is performed using Neural Network model. However in this work, the Ecg R-peak detection method, the classification algorithm are improved and the chart flow include a predictive step. Indeed, the classification outputs are used to perform prediction of cardiac rhythm pattern. The proposed model is illustrated using the MIT-BIH database, compared to other methods and discussed. The obtained results are very promising.