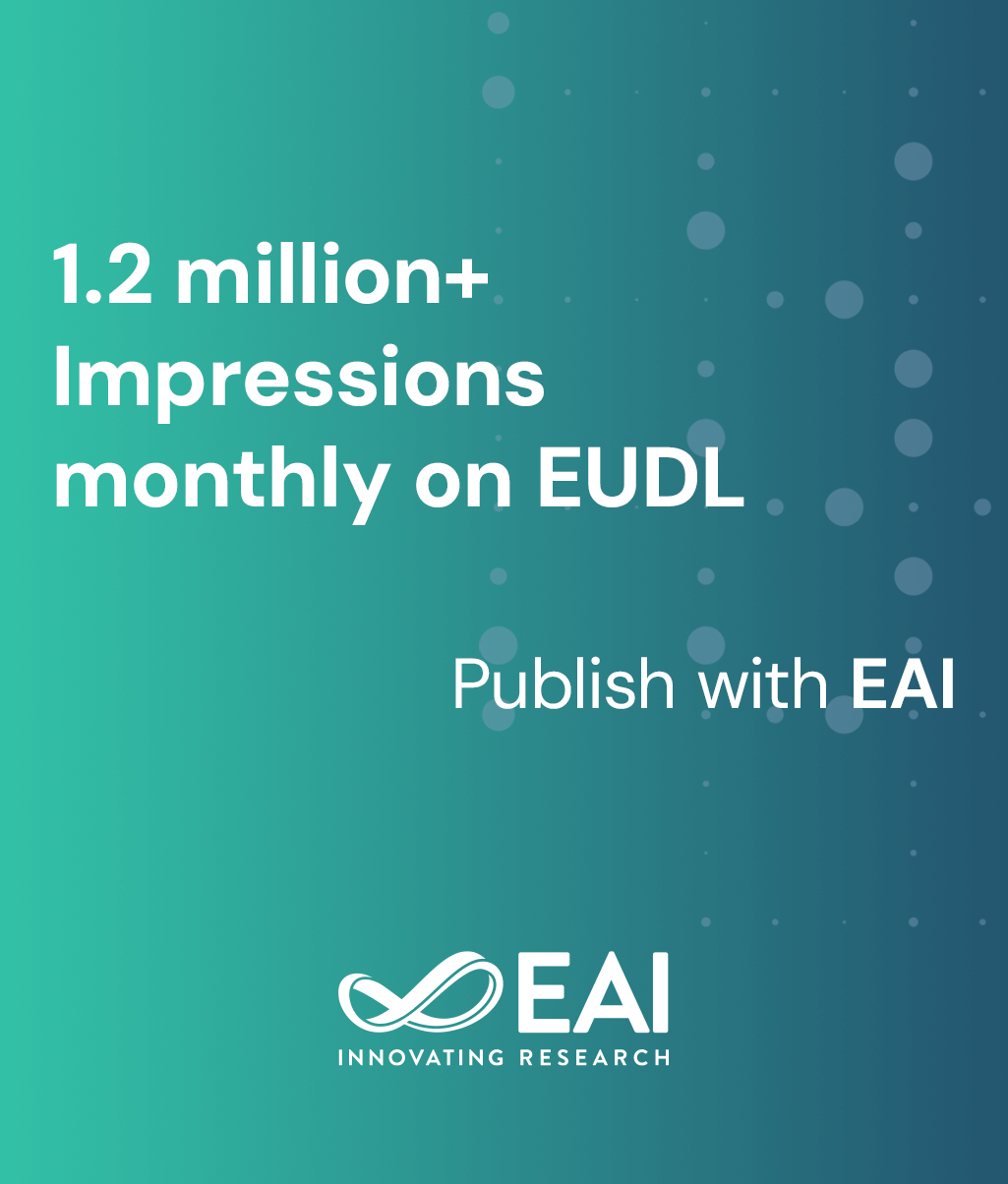
Research Article
Wind Energy Conversion System Model Identification and Validation
@INPROCEEDINGS{10.1007/978-3-030-15357-1_29, author={Endalew Ayenew and Mulugeta Debebe and Beza Nekatibeb and Venkata Komanapalli}, title={Wind Energy Conversion System Model Identification and Validation}, proceedings={Advances of Science and Technology. 6th EAI International Conference, ICAST 2018, Bahir Dar, Ethiopia, October 5-7, 2018, Proceedings}, proceedings_a={ICAST}, year={2019}, month={3}, keywords={WECS Model identification BJ33221 nlarx121}, doi={10.1007/978-3-030-15357-1_29} }
- Endalew Ayenew
Mulugeta Debebe
Beza Nekatibeb
Venkata Komanapalli
Year: 2019
Wind Energy Conversion System Model Identification and Validation
ICAST
Springer
DOI: 10.1007/978-3-030-15357-1_29
Abstract
Wind energy conversion system (WECS) is complex because of wind speed varies in time and space. Model identification is required to represent its dynamics for real-time implementation. In this paper a doubly-fed induction generator (DFIG) WECS is used. Different model structures are generated and simulated using MATLAB/SIMULINK. The models are generated using both nonlinear and linear system identification tool boxes. Linear system identification toolbox generates both model structure and model parameters; whereas the nonlinear system identification tool generates only the system model structures. From linear models, the BJ33221 model has better performance with best fit of 74.78%, final prediction error (FPE) value of 0.0445 and mean square error (MSE) is 0.04265. ARX211 model structure provides best fit of 74.39%, FPE of 0.0453, and MSE is 0.04465. This study shows as model order increases, the best fit value too, but the system become more complex. The nonlinear models have better performance than the linear models. The nlarx121 model structure provides the best fit of 96.43% and MSE of 0.0322, with other technique for its model parameters estimation. The output residuals are within the confident range (0.2 to −0.2), indicating the model structure was validated.