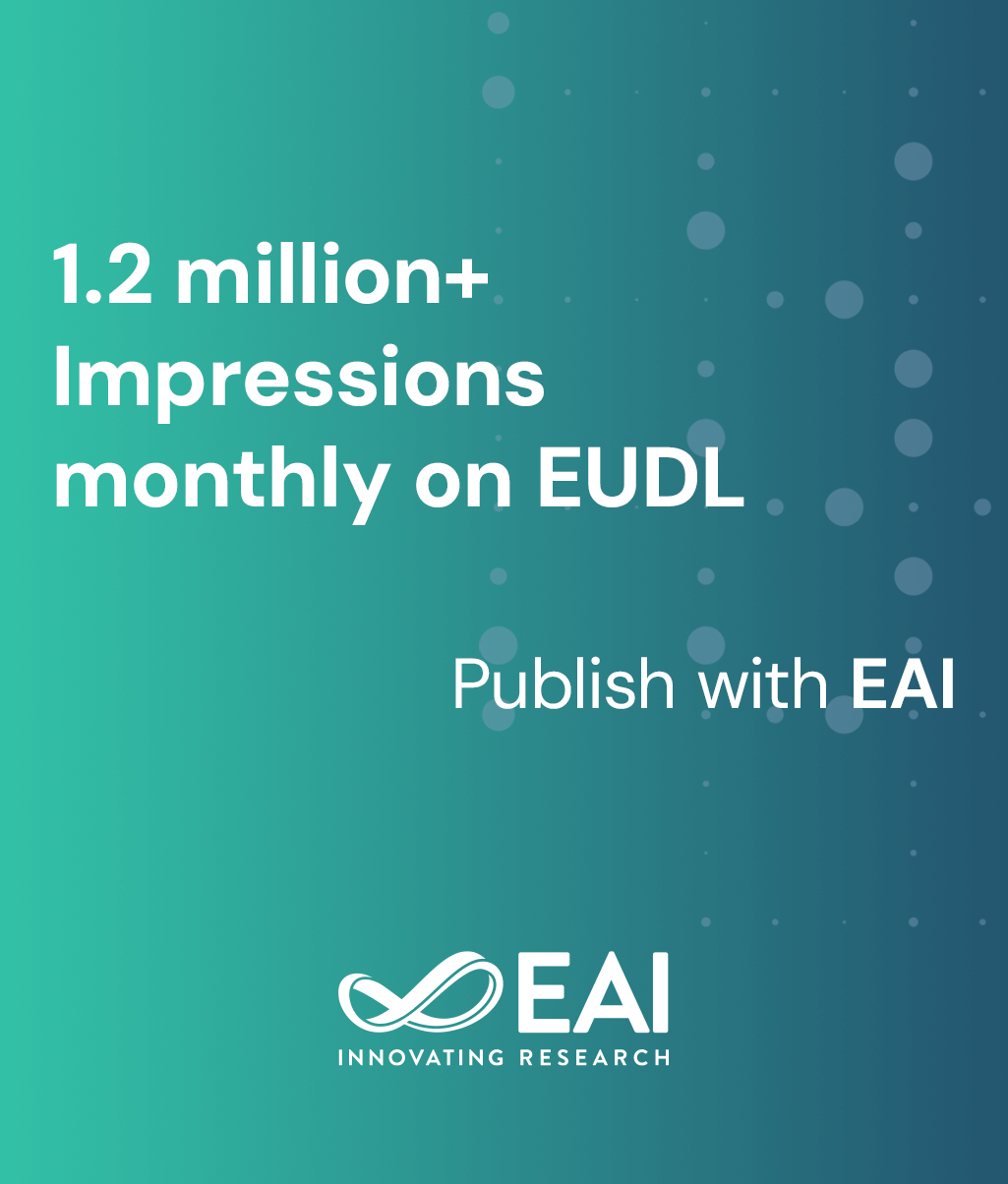
Research Article
Multi-font Printed Amharic Character Image Recognition: Deep Learning Techniques
504 downloads
@INPROCEEDINGS{10.1007/978-3-030-15357-1_27, author={Birhanu Hailu Belay and Gebeyehu Belay and Tewodros Amberbir Hebtegebrial and Didier Stricker}, title={Multi-font Printed Amharic Character Image Recognition: Deep Learning Techniques}, proceedings={Advances of Science and Technology. 6th EAI International Conference, ICAST 2018, Bahir Dar, Ethiopia, October 5-7, 2018, Proceedings}, proceedings_a={ICAST}, year={2019}, month={3}, keywords={Amharic script Deep convolutional neural network Deep learning Printed Amharic character Pattern recognition OCR OCRopus Visual Geometry Group}, doi={10.1007/978-3-030-15357-1_27} }
- Birhanu Hailu Belay
Gebeyehu Belay
Tewodros Amberbir Hebtegebrial
Didier Stricker
Year: 2019
Multi-font Printed Amharic Character Image Recognition: Deep Learning Techniques
ICAST
Springer
DOI: 10.1007/978-3-030-15357-1_27
Abstract
In this paper, we propose a technique to recognize multi-font printed Amharic character images using deep convolutional neural network (DCNN) which is one of the recent techniques adopted from the deep learning community. Experiments were done on 86,715 Amharic character images with different level of degradation and multiple font types. The proposed method has fewer pre-processing steps and outperforms the standard approach used in classical machine learning techniques. We systematically evaluated the performance of the recognition model and achieved 96.02% of character recognition accuracy.
Copyright © 2018–2025 ICST