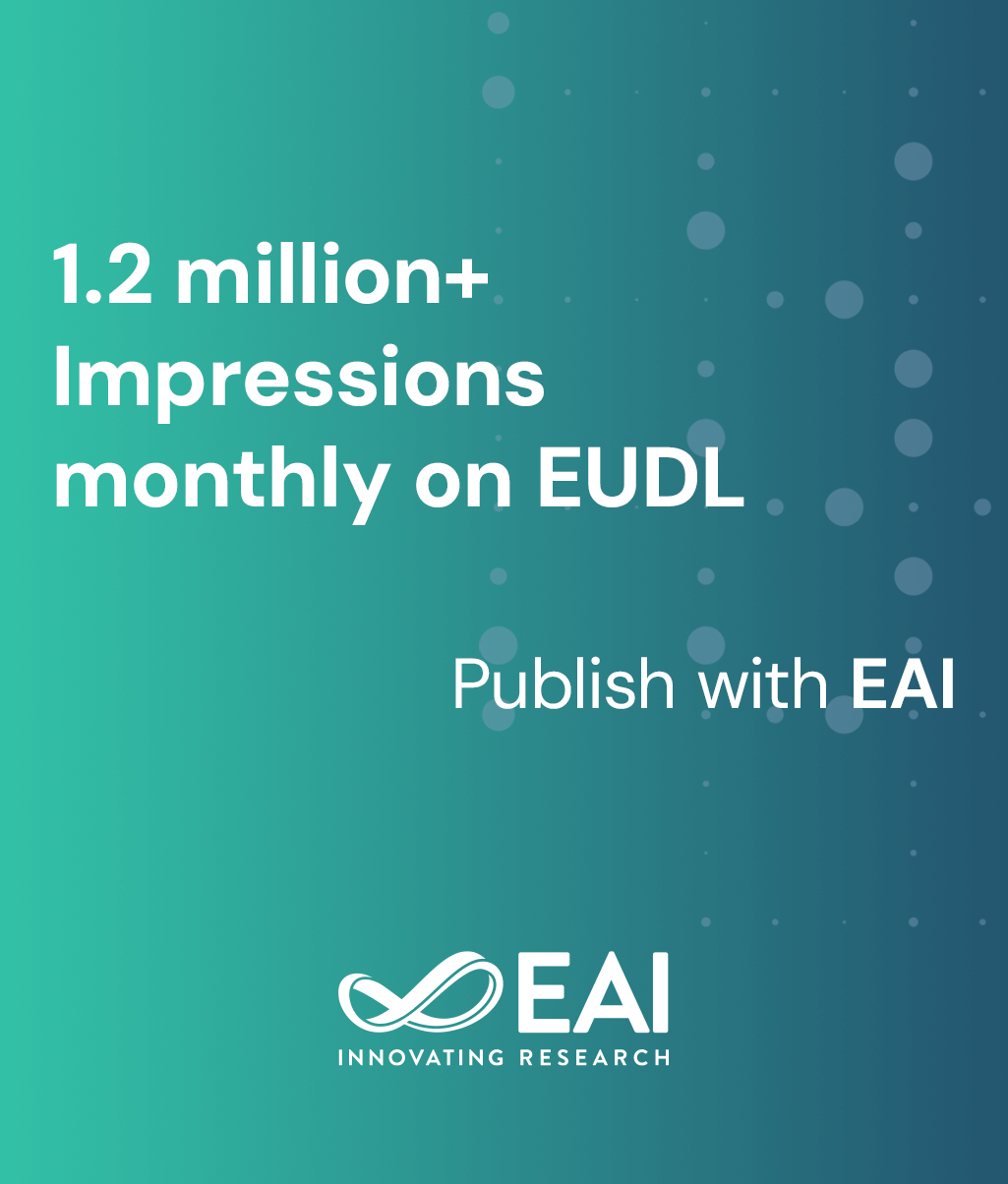
Research Article
Prediction of Journey Destination for Travelers of Urban Public Transport: A Comparison Model Study
@INPROCEEDINGS{10.1007/978-3-030-14757-0_9, author={Vera Costa and T\~{a}nia Fontes and Jos\^{e} Borges and Teresa Dias}, title={Prediction of Journey Destination for Travelers of Urban Public Transport: A Comparison Model Study}, proceedings={Intelligent Transport Systems, From Research and Development to the Market Uptake. Second EAI International Conference, INTSYS 2018, Guimar\"{a}es, Portugal, November 21--23, 2018, Proceedings}, proceedings_a={INTSYS}, year={2019}, month={2}, keywords={Urban public transportation Travel patterns Journey destination Prediction models}, doi={10.1007/978-3-030-14757-0_9} }
- Vera Costa
Tânia Fontes
José Borges
Teresa Dias
Year: 2019
Prediction of Journey Destination for Travelers of Urban Public Transport: A Comparison Model Study
INTSYS
Springer
DOI: 10.1007/978-3-030-14757-0_9
Abstract
In public transport, smart card-based ticketing system allows to redesign the UPT network, by providing customized transport services, or incentivize travelers to change specific patterns. However, in open systems, to develop personalized connections the journey destination must be known before the end of the travel. Thus, to obtain that knowledge, in this study three models (Top-K, NB, and J48) were applied using different groups of travelers of an urban public transport network located in a medium-sized European metropolitan area (Porto, Portugal). Typical travelers were selected from the segmentation of transportation card signatures, and groups were defined based on the traveler age or economic conditions. The results show that is possible to predict the journey’s destination based on the past with an accuracy rate that varies, on average, from 20% in the worst scenarios to 65% in the best.