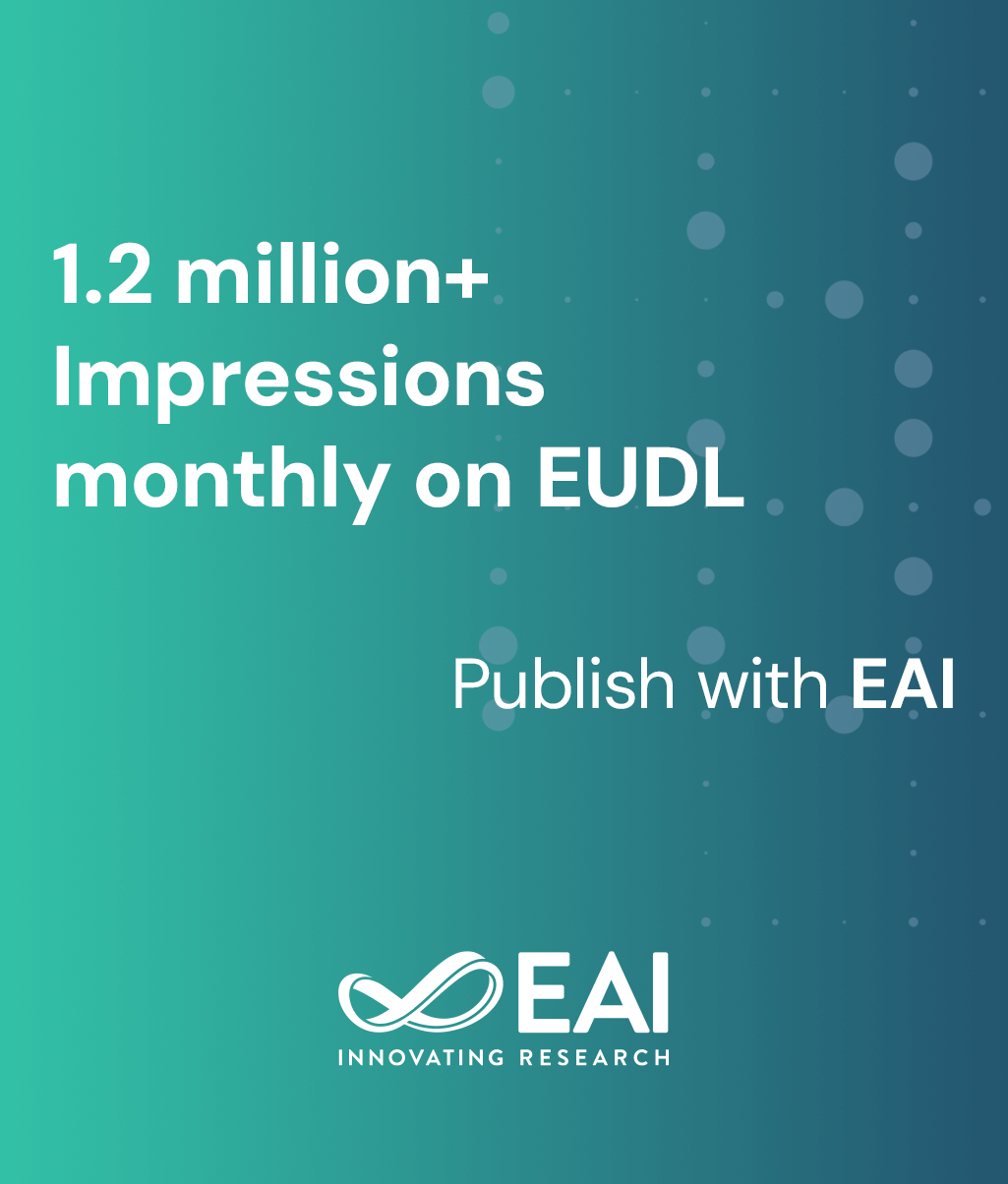
Research Article
Video Captioning Using Hierarchical LSTM and Text-Based Sliding Window
@INPROCEEDINGS{10.1007/978-3-030-14657-3_6, author={Huanhou Xiao and Jinglun Shi}, title={Video Captioning Using Hierarchical LSTM and Text-Based Sliding Window}, proceedings={IoT as a Service. 4th EAI International Conference, IoTaaS 2018, Xi’an, China, November 17--18, 2018, Proceedings}, proceedings_a={IOTAAS}, year={2019}, month={3}, keywords={Multimedia Sentence semantics Long short term memory Sliding window Video captioning}, doi={10.1007/978-3-030-14657-3_6} }
- Huanhou Xiao
Jinglun Shi
Year: 2019
Video Captioning Using Hierarchical LSTM and Text-Based Sliding Window
IOTAAS
Springer
DOI: 10.1007/978-3-030-14657-3_6
Abstract
Automatically describing video content with natural language has been attracting a lot of attention in multimedia community. However, most existing methods only use the word-level cross entropy loss to train the model, while ignoring the relationship between visual content and sentence semantics. In addition, during the decoding stage, the resulting models are used to predict one word at a time, and by feeding the generated word back as input at the next time step. Nevertheless, the other generated words are not fully exploited. As a result, the model is easy to “run off” if the last generated word is ambiguous. To tackle these issues, we propose a novel framework consisting of hierarchical long short term memory and text-based sliding window (HLSTM-TSW), which not only optimizes the model at word level, but also enhances the semantic relationship between the visual content and the entire sentence during training. Moreover, a sliding window is used to focus on k previously generated words when predicting the next word, so that our model can make use of more useful information to further improve the accuracy of forecast. Experiments on the benchmark dataset YouTube2Text demonstrate that our method which only uses single feature achieves superior or even better results than the state-of-the-art baselines for video captioning.