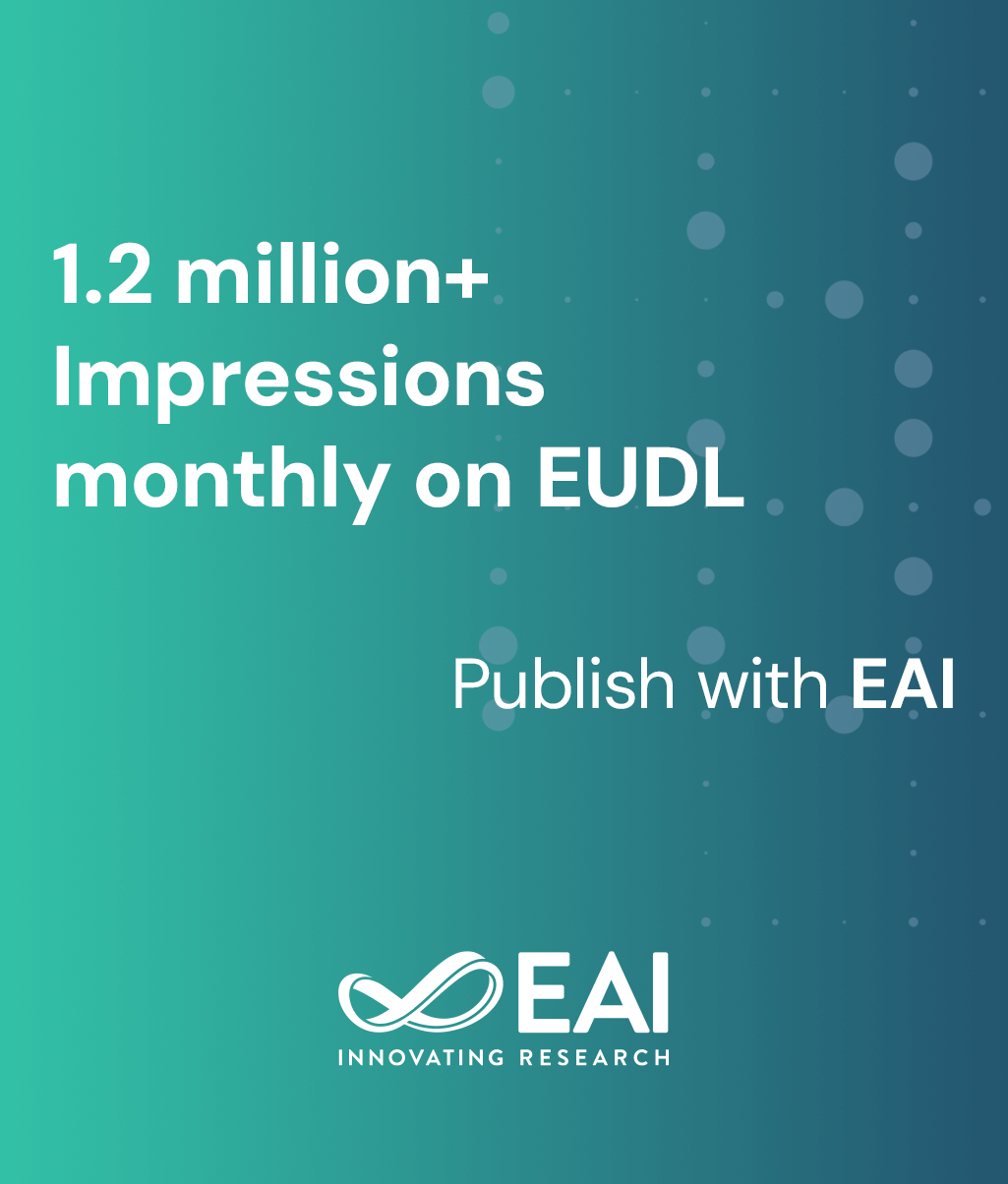
Research Article
Reinforcement Learning-Based Cooperative Spectrum Sensing
@INPROCEEDINGS{10.1007/978-3-030-14657-3_16, author={Wenli Ning and Xiaoyan Huang and Fan Wu and Supeng Leng and Lixiang Ma}, title={Reinforcement Learning-Based Cooperative Spectrum Sensing}, proceedings={IoT as a Service. 4th EAI International Conference, IoTaaS 2018, Xi’an, China, November 17--18, 2018, Proceedings}, proceedings_a={IOTAAS}, year={2019}, month={3}, keywords={Spectrum sensing Reinforcement learning Cooperative sensing Q-Learning Multi-armed bandit}, doi={10.1007/978-3-030-14657-3_16} }
- Wenli Ning
Xiaoyan Huang
Fan Wu
Supeng Leng
Lixiang Ma
Year: 2019
Reinforcement Learning-Based Cooperative Spectrum Sensing
IOTAAS
Springer
DOI: 10.1007/978-3-030-14657-3_16
Abstract
In cognitive radio (CR) networks, the detection result of a single user is susceptible due to shadowing and multipath fading. In order to find an idle channel, the secondary user (SU) should detect channels in sequence, while the sequential detection may cause excessive overhead and access delay. In this paper, a reinforcement learning (RL) based cooperative sensing scheme is proposed to help SU determine the detection order of channels and select the cooperative sensing partner, so as to reduce the overhead and access delay as well improve the detection efficiency in spectrum sensing. By applying Q-Learning, each SU forms a dynamic priority list of the channels based on neighbors’ sensing results and recent act-observation. When a call arrives at a SU, the SU scans the channel in list order. To improve the detection efficiency, the SU can select a neighbor with potential highest detection probability as cooperative partner using multi-armed bandit (MAB) algorithm. Simulation results show that the proposed scheme can significantly reduce the scanning overhead and access delay, and improve the detection efficiency.