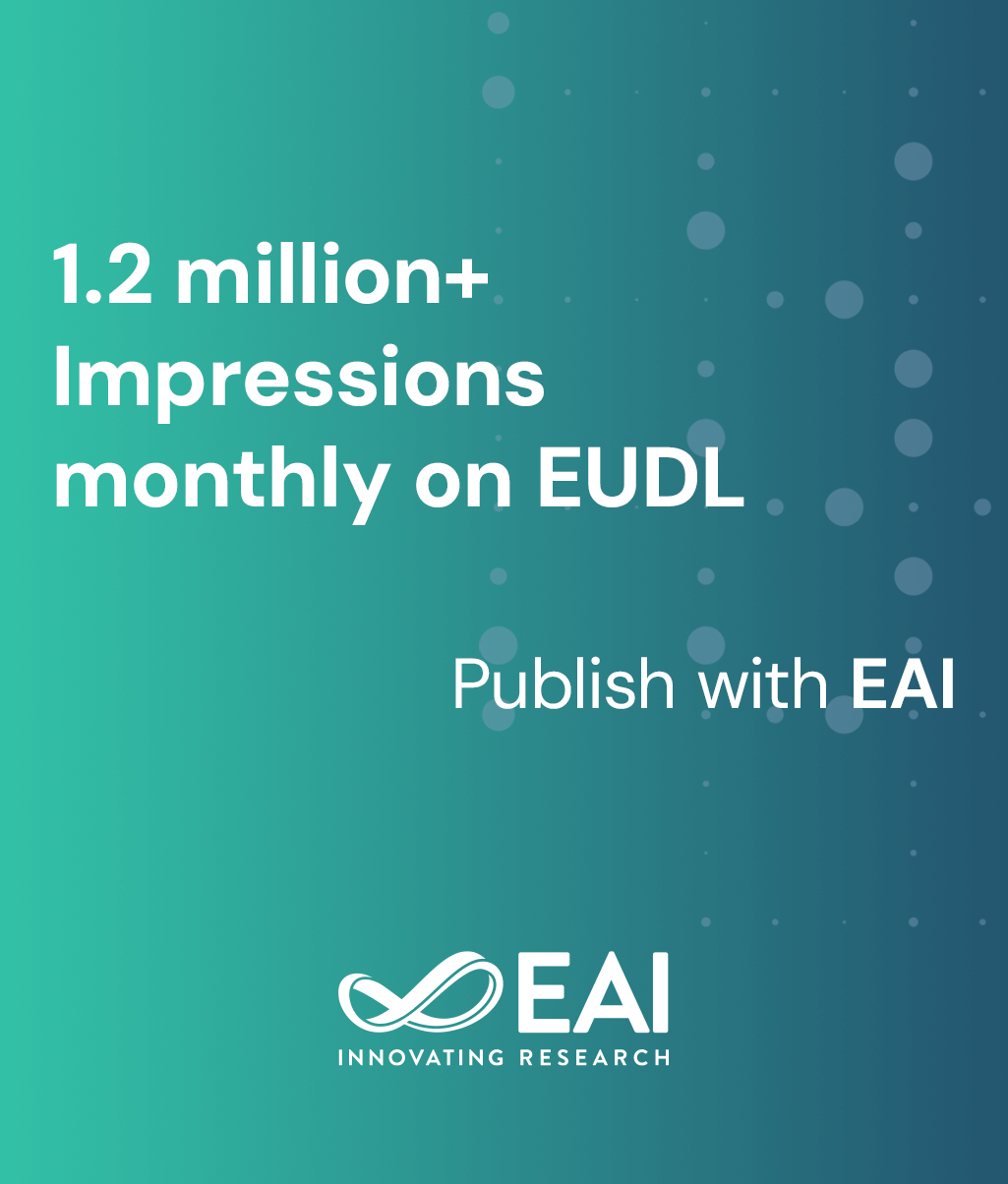
Research Article
A Location Spoofing Detection Method for Social Networks (Short Paper)
@INPROCEEDINGS{10.1007/978-3-030-12981-1_9, author={Chaoping Ding and Ting Wu and Tong Qiao and Ning Zheng and Ming Xu and Yiming Wu and Wenjing Xia}, title={A Location Spoofing Detection Method for Social Networks (Short Paper)}, proceedings={Collaborative Computing: Networking, Applications and Worksharing. 14th EAI International Conference, CollaborateCom 2018, Shanghai, China, December 1-3, 2018, Proceedings}, proceedings_a={COLLABORATECOM}, year={2019}, month={2}, keywords={Location spoofing Social networks Semantic analysis}, doi={10.1007/978-3-030-12981-1_9} }
- Chaoping Ding
Ting Wu
Tong Qiao
Ning Zheng
Ming Xu
Yiming Wu
Wenjing Xia
Year: 2019
A Location Spoofing Detection Method for Social Networks (Short Paper)
COLLABORATECOM
Springer
DOI: 10.1007/978-3-030-12981-1_9
Abstract
It is well known that check-in data from location-based social networks (LBSN) can be used to predict human movement. However, there are large discrepancies between check-in data and actual user mobility, because users can easily spoof their location in LBSN. The act of location spoofing refers to intentionally making false location, leading to a negative impact both on the credibility of location-based social networks and the reliability of spatial-temporal data. In this paper, a location spoofing detection method in social networks is proposed. First, Latent Dirichlet Allocation (LDA) model is used to learn the topics of users by mining user-generated microblog information, based on this a similarity matrix associated with the venue is calculated. And the venue visiting probability is computed based on user historical check-in data by using Bayes model. Then, the similarity value and visiting probability is combined to quantize the probability of location spoofing. Experiments on a large scale and real-world LBSN dataset collected from Weibo show that the proposed approach can effectively detect certain types of location spoofing.