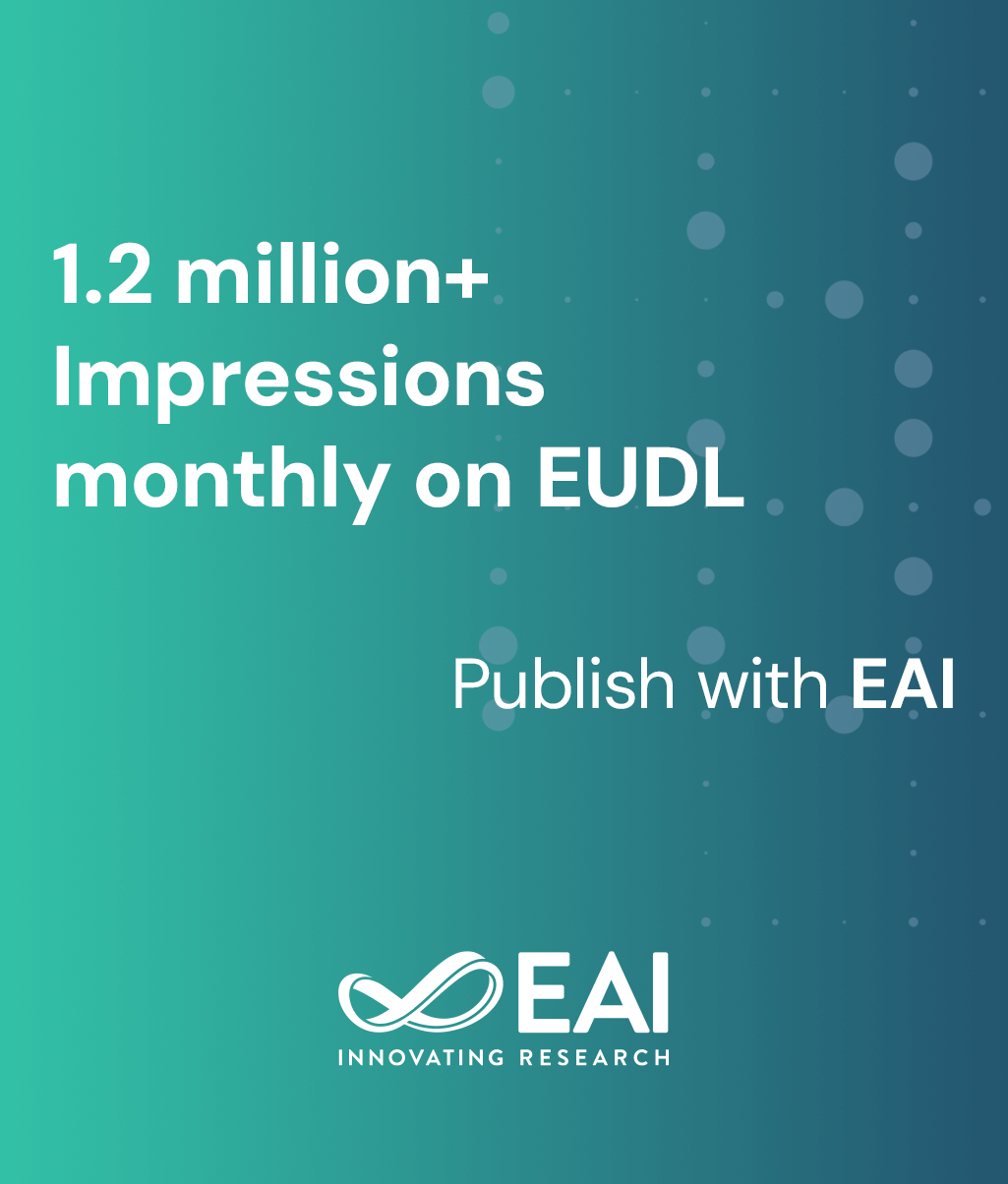
Research Article
Recommending More Suitable Music Based on Users’ Real Context
@INPROCEEDINGS{10.1007/978-3-030-12981-1_8, author={Qing Yang and Le Zhan and Li Han and Jingwei Zhang and Zhongqin Bi}, title={Recommending More Suitable Music Based on Users’ Real Context}, proceedings={Collaborative Computing: Networking, Applications and Worksharing. 14th EAI International Conference, CollaborateCom 2018, Shanghai, China, December 1-3, 2018, Proceedings}, proceedings_a={COLLABORATECOM}, year={2019}, month={2}, keywords={Music recommendation Feature selection User context}, doi={10.1007/978-3-030-12981-1_8} }
- Qing Yang
Le Zhan
Li Han
Jingwei Zhang
Zhongqin Bi
Year: 2019
Recommending More Suitable Music Based on Users’ Real Context
COLLABORATECOM
Springer
DOI: 10.1007/978-3-030-12981-1_8
Abstract
Music recommendation is an popular function for personalized services and smart applications since it focuses on discovering users’ leisure preference. The traditional music recommendation strategy captured users’ music preference by analyzing their historical behaviors to conduct personalized recommendation. However, users’ current states, such as in busy working or in a leisure travel, etc., have an important influence on their music enjoyment. Usually, those existing methods only focus on pushing their favorite music to users, which may be not the most suitable for current scenarios. Users’ current states should be taken into account to make more perfect music recommendation. Considering the above problem, this paper proposes a music recommendation method by considering both users’ current states and their historical behaviors. First, a feature selection process based on ReliefF method is applied to discover the optimal features for the following recommendation. Second, we construct different feature groups according to the feature weights and introduce Naive Bayes model and Adaboost algorithm to train these feature groups, which will output a base classifier for each feature group. Finally, a majority voting strategy decides the optimal music type and each user will be recommended more suitable music based on their current context. The experiments on the real datasets show the effectiveness of the proposed method.