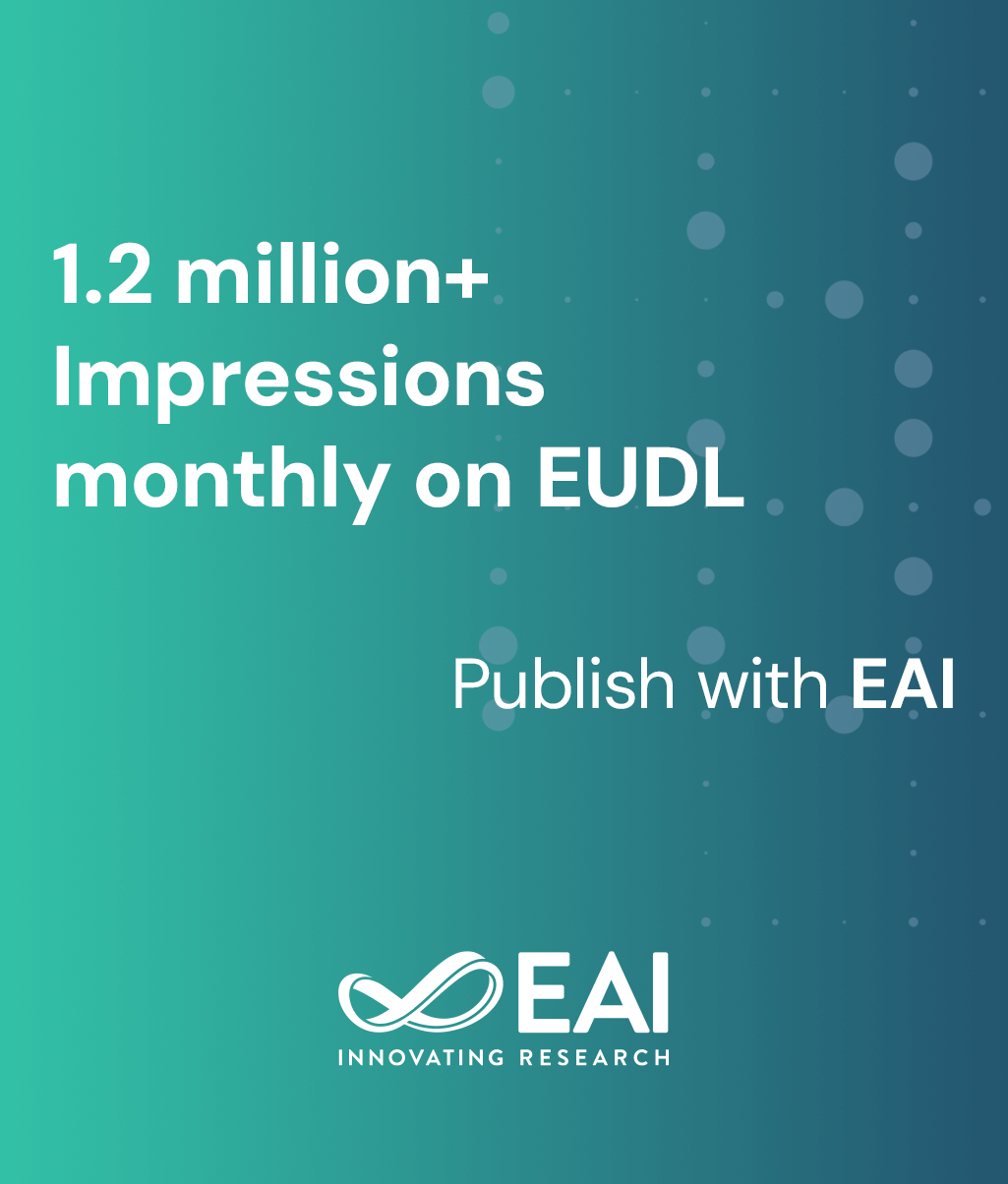
Research Article
Worker Recommendation with High Acceptance Rates in Collaborative Crowdsourcing Systems
@INPROCEEDINGS{10.1007/978-3-030-12981-1_4, author={Mingchu Li and Xiaomei Sun and Xing Jin and Linlin Tian}, title={Worker Recommendation with High Acceptance Rates in Collaborative Crowdsourcing Systems}, proceedings={Collaborative Computing: Networking, Applications and Worksharing. 14th EAI International Conference, CollaborateCom 2018, Shanghai, China, December 1-3, 2018, Proceedings}, proceedings_a={COLLABORATECOM}, year={2019}, month={2}, keywords={Collaborative crowdsourcing Trust Worker recommendation Matrix factorization Task matching}, doi={10.1007/978-3-030-12981-1_4} }
- Mingchu Li
Xiaomei Sun
Xing Jin
Linlin Tian
Year: 2019
Worker Recommendation with High Acceptance Rates in Collaborative Crowdsourcing Systems
COLLABORATECOM
Springer
DOI: 10.1007/978-3-030-12981-1_4
Abstract
Crowdsourcing has emerged as a popular Internet-based collaboration paradigm, in which tasks published by requesters can be economically and efficiently accomplished by crowd workers. To ensure the quality of service (QoS) provided by crowd workers, requesters are more likely to assign tasks to trustworthy workers, therefore, trust have played an important role in the design of worker recommendation mechanisms in crowdsourcing systems. Most existing studies focus on the trust that requesters place on workers, however, which would suffer the low-acceptance problem because crowd workers would refuse to participate in tasks published by low-trustworthy requesters with a great probability. In order to address the low-acceptance problem, in this paper, by using biased matrix factorization, we proposed a novel worker recommendation mechanism which can evaluate mutual trust relationship between requesters and workers. And also, to accurately measure the matching degree between tasks and workers, a comprehensive and practical task matching mechanism has been presented by incorporating time matching, skill matching, payment matching, and location matching. Finally, extensive simulations and real data experiments highlight the performance of our proposed worker recommendation mechanism.