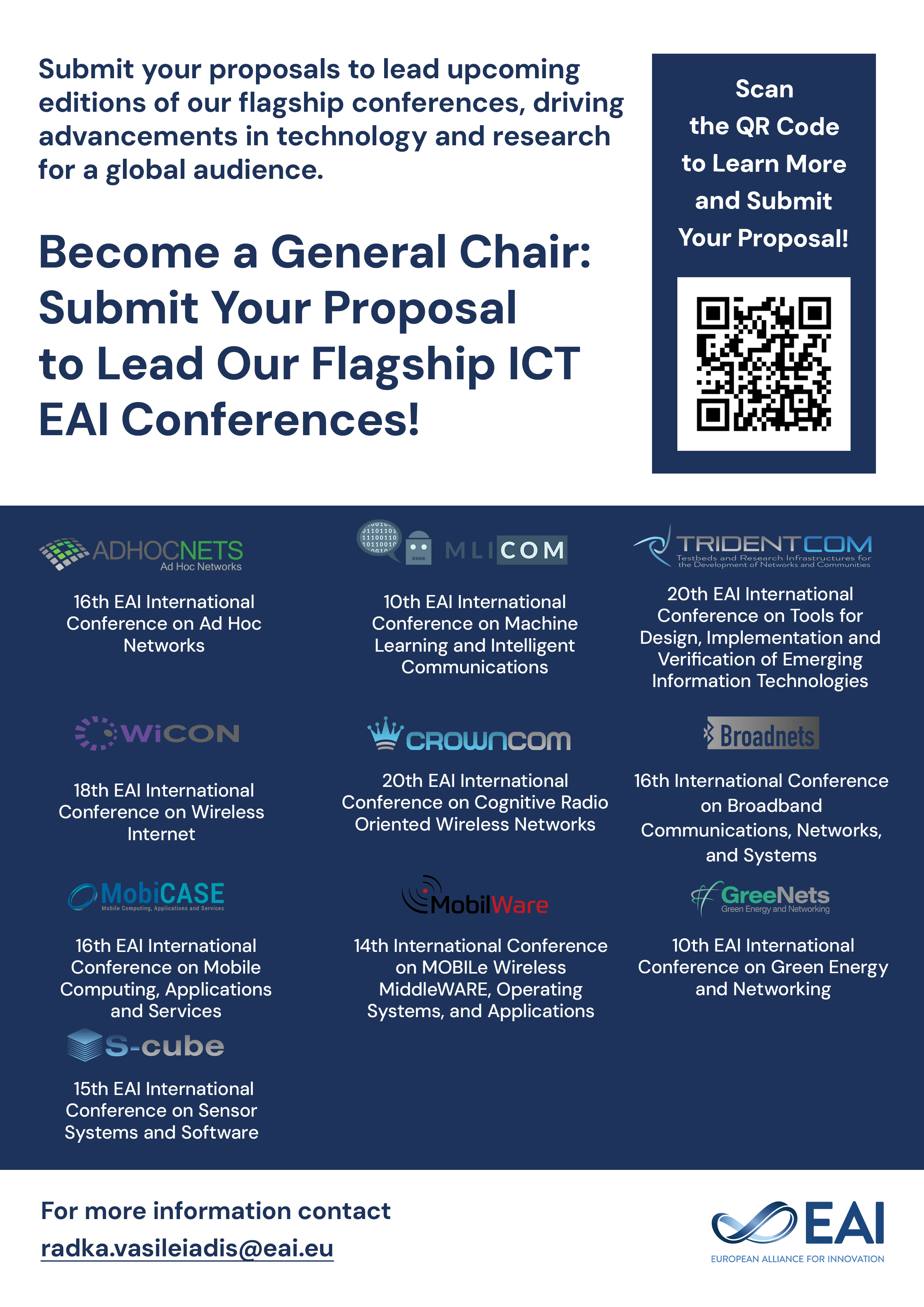
Research Article
A 2D Transform Based Distance Function for Time Series Classification
@INPROCEEDINGS{10.1007/978-3-030-12981-1_38, author={Cun Ji and Xiunan Zou and Yupeng Hu and Shijun Liu}, title={A 2D Transform Based Distance Function for Time Series Classification}, proceedings={Collaborative Computing: Networking, Applications and Worksharing. 14th EAI International Conference, CollaborateCom 2018, Shanghai, China, December 1-3, 2018, Proceedings}, proceedings_a={COLLABORATECOM}, year={2019}, month={2}, keywords={Internet of Things Data mining Time series classification 2D transform Distance function}, doi={10.1007/978-3-030-12981-1_38} }
- Cun Ji
Xiunan Zou
Yupeng Hu
Shijun Liu
Year: 2019
A 2D Transform Based Distance Function for Time Series Classification
COLLABORATECOM
Springer
DOI: 10.1007/978-3-030-12981-1_38
Abstract
Along with the arrival of Industry 4.0 era, time series classification (TSC) has attracted a lot of attention in the last decade. The high dimensionality, high feature correlation and typically high levels of noise that found in time series bring great challenges to TSC. Among TSC algorithms, the 1NN classifier has been shown as effective and difficult to beat. The core of the 1NN classifier is the distance function. The large majority of TSC have concentrated on alternative distance functions. In this paper, a two-dimensional (2D) transform based distance (2DTbD) function is proposed. There are three steps in 2DTbD. Firstly, we convert time series to 2D space by turning time series around the coordinate origin. Then we calculate distances of each dimension. Finally, we ensemble distances in 2D space to get the final time series distance. Our distance function raises the accuracy rate through the fusion of 2D information. Experimental results demonstrate that the classification accuracy can be improved by 2DTbD.