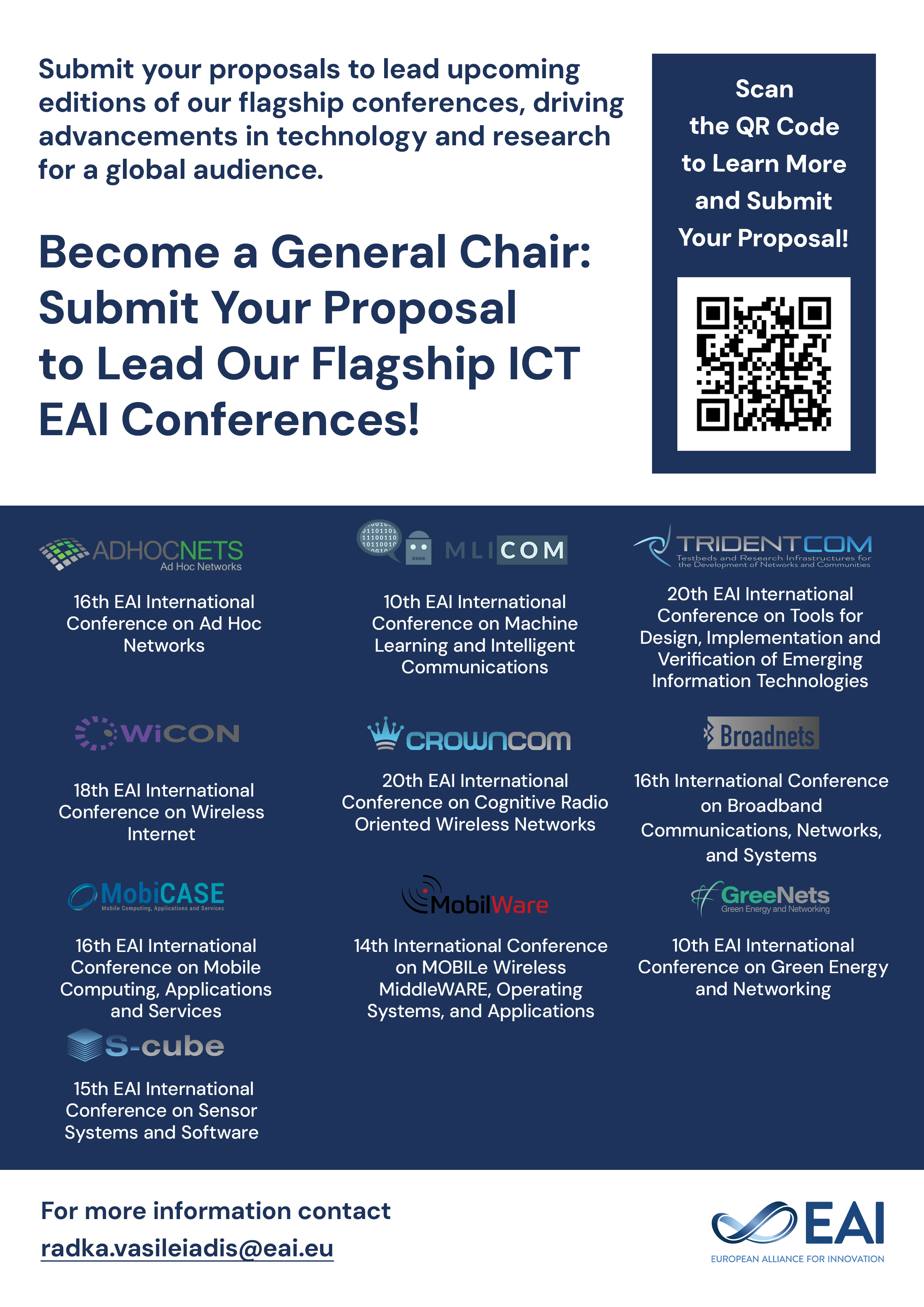
Research Article
Booter Blacklist Generation Based on Content Characteristics
@INPROCEEDINGS{10.1007/978-3-030-12981-1_37, author={Wang Zhang and Xu Bai and Chanjuan Chen and Zhaolin Chen}, title={Booter Blacklist Generation Based on Content Characteristics}, proceedings={Collaborative Computing: Networking, Applications and Worksharing. 14th EAI International Conference, CollaborateCom 2018, Shanghai, China, December 1-3, 2018, Proceedings}, proceedings_a={COLLABORATECOM}, year={2019}, month={2}, keywords={Booter service Feature selection Text classification}, doi={10.1007/978-3-030-12981-1_37} }
- Wang Zhang
Xu Bai
Chanjuan Chen
Zhaolin Chen
Year: 2019
Booter Blacklist Generation Based on Content Characteristics
COLLABORATECOM
Springer
DOI: 10.1007/978-3-030-12981-1_37
Abstract
Distributed Denial of Service (DDoS) attacks-as-a-service, known as Booter or Stresser, is convenient and low-priced for ordinary people to launch DDoS attacks. It makes DDoS attacks even more rampant. However, until now there is not much research on Booter and little acquaintance with their backend infrastructure, customers, business, etc. In this paper, we present a new method which focuses on the content (text) characteristics on Booters websites and selects more discriminative features between Booter and non-Booter to identify Booters more effectively in the Internet. The experimental results show that the classification accuracy of distinguishing Booter and non-Booter websites is 98.74%. In addition, our method is compared with several representative methods and the results show that the proposed method outperforms the classical methods in 66% of the classification cases on three datasets: Booter websites, 20-Newsgroups and WebKB.