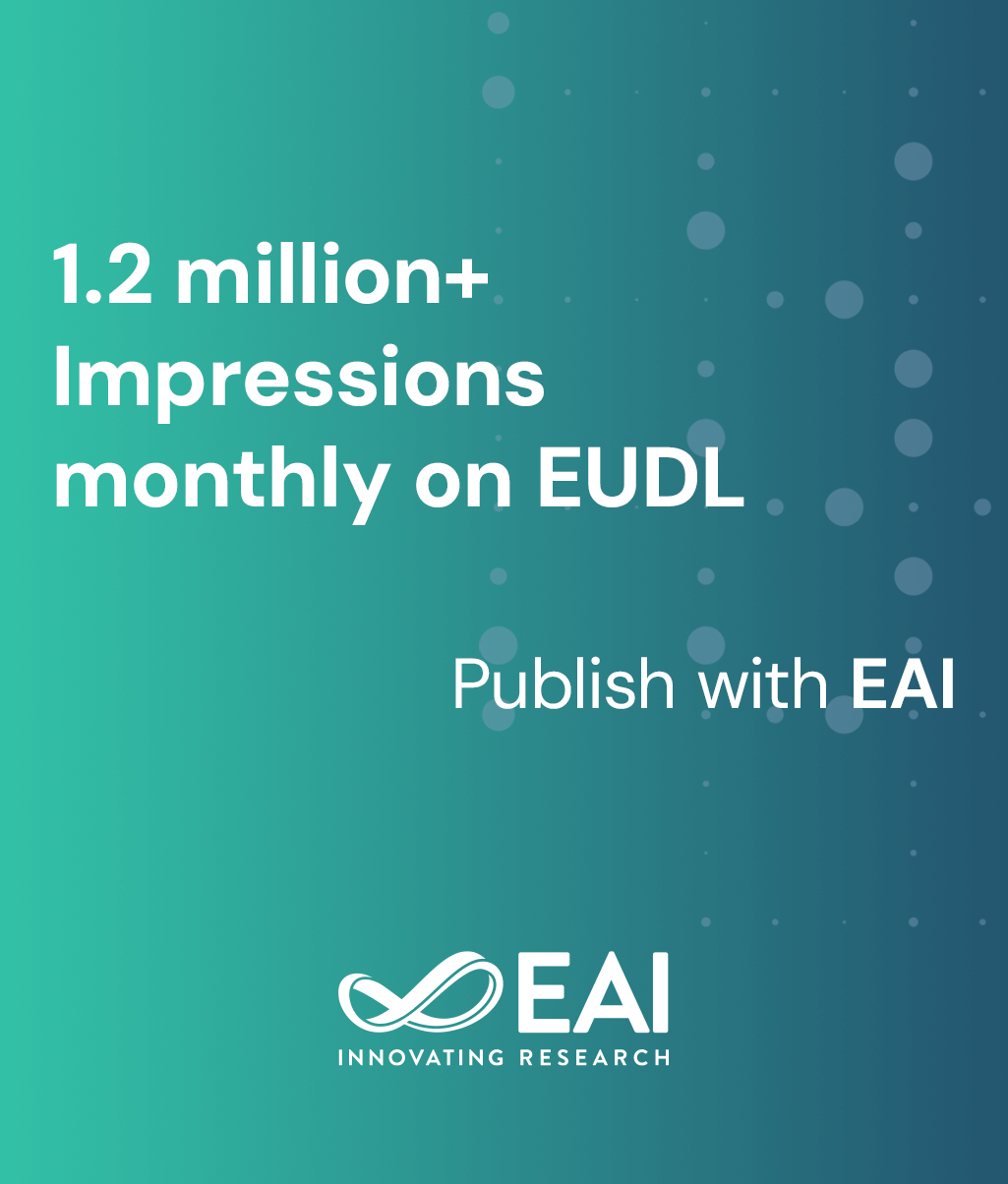
Research Article
Learning from High-Degree Entities for Knowledge Graph Modeling
@INPROCEEDINGS{10.1007/978-3-030-12981-1_35, author={Tienan Zhang and Fangfang Liu and Yan Shen and Honghao Gao and Jing Duan}, title={Learning from High-Degree Entities for Knowledge Graph Modeling}, proceedings={Collaborative Computing: Networking, Applications and Worksharing. 14th EAI International Conference, CollaborateCom 2018, Shanghai, China, December 1-3, 2018, Proceedings}, proceedings_a={COLLABORATECOM}, year={2019}, month={2}, keywords={KB completion Entity degree Indirect relation Entity prediction Relation weakening}, doi={10.1007/978-3-030-12981-1_35} }
- Tienan Zhang
Fangfang Liu
Yan Shen
Honghao Gao
Jing Duan
Year: 2019
Learning from High-Degree Entities for Knowledge Graph Modeling
COLLABORATECOM
Springer
DOI: 10.1007/978-3-030-12981-1_35
Abstract
Knowledge base (KB) completion aims to infer missing facts based on existing ones in a KB. Many approaches firstly suppose that the constituents themselves (e.g., head, tail entity and relation) of a fact meet some formulas and then minimize the loss of formula to obtain the feature vectors of entities and relations. Due to the sparsity of KB, some methods also take into consideration the indirect relations between entities. However, indirect relations further widen the differences of training times of high-degree entities (entities linking by many relations) and low-degree entities. This results in underfitting of low-degree entities. In this paper, we propose the path-based TransE with aggregation () to fine-tune the feature vector of an entity by comparing it to its related entities that linked by the same relations. In this way, low-degree entities can draw useful information from high-degree entities to directly adjust their representations. Conversely, the overfitting of high-degree entities can be relieved. Extensive experiments carried on the real world dataset show our method can define entities more accurately, and inferring is more effectively than in previous methods.