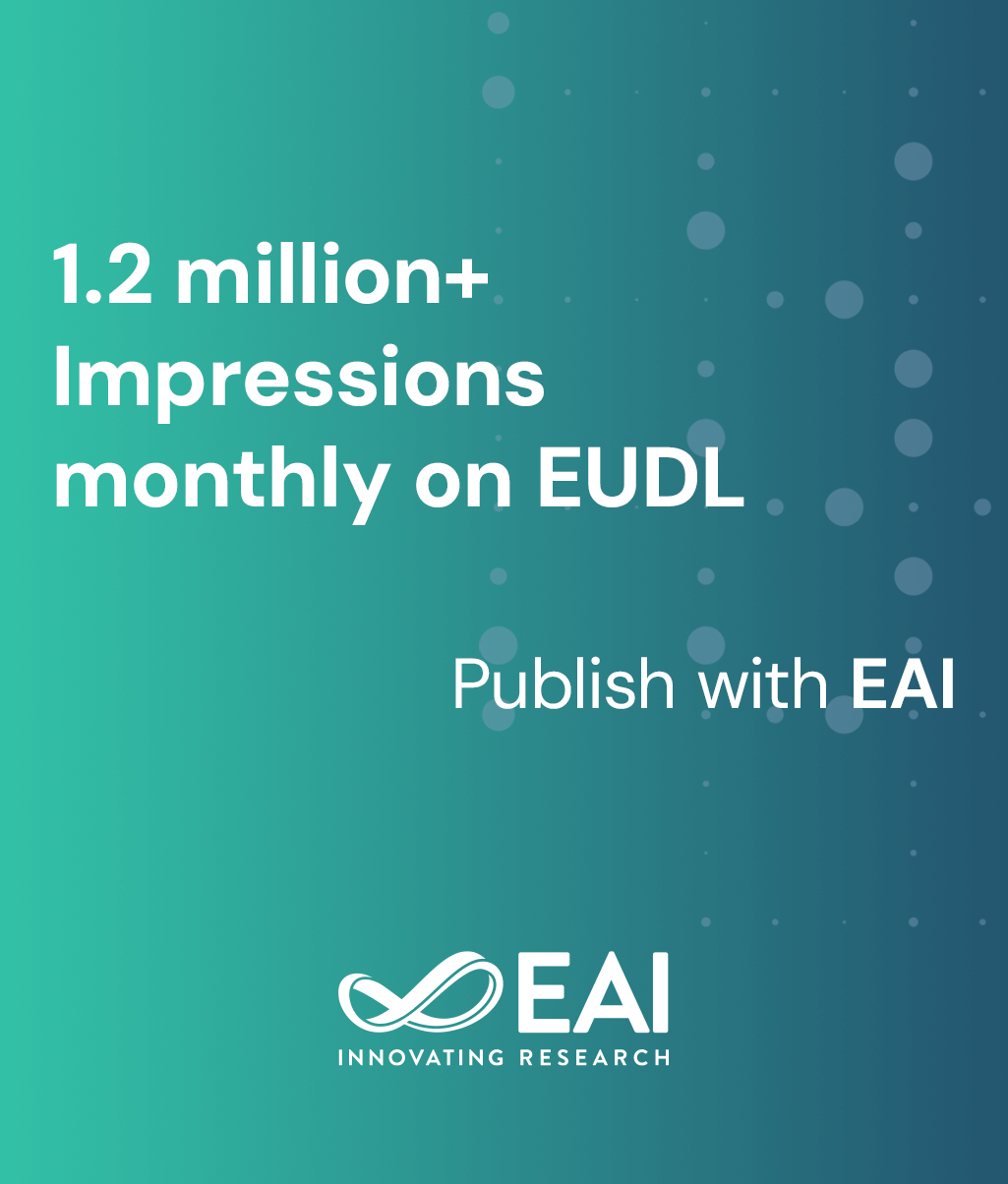
Research Article
Assessing Data Anomaly Detection Algorithms in Power Internet of Things
@INPROCEEDINGS{10.1007/978-3-030-12981-1_28, author={Zixiang Wang and Zhoubin Liu and Xiaolu Yuan and Yueshen Xu and Rui Li}, title={Assessing Data Anomaly Detection Algorithms in Power Internet of Things}, proceedings={Collaborative Computing: Networking, Applications and Worksharing. 14th EAI International Conference, CollaborateCom 2018, Shanghai, China, December 1-3, 2018, Proceedings}, proceedings_a={COLLABORATECOM}, year={2019}, month={2}, keywords={Anomaly detection Internet of Things LOF}, doi={10.1007/978-3-030-12981-1_28} }
- Zixiang Wang
Zhoubin Liu
Xiaolu Yuan
Yueshen Xu
Rui Li
Year: 2019
Assessing Data Anomaly Detection Algorithms in Power Internet of Things
COLLABORATECOM
Springer
DOI: 10.1007/978-3-030-12981-1_28
Abstract
At present, the data related to the Internet of Things has shown explosive growth, and the importance of data has been greatly improved. Data collection and analysis are becoming more and more valuable. However, a large number of abnormal data will bring great trouble to our research, and even lead people into misunderstandings. Therefore, anomaly detection is particularly necessary and important. The purpose of this paper is to find an efficient and accurate outlier detection algorithm. Our work also analyzes their advantages and disadvantages theoretically. At the same time, the effects of the data set size, number of proximity points, and data dimension on CPU time and precision are discussed. The performance, advantages and disadvantages of each algorithm in dealing with high-dimensional data are compared and analyzed. Finally, the algorithm is used to analyze the actual anomaly data collected from the Internet of Things and analyze the results. The results show that the LOF algorithm can find the abnormal data in the data set in a shorter time and with higher accuracy.