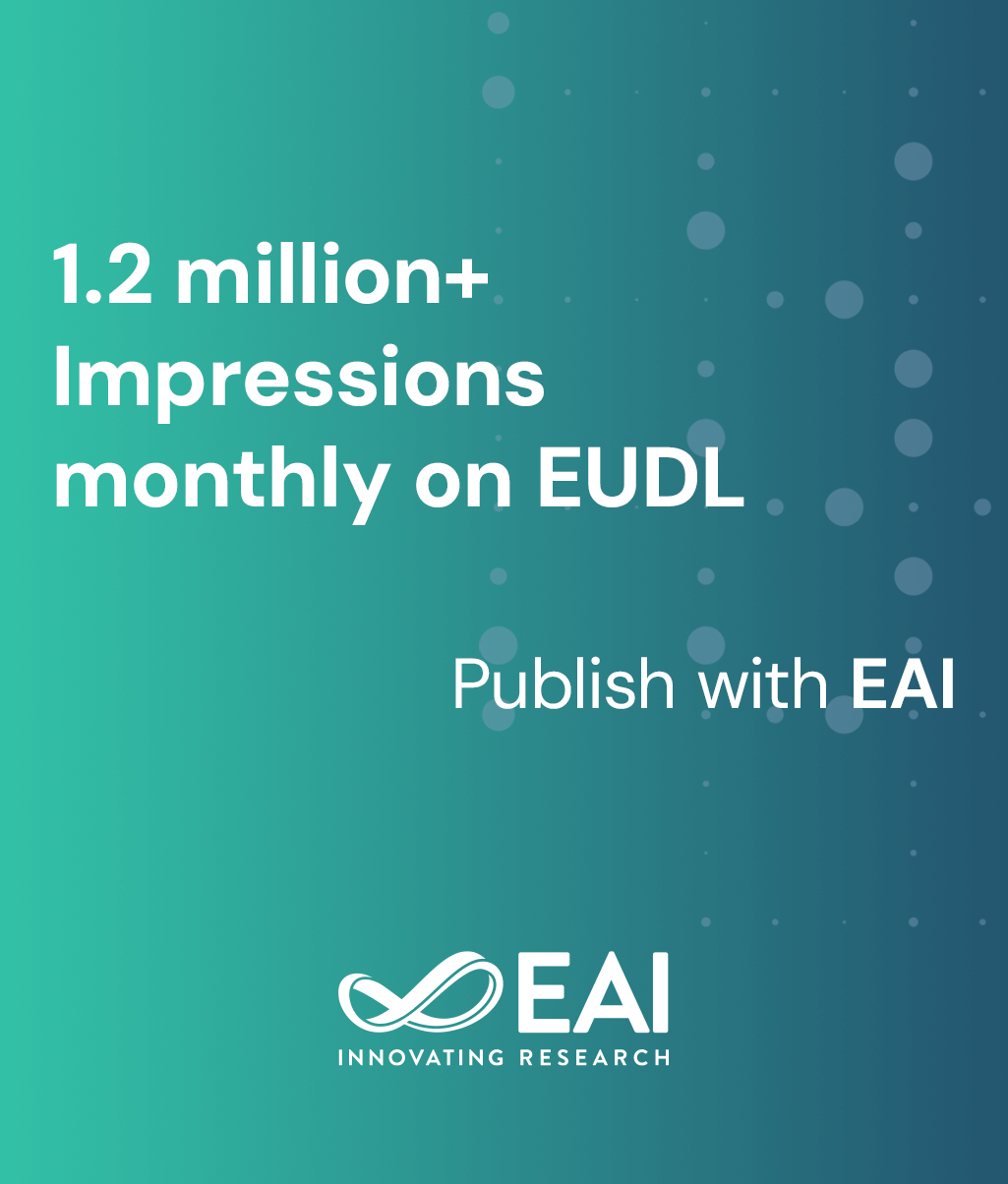
Research Article
Predicting Duration of Traffic Accidents Based on Ensemble Learning
@INPROCEEDINGS{10.1007/978-3-030-12981-1_18, author={Lina Shan and Zikun Yang and Huan Zhang and Ruyi Shi and Li Kuang}, title={Predicting Duration of Traffic Accidents Based on Ensemble Learning}, proceedings={Collaborative Computing: Networking, Applications and Worksharing. 14th EAI International Conference, CollaborateCom 2018, Shanghai, China, December 1-3, 2018, Proceedings}, proceedings_a={COLLABORATECOM}, year={2019}, month={2}, keywords={The duration of traffic accidents XGBoost Artificial neural networks}, doi={10.1007/978-3-030-12981-1_18} }
- Lina Shan
Zikun Yang
Huan Zhang
Ruyi Shi
Li Kuang
Year: 2019
Predicting Duration of Traffic Accidents Based on Ensemble Learning
COLLABORATECOM
Springer
DOI: 10.1007/978-3-030-12981-1_18
Abstract
Traffic congestion can be divided into recurrent congestion and accidental congestion, and the latter one is usually caused by traffic accidents. It is of great significance to predict the duration of traffic accidents accurately and transfer the results to drivers on the road in time. Most of the existing works utilize traditional, single machine learning model to predict the duration of accident, while the accuracy is not satisfying. In this paper, we firstly construct and extract features from the accident records including description, location, as well as some external information such as weather. We then divide the duration into multiple periods, corresponding to multiple categories. In order to improve the prediction precision of rare categories, we convert the multi-class classification problem into a binary classification problem, constructing multiple XGBoost binary classifiers which are restricted by F1 (harmonic mean) evaluation index. Finally, in order to improve the overall accuracy further, the classification results are integrated by using artificial neural networks. The experiment is conducted on real datasets in Xiamen and employs mean absolute percentage error (MAPE) and root-mean-square error (RMSE) as indicators. The experimental results show the effectiveness of the proposed method and show better performance in comparison with traditional models.