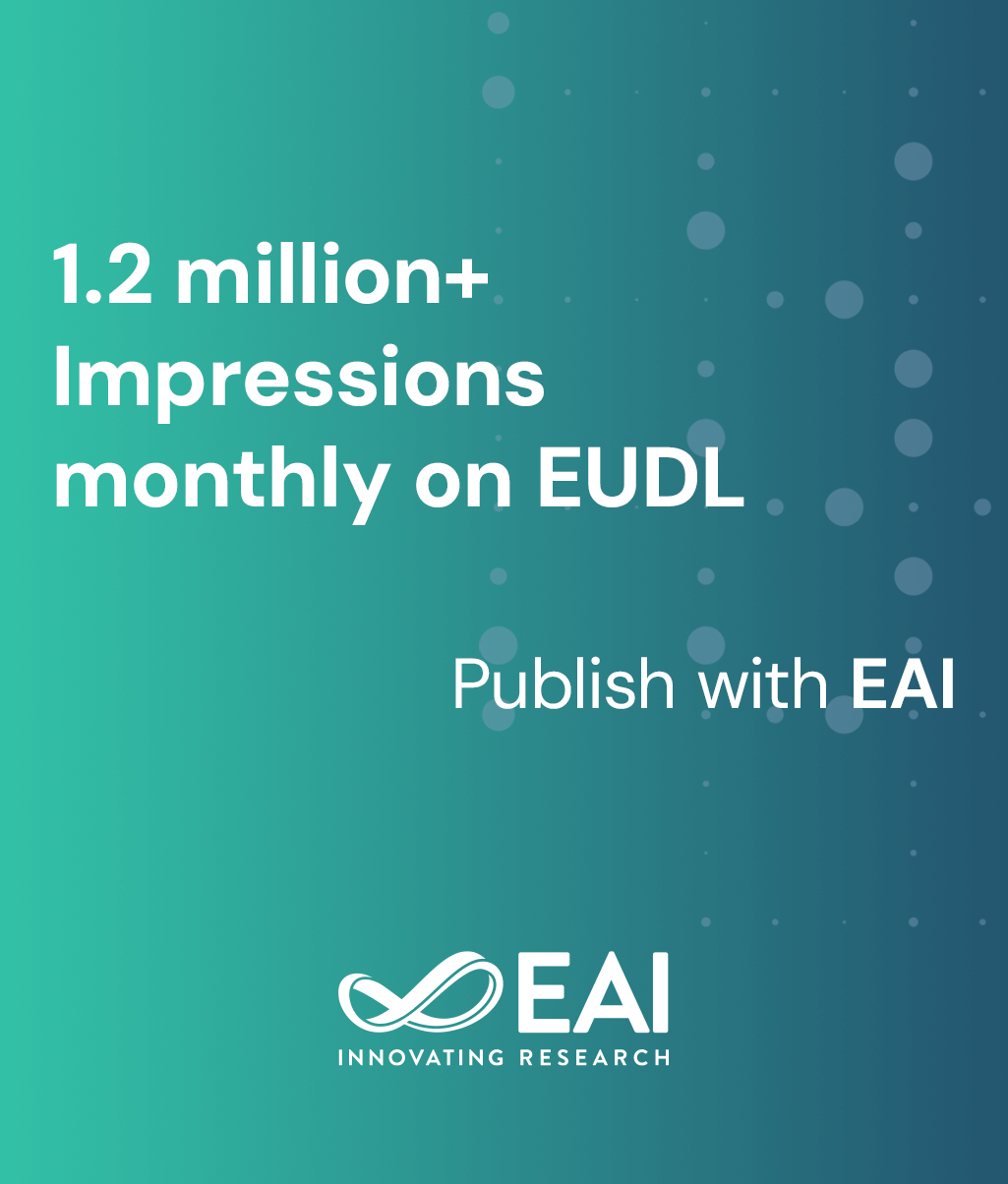
Research Article
A Machine Learning Based Temporary Base Station (BS) Placement Scheme in Booming Customers Circumstance
@INPROCEEDINGS{10.1007/978-3-030-06161-6_55, author={Qinglong Dai and Li Zhu and Peng Wang and Guodong Li and Jianjun Chen}, title={A Machine Learning Based Temporary Base Station (BS) Placement Scheme in Booming Customers Circumstance}, proceedings={Communications and Networking. 13th EAI International Conference, ChinaCom 2018, Chengdu, China, October 23-25, 2018, Proceedings}, proceedings_a={CHINACOM}, year={2019}, month={1}, keywords={Network planning Machine learning Big data K-means algorithm}, doi={10.1007/978-3-030-06161-6_55} }
- Qinglong Dai
Li Zhu
Peng Wang
Guodong Li
Jianjun Chen
Year: 2019
A Machine Learning Based Temporary Base Station (BS) Placement Scheme in Booming Customers Circumstance
CHINACOM
Springer
DOI: 10.1007/978-3-030-06161-6_55
Abstract
Explosive increase of terminal users and the amount of data traffic give a great challenge for Internet service providers (ISPs). At the same time, this big data also brings an opportunity for ISPs. How to solve network planning problem in emergency or clogging situation, based on big data? In this paper, we try to realize effective and flexible temporary base station (BS) placement through machine learning in a booming customers situation, with ISPs’ massive data. A machine learning based temporary BS placement scheme is presented. A K-means based model training algorithm is put forward, as a vital part of machine learning based temporary BS placement scheme. K-means algorithm is selected as a representative example of machine learning algorithm. The performances of BS position with random starting point, BS position iteration, average path length with different parameters, are conducted to prove the availability of our work.