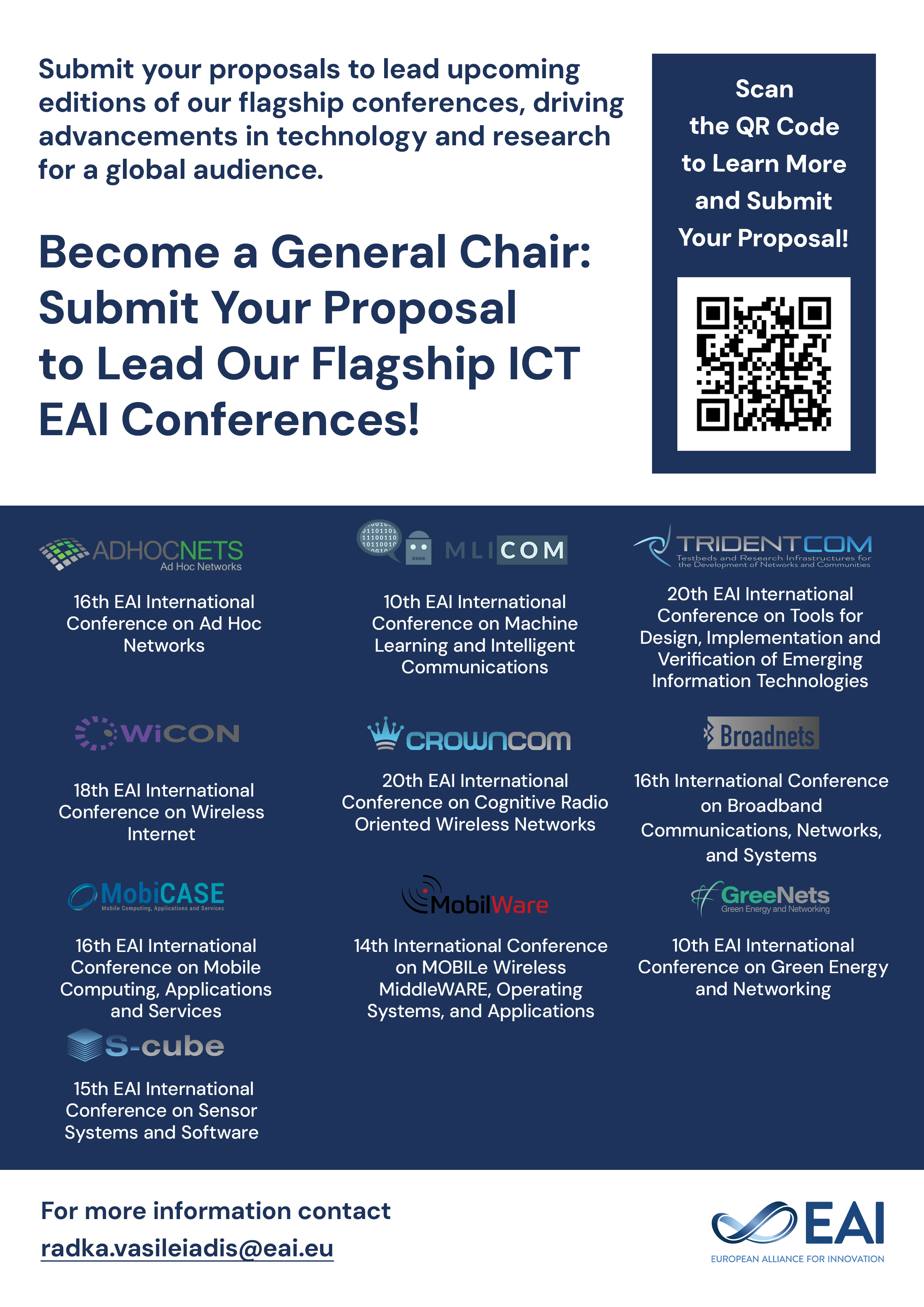
Research Article
Aggregating Multidimensional Wireless Link Information for Device-Free Localization
@INPROCEEDINGS{10.1007/978-3-030-06161-6_4, author={Dongping Yu and Yan Guo and Ning Li and Sixing Yang}, title={Aggregating Multidimensional Wireless Link Information for Device-Free Localization}, proceedings={Communications and Networking. 13th EAI International Conference, ChinaCom 2018, Chengdu, China, October 23-25, 2018, Proceedings}, proceedings_a={CHINACOM}, year={2019}, month={1}, keywords={Device-free localization Wireless sensor network Compressive sensing Sparse Bayesian learning}, doi={10.1007/978-3-030-06161-6_4} }
- Dongping Yu
Yan Guo
Ning Li
Sixing Yang
Year: 2019
Aggregating Multidimensional Wireless Link Information for Device-Free Localization
CHINACOM
Springer
DOI: 10.1007/978-3-030-06161-6_4
Abstract
Device-free localization (DFL) is an emerging and promising technique, which can realize target localization without the requirement of attaching any wireless devices to targets. By analyzing the shadowing loss caused by targets on wireless links, we can estimate the target locations. However, for existing DFL approaches, a large number of wireless links is required to guarantee a certain localization precision, which may lead to high hardware cost. In this paper, we propose a novel multi-target device-free localization method with multidimensional wireless link information (MDMI). Unlike previous works that measure RSS only on a single transmission power level, MDMI collects RSS measurements from multiple transmission power levels to enrich the measurement information. Furthermore, the compressive sensing (CS) theory is applied by exploiting the inherent spatial sparsity of DFL. We model the DFL problem as a joint sparse recovery problem and adopt the multiple sparse Bayesian learning (M-SBL) algorithm to reconstruct the sparse vectors of different transmission power levels. Numerical simulation results demonstrate the outstanding performance of the proposed method.