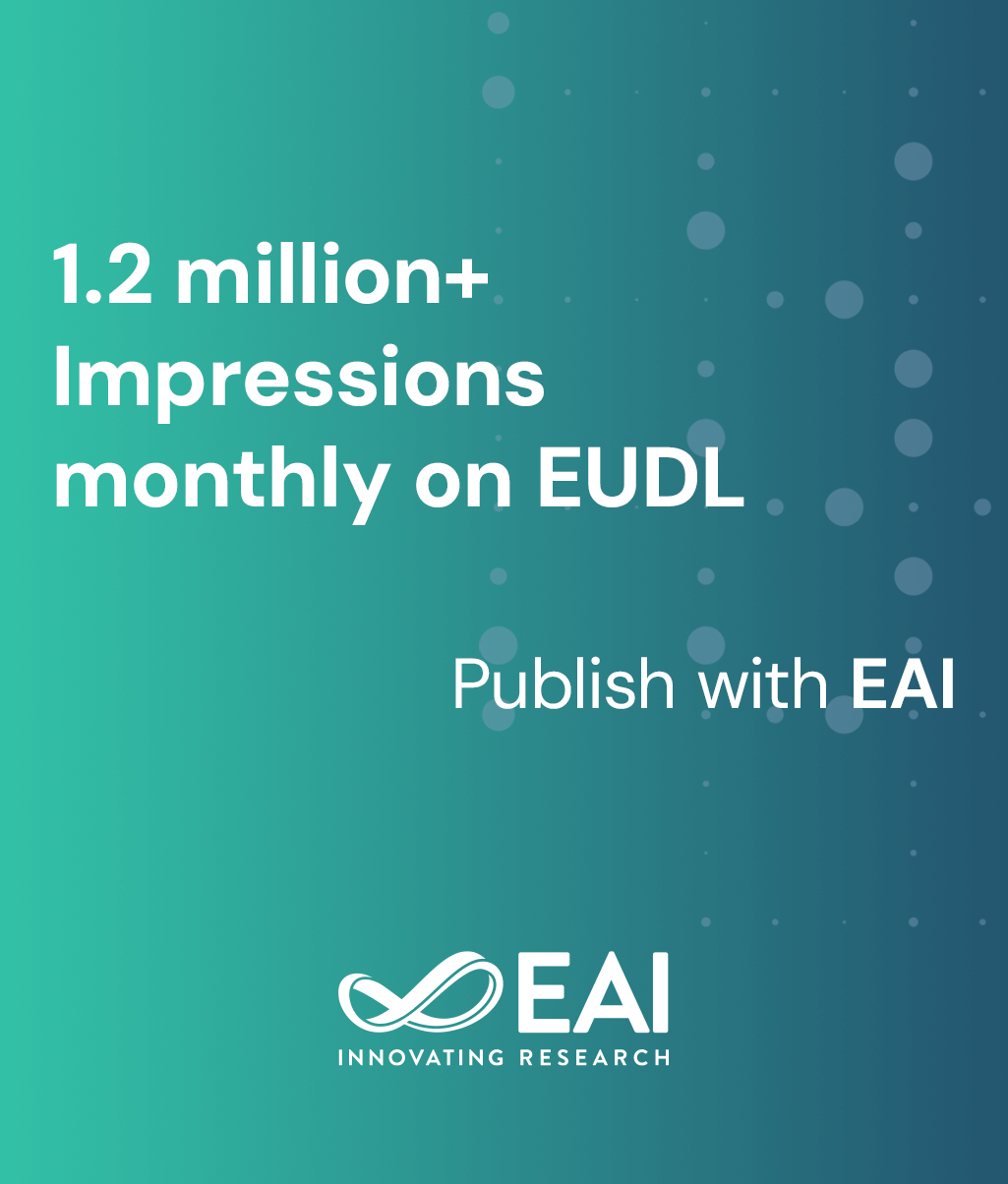
Research Article
Deep Learning for Smartphone-Based Human Activity Recognition Using Multi-sensor Fusion
@INPROCEEDINGS{10.1007/978-3-030-06158-6_7, author={Charlene San Buenaventura and Nestor Tiglao and Rowel Atienza}, title={Deep Learning for Smartphone-Based Human Activity Recognition Using Multi-sensor Fusion}, proceedings={Wireless Internet. 11th EAI International Conference, WiCON 2018, Taipei, Taiwan, October 15-16, 2018, Proceedings}, proceedings_a={WICON}, year={2019}, month={1}, keywords={Deep learning Human activity recognition Sensor fusion Hyperparameter optimization}, doi={10.1007/978-3-030-06158-6_7} }
- Charlene San Buenaventura
Nestor Tiglao
Rowel Atienza
Year: 2019
Deep Learning for Smartphone-Based Human Activity Recognition Using Multi-sensor Fusion
WICON
Springer
DOI: 10.1007/978-3-030-06158-6_7
Abstract
In the field of ubiquitous computing, machines need to be aware of the present context to enable anticipatory communication with humans. This leads to human-centric applications that have the primary objective of improving the Quality-of-Life (QoL) of its users. One important type of context information for these applications is the current activity of the user, which can be derived from environmental and wearable sensors. Due to the processing capabilities and the number of sensors embedded in a smartphone, this device exhibits the most promise among other existing technologies in human activity recognition (HAR) research. While machine learning-based solutions have been successful in past HAR studies, several design struggles can be easily resolved with deep learning. In this paper, we investigated Convolutional Neural Networks and Long Short-Term Memory Networks in dealing with common challenges in smartphone-based HAR, such as device location and subject dependency, and manual feature extraction. We showed that the CNN model accomplished location- and subject-independent recognition with overall accuracy of 98.38% and 90.61%, respectively. The LSTM model also performed location-independent recognition with an accuracy of 97.17% but has a subject-independent recognition accuracy of only 80.02%. Finally, optimal performance of the network was achieved by performing Bayesian Optimization using Gaussian Processes in tuning the design hyperparameters.