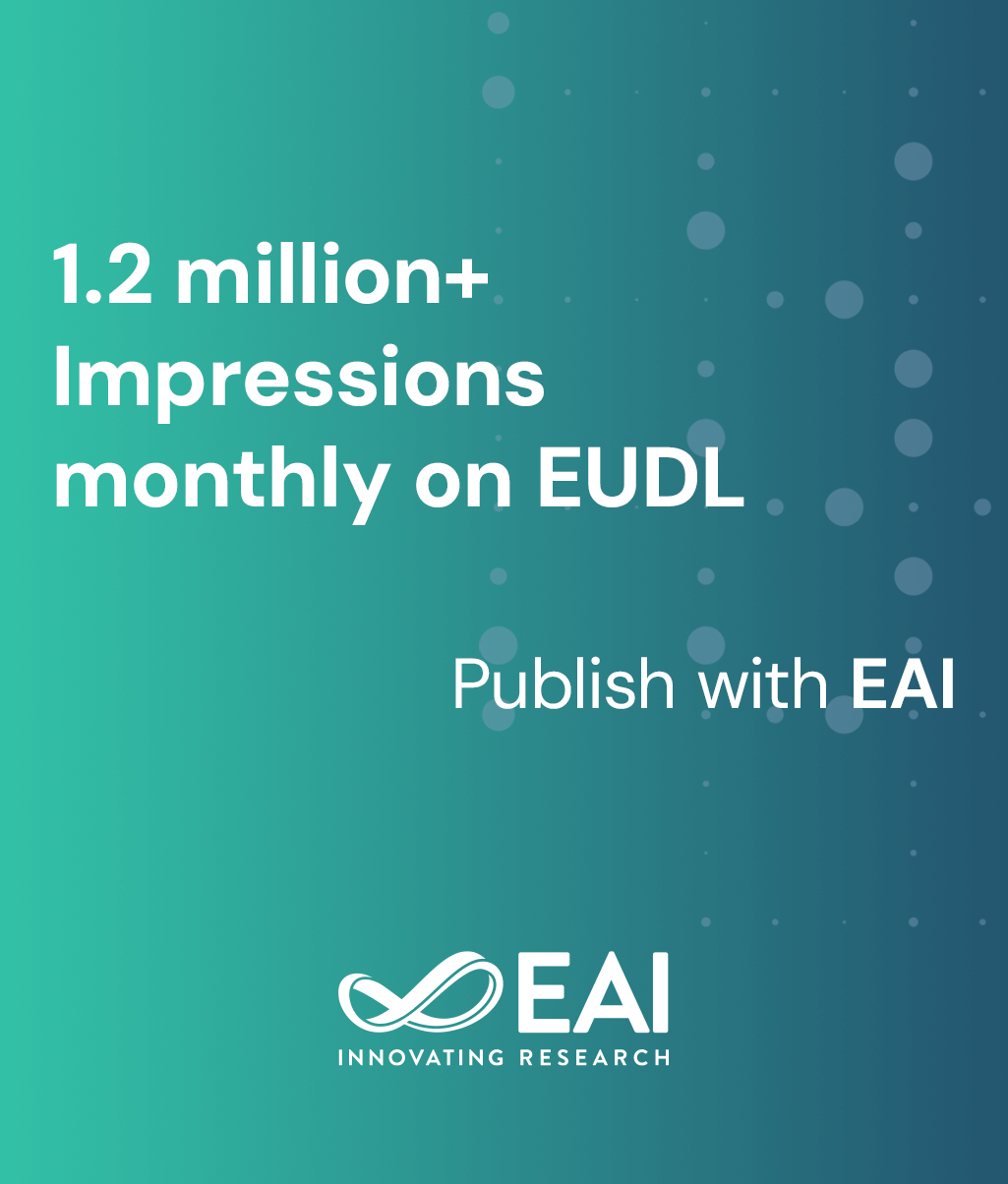
Research Article
Audio Event Detection Using Wireless Sensor Networks Based on Deep Learning
@INPROCEEDINGS{10.1007/978-3-030-06158-6_11, author={Jose Mendoza and Vanessa Tan and Vivencio Fuentes and Gabriel Perez and Nestor Tiglao}, title={Audio Event Detection Using Wireless Sensor Networks Based on Deep Learning}, proceedings={Wireless Internet. 11th EAI International Conference, WiCON 2018, Taipei, Taiwan, October 15-16, 2018, Proceedings}, proceedings_a={WICON}, year={2019}, month={1}, keywords={Audio event detection Ambient assisted living}, doi={10.1007/978-3-030-06158-6_11} }
- Jose Mendoza
Vanessa Tan
Vivencio Fuentes
Gabriel Perez
Nestor Tiglao
Year: 2019
Audio Event Detection Using Wireless Sensor Networks Based on Deep Learning
WICON
Springer
DOI: 10.1007/978-3-030-06158-6_11
Abstract
Wireless acoustic sensor network is useful for ambient assisted living applications. Its capability of incorporating an audio event detection and classification system helps its users, especially elderly, on their everyday needs. In this paper, we propose using convolutional neural networks (CNN) for classifying audio streams. In contrast to AAL systems using traditional machine learning, our solution is capable of learning and inferring activities in an end-to-end manner. To demonstrate the system, we developed a wireless sensor network composed of Raspberry Pi boards with microphones as nodes. The audio classification system results to an accuracy of using a parallel network for the Urban8k dataset, extracting constant-Q transform (CQT) features as system inputs. The overall system is scalabale and flexible in terms of the number of nodes, hence it is applicable on wide areas where assisted living applications are utilized.