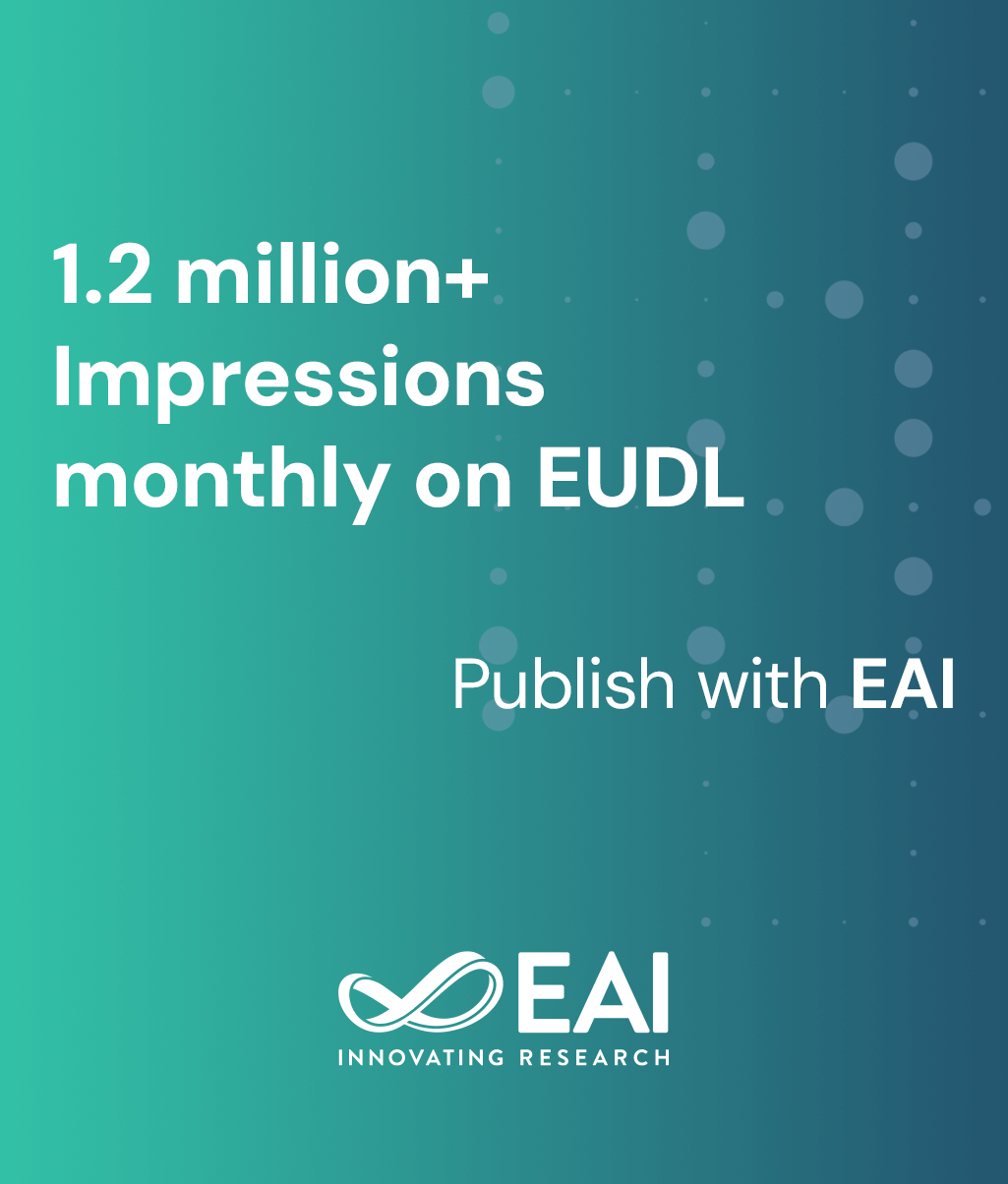
Research Article
IoT Big Data Analytics with Fog Computing for Household Energy Management in Smart Grids
@INPROCEEDINGS{10.1007/978-3-030-05928-6_2, author={Shailendra Singh and Abdulsalam Yassine}, title={IoT Big Data Analytics with Fog Computing for Household Energy Management in Smart Grids}, proceedings={Smart Grid and Internet of Things. Second EAI International Conference, SGIoT 2018, Niagara Falls, ON, Canada, July 11, 2018, Proceedings}, proceedings_a={SGIOT}, year={2019}, month={1}, keywords={Internet of Things Cloud computing Fog computing Big data analytics Energy management Smart grids}, doi={10.1007/978-3-030-05928-6_2} }
- Shailendra Singh
Abdulsalam Yassine
Year: 2019
IoT Big Data Analytics with Fog Computing for Household Energy Management in Smart Grids
SGIOT
Springer
DOI: 10.1007/978-3-030-05928-6_2
Abstract
Smart homes generate a vast amount of data measurements from smart meters and devices. These data have all the velocity and veracity characteristics to be called as Big Data. Meter data analytics holds tremendous potential for utilities to understand customers’ energy consumption patterns, and allows them to manage, plan, and optimize the operation of the power grid efficiently. In this paper, we propose a unified architecture that enables innovative operations for near real-time processing of large fine-grained energy consumption data. Specifically, we propose an Internet of Things (IoT) big data analytics system that makes use of fog computing to address the challenges of complexities and resource demands for near real-time data processing, storage, and classification analysis. The design architecture and requirements of the proposed framework are illustrated in this paper while the analytics components are validated using datasets acquired from real homes.