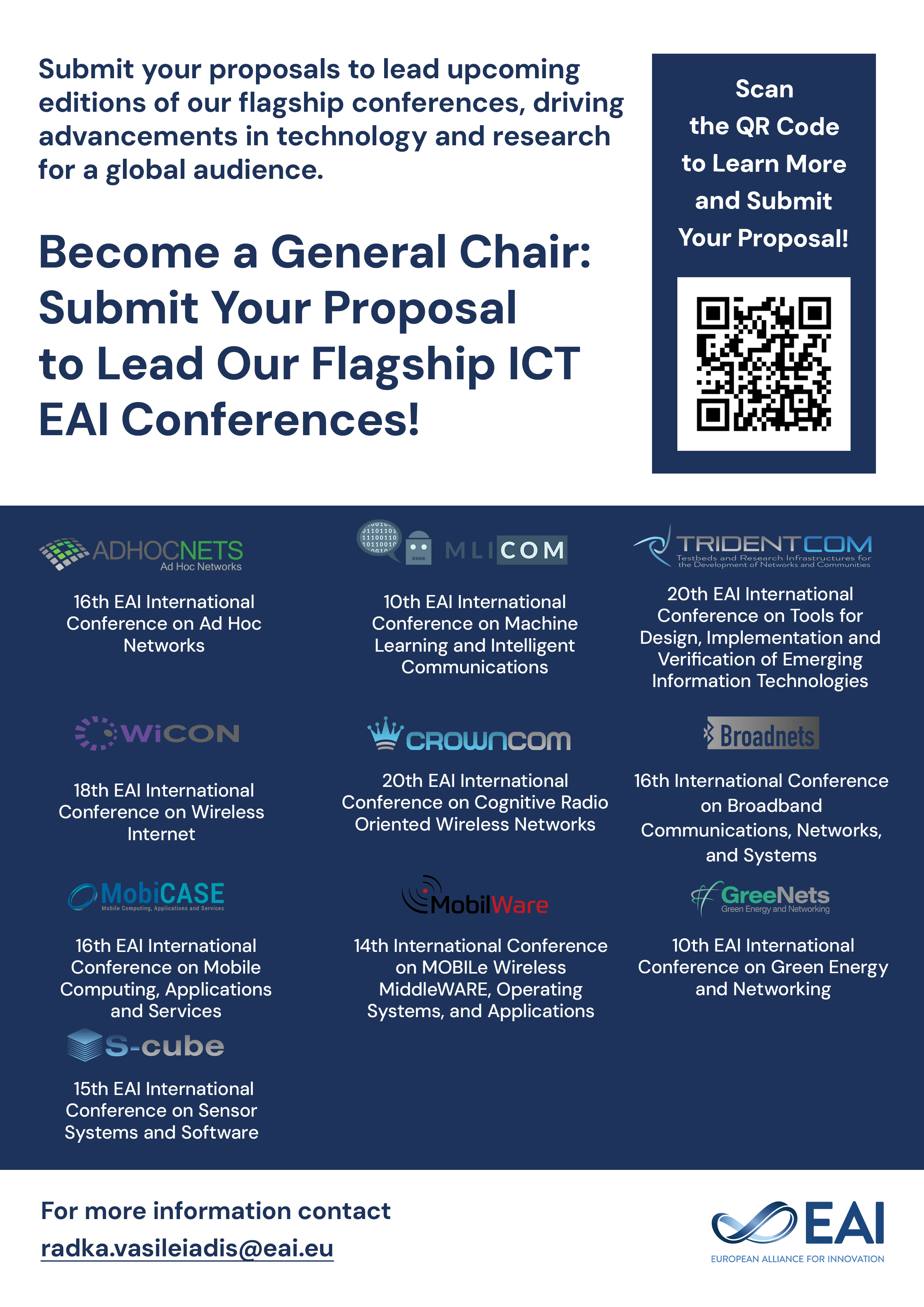
Research Article
A Decision Tree Candidate Property Selection Method Based on Improved Manifold Learning Algorithm
@INPROCEEDINGS{10.1007/978-3-030-05888-3_24, author={Fangfang Guo and Luomeng Chao and Huiqiang Wang}, title={A Decision Tree Candidate Property Selection Method Based on Improved Manifold Learning Algorithm}, proceedings={Ad Hoc Networks. 10th EAI International Conference, ADHOCNETS 2018, Cairns, Australia, September 20-23, 2018, Proceedings}, proceedings_a={ADHOCNETS}, year={2018}, month={12}, keywords={Network security Decision tree Manifold learning algorithm}, doi={10.1007/978-3-030-05888-3_24} }
- Fangfang Guo
Luomeng Chao
Huiqiang Wang
Year: 2018
A Decision Tree Candidate Property Selection Method Based on Improved Manifold Learning Algorithm
ADHOCNETS
Springer
DOI: 10.1007/978-3-030-05888-3_24
Abstract
When the traditional decision tree algorithm is applied to the field of network security analysis, due to the unreasonable property selection method, the overfitting problem may be caused, and the accuracy of the constructed decision tree is low. Therefore, this paper proposes a decision tree selection method based on improved manifold learning algorithm. The manifold learning algorithm maps the high-dimensional feature space to the low-dimensional space, so the algorithm can acquire the essential attributes of the data source. According to this, the problems of low accuracy and overfitting can be solved. Aiming at the traditional manifold learning algorithms are sensitive to noise and the algorithms converges slowly, this paper proposes a Global and Local Mapping manifold learning algorithm, and this method is used to construct a decision tree. The experimental results show that compared with the traditional ID3 decision tree construction algorithm, the improved method reduces 2.16% and 1.626% in false positive rate and false negative rate respectively.