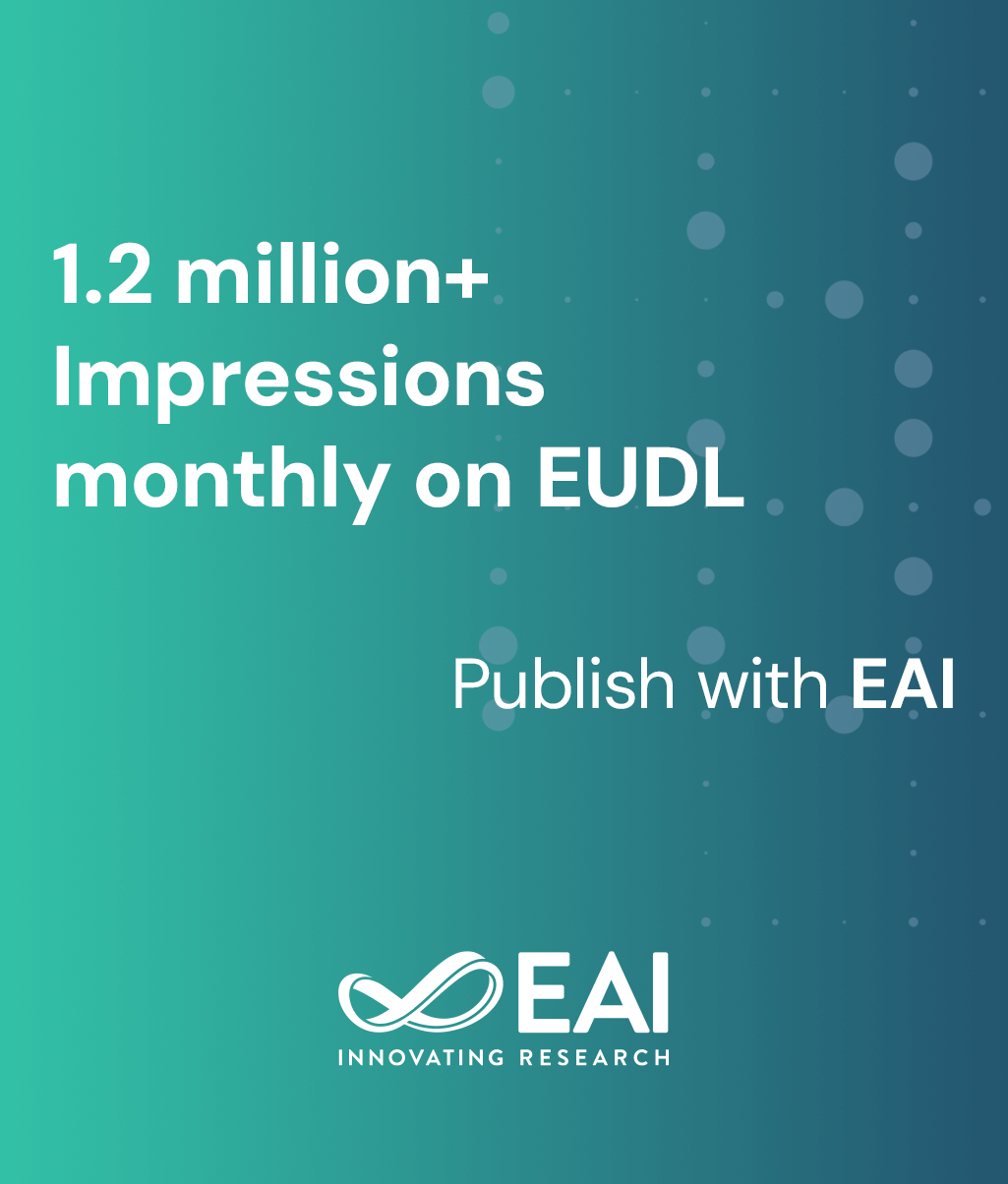
Research Article
A Predictive Model for Automatic Generation Control in Smart Grids Using Artificial Neural Networks
@INPROCEEDINGS{10.1007/978-3-030-05198-3_5, author={Chika Yinka-Banjo and Ogban-Asuquo Ugot}, title={A Predictive Model for Automatic Generation Control in Smart Grids Using Artificial Neural Networks}, proceedings={Emerging Technologies for Developing Countries. Second EAI International Conference, AFRICATEK 2018, Cotonou, Benin, May 29--30, 2018, Proceedings}, proceedings_a={AFRICATEK}, year={2018}, month={12}, keywords={Smart grid Artificial intelligence Artificial neural networks Deep learning}, doi={10.1007/978-3-030-05198-3_5} }
- Chika Yinka-Banjo
Ogban-Asuquo Ugot
Year: 2018
A Predictive Model for Automatic Generation Control in Smart Grids Using Artificial Neural Networks
AFRICATEK
Springer
DOI: 10.1007/978-3-030-05198-3_5
Abstract
This paper presents a predictive model that estimates the load for an Automatic Generation Control (AGC) system. We start by laying the foundation for our system by discussing the AGC, and the benefits of embedding it in a smart power grid. The AGC as a system is discussed with a keen focus on the mathematical relationship between the load on the system and the frequency deviation. Our predictive model is a deep neural network trained on a multivariate time series dataset for energy consumption collected over 47 months. The results show that it is possible to predict to a high accuracy, the total load on the power system within the next minute. The goal of the predictive model is predicated upon the notion that the ability to forecast the future load on the system results in the ability to estimate the frequency deviation as well, and thus giving the AGC the ability to forecast risks such as a system overload.