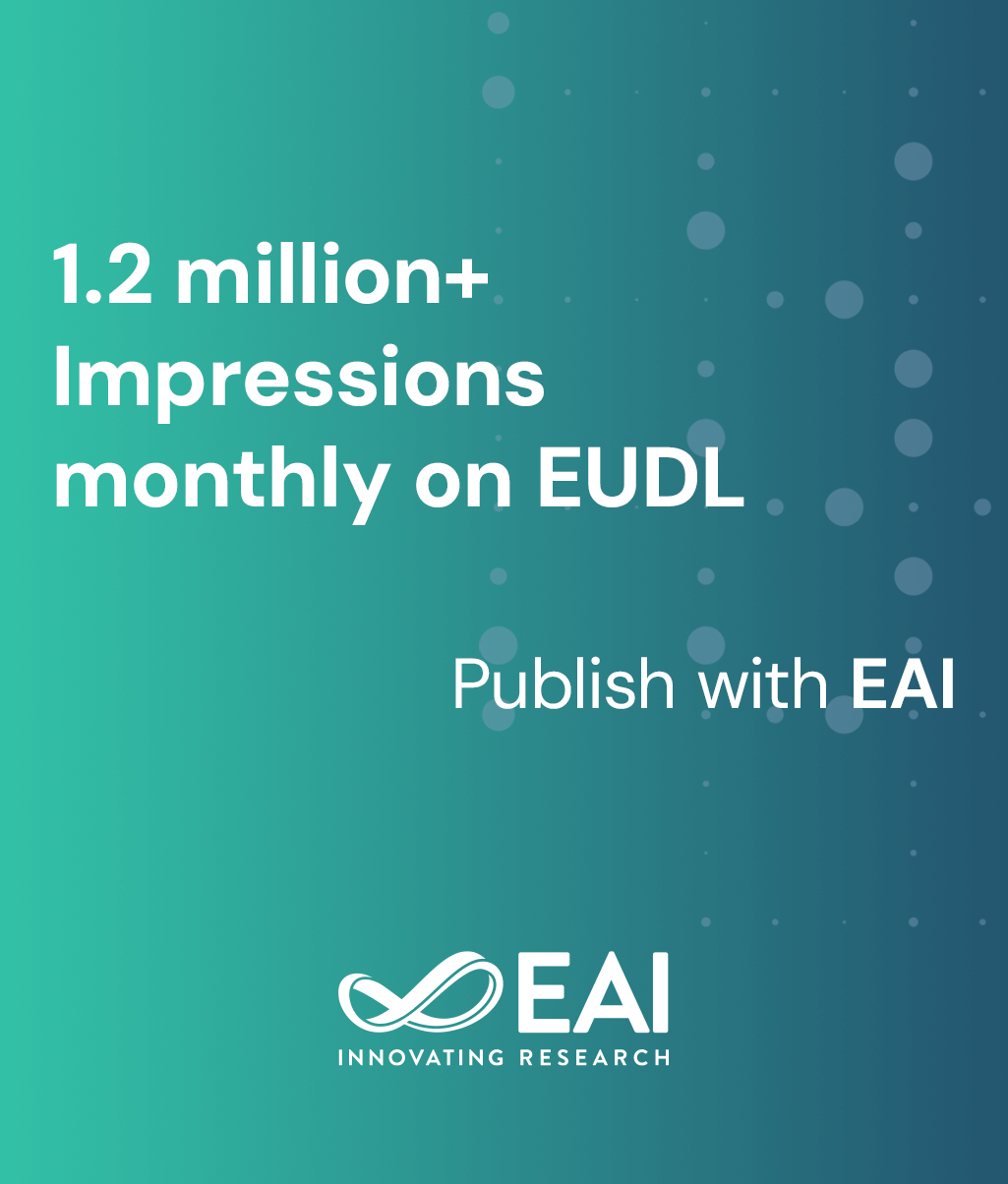
Research Article
An Electricity Power Collection Data Oriented Missing Data Imputation Solution
@INPROCEEDINGS{10.1007/978-3-030-00916-8_23, author={Jiangqi Chen and Han Li and Ting Zhao and He Liu}, title={An Electricity Power Collection Data Oriented Missing Data Imputation Solution}, proceedings={Collaborative Computing: Networking, Applications and Worksharing. 13th International Conference, CollaborateCom 2017, Edinburgh, UK, December 11--13, 2017, Proceedings}, proceedings_a={COLLABORATECOM}, year={2018}, month={10}, keywords={Missing data imputation Electricity power collection data Big data KNN Data quality}, doi={10.1007/978-3-030-00916-8_23} }
- Jiangqi Chen
Han Li
Ting Zhao
He Liu
Year: 2018
An Electricity Power Collection Data Oriented Missing Data Imputation Solution
COLLABORATECOM
Springer
DOI: 10.1007/978-3-030-00916-8_23
Abstract
In Smart Grid, the incompleteness of electricity power collection data is gradually prominent. Thus, this paper presents an electricity power collection big data oriented missing data imputation solution, which comprises a big data processing framework and a missing data imputation method for power consumption data. Based on big data techniques, the given framework supports the large-scale electricity power collection data acquisition, storage and processing. To get a better result, the proposed method takes advantage of the correlation between the loss rate of power and the user power consumption. The feasibility and the effectiveness of the proposed method is evaluated. Experimental results show the proposed method is able to convert incomplete data set into complete data set, and has good imputation stability. Compared with the KNN algorithm, the proposed method has a lower imputation error, and is a positive attempt to combine domain-specific algorithms with traditional algorithms.