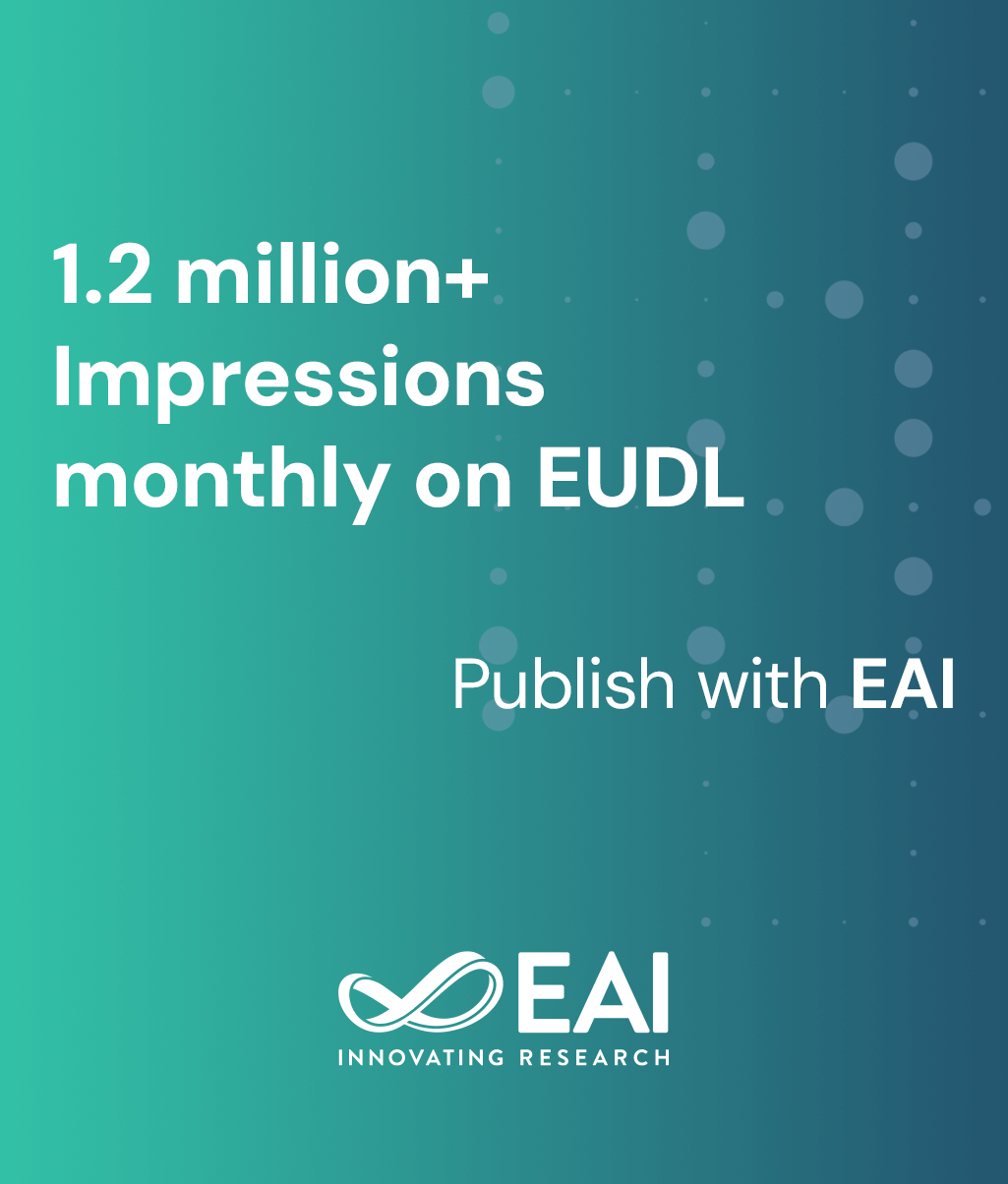
Research Article
RETaIL: A Machine Learning-Based Item-Level Localization System in Retail Environment
@INPROCEEDINGS{10.1007/978-3-030-00916-8_21, author={Xiaoyi Xu and Xiaoming Chen and Jiang Ji and Feng Chen and Addicam Sanjay}, title={RETaIL: A Machine Learning-Based Item-Level Localization System in Retail Environment}, proceedings={Collaborative Computing: Networking, Applications and Worksharing. 13th International Conference, CollaborateCom 2017, Edinburgh, UK, December 11--13, 2017, Proceedings}, proceedings_a={COLLABORATECOM}, year={2018}, month={10}, keywords={Passive RFID Machine learning Item-level localization Retail environment}, doi={10.1007/978-3-030-00916-8_21} }
- Xiaoyi Xu
Xiaoming Chen
Jiang Ji
Feng Chen
Addicam Sanjay
Year: 2018
RETaIL: A Machine Learning-Based Item-Level Localization System in Retail Environment
COLLABORATECOM
Springer
DOI: 10.1007/978-3-030-00916-8_21
Abstract
Radio-frequency identification (RFID) technology has become the key focus of indoor localization recently. The low cost and flexibility allow numbers of passive RFID-based algorithms been proposed for indoor localization. However, in a real-world environment including retail store and supermarket with large-scale item-level deployment of RFID tags and complex surroundings, these algorithms may not be available due to the collision and interference. Existing algorithms either require extra hardware or only take a small number of tags into consideration, facing difficulty in applying to these places. In this paper, we propose a novel machine learning-based REal-Time and Item-Level (RETaIL) indoor localization system, which is designed to tolerate various interference. RETaIL incorporates three machine learning algorithm, J48, SVM and cloth grouping, for indoor localization. Validations in both complex laboratory environment and real-world Levis outlet store demonstrate the accuracy and efficiency of RETaIL and its capability of dealing with interference in retail environment.