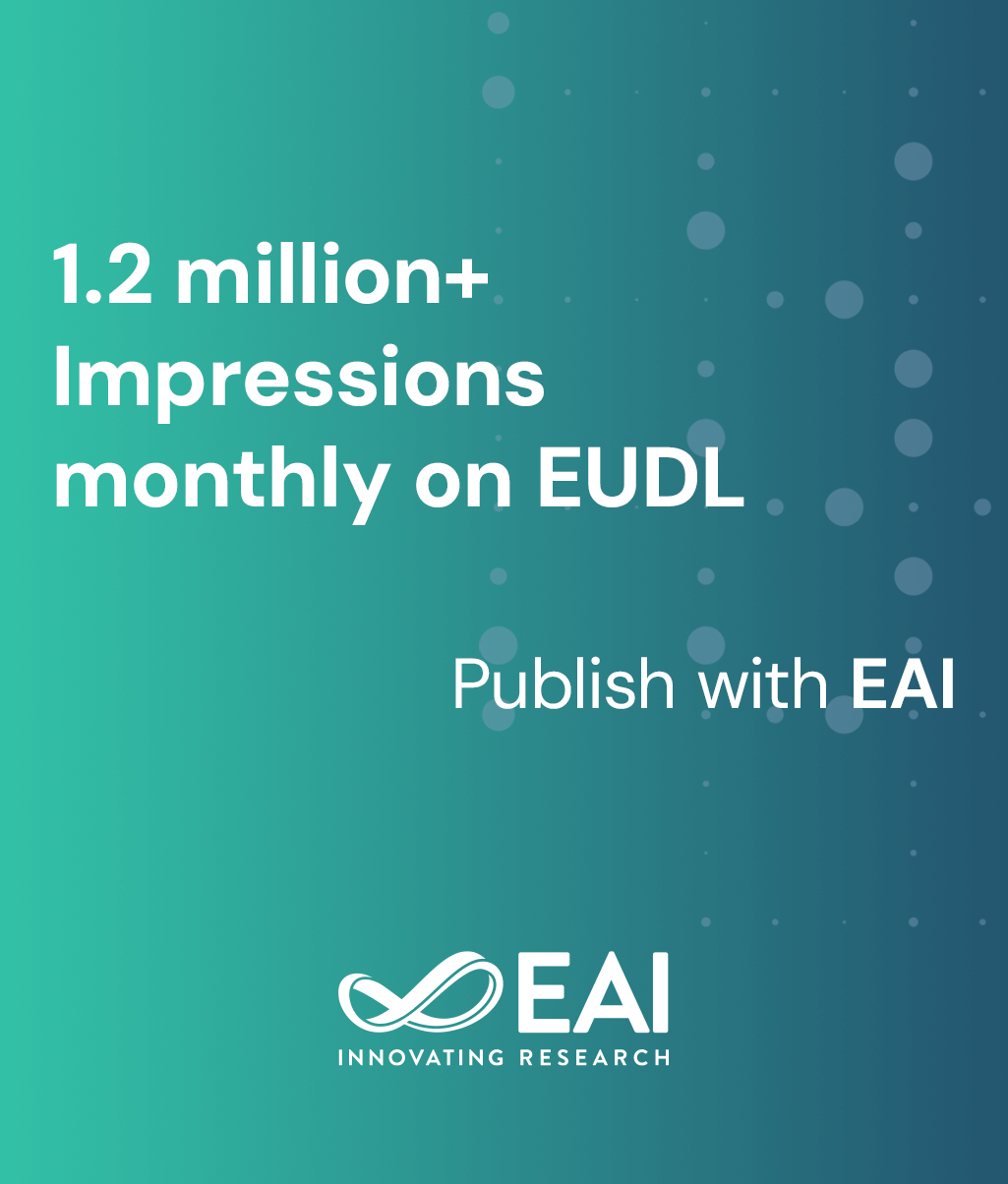
Research Article
p-Faster R-CNN Algorithm for Food Detection
@INPROCEEDINGS{10.1007/978-3-030-00916-8_13, author={Yanchen Wan and Yu Liu and Yuan Li and Puhong Zhang}, title={p-Faster R-CNN Algorithm for Food Detection}, proceedings={Collaborative Computing: Networking, Applications and Worksharing. 13th International Conference, CollaborateCom 2017, Edinburgh, UK, December 11--13, 2017, Proceedings}, proceedings_a={COLLABORATECOM}, year={2018}, month={10}, keywords={Food health Object detection Faster R-CNN Pyramid Convolutional neural network}, doi={10.1007/978-3-030-00916-8_13} }
- Yanchen Wan
Yu Liu
Yuan Li
Puhong Zhang
Year: 2018
p-Faster R-CNN Algorithm for Food Detection
COLLABORATECOM
Springer
DOI: 10.1007/978-3-030-00916-8_13
Abstract
Eating healthily helps prevent disease, and it can be achieved by identifying the kinds and ingredients of the food to determine whether the diet is healthy. In this paper, we innovatively propose p-Faster R-CNN algorithm for healthy diet detection, which is based on Faster R-CNN with Zeiler and Fergus model (ZF-net) and Caffe framework. Before the input layer, the Gauss Pyramid is applied to form a multi-resolution pyramid of images, which expands the number and the scale of the samples. In the training stage, the multi-scale Spatial Pyramid Pooling Layer is added after the convolution layer to extract multi-scale features. To evaluate the performance of p-Faster R-CNN, we compare it with Fast R-CNN and Faster R-CNN. The experiment results demonstrated that p-Faster R-CNN increases the AP value of each kind of food by more than 2% compared with Faster R-CNN, and p-Faster R-CNN, Faster R-CNN are superior to Fast R-CNN in accuracy and speed. At last, the total dataset we established is used to construct the application of judging the healthy diet by uploading intake photos.