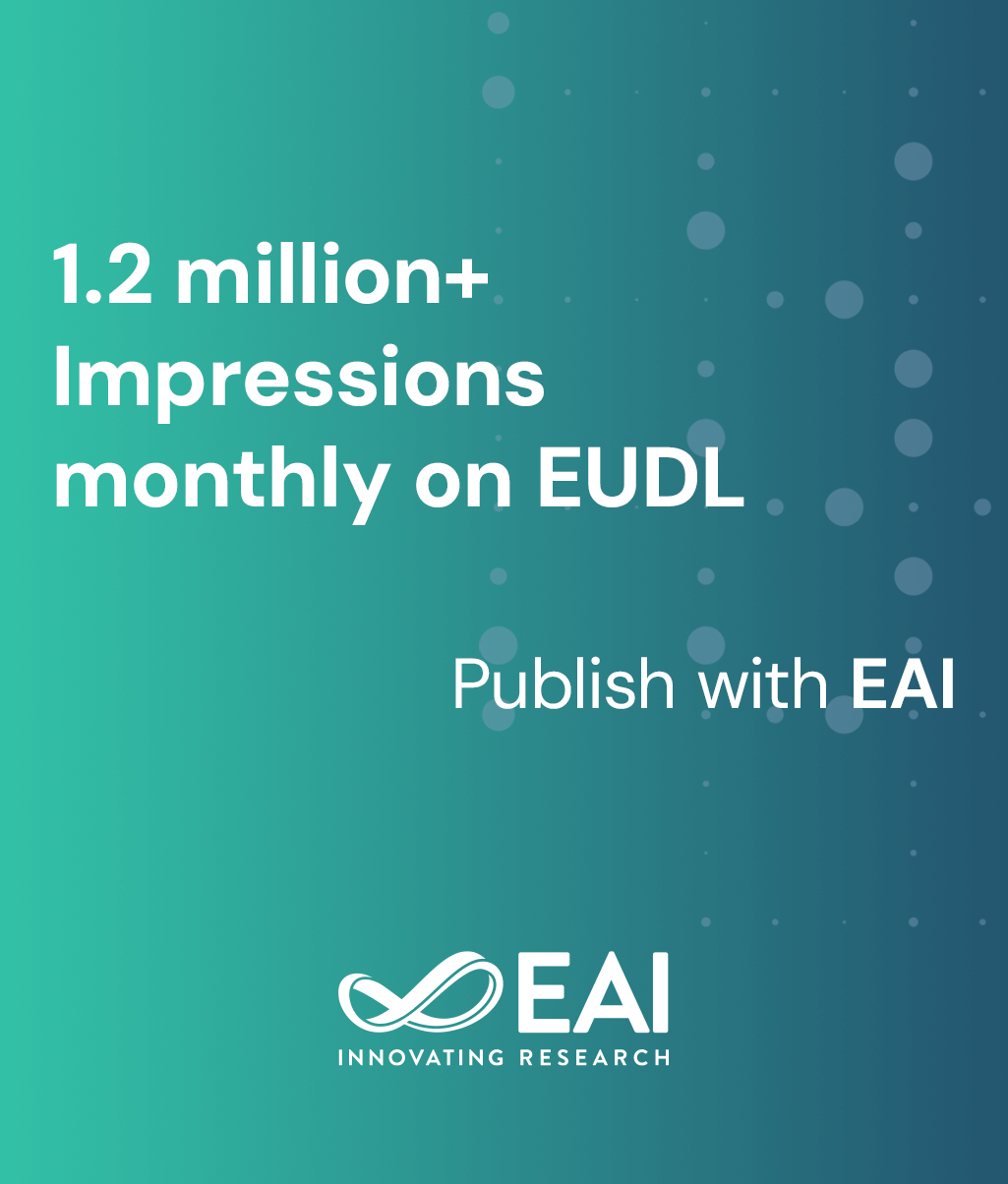
Research Article
MOPSO Optimized Radar CBMeMBer Forward-Backward Smoothing Filter
@INPROCEEDINGS{10.1007/978-3-030-00557-3_60, author={Jiazheng Pei and Yong Huang and Yunlong Dong and Xiaolong Chen}, title={MOPSO Optimized Radar CBMeMBer Forward-Backward Smoothing Filter}, proceedings={Machine Learning and Intelligent Communications. Third International Conference, MLICOM 2018, Hangzhou, China, July 6-8, 2018, Proceedings}, proceedings_a={MLICOM}, year={2018}, month={10}, keywords={Particle swarm optimization Particle filter CBMeMBer filter Forward-backward smoothing}, doi={10.1007/978-3-030-00557-3_60} }
- Jiazheng Pei
Yong Huang
Yunlong Dong
Xiaolong Chen
Year: 2018
MOPSO Optimized Radar CBMeMBer Forward-Backward Smoothing Filter
MLICOM
Springer
DOI: 10.1007/978-3-030-00557-3_60
Abstract
For the tracking of multiple maneuvering targets under radar observations, the Cardinality-Balanced Multi-Bernoulli based Sequential Monte-Carlo Filter (SMC-CBMeMBer) tracking algorithm gets its shortcomings that the estimation of number is inaccurate and the state estimation accuracy is degraded. This paper presents an improved tracking algorithm based on SMC-CBMeMBer smoothing filter. In the prediction process, the algorithm uses Multi-objectIve Particle Swarm Optimization (MOPSO), combined with the measured values at the current moment, to move the particles to the location where the posterior probability density distribution takes a larger value; Besides the smooth recursive method is used to smooth the filter value with multi-target measurement data, and the estimation accuracy of the algorithm is improved on the basis of sacrificing certain operation efficiency. The simulation results show that compared with the traditional filter and smoothing methods, the proposed algorithm performs better in terms of the accuracy of the estimation of the number of maneuvering targets and the accuracy of the target state estimation.