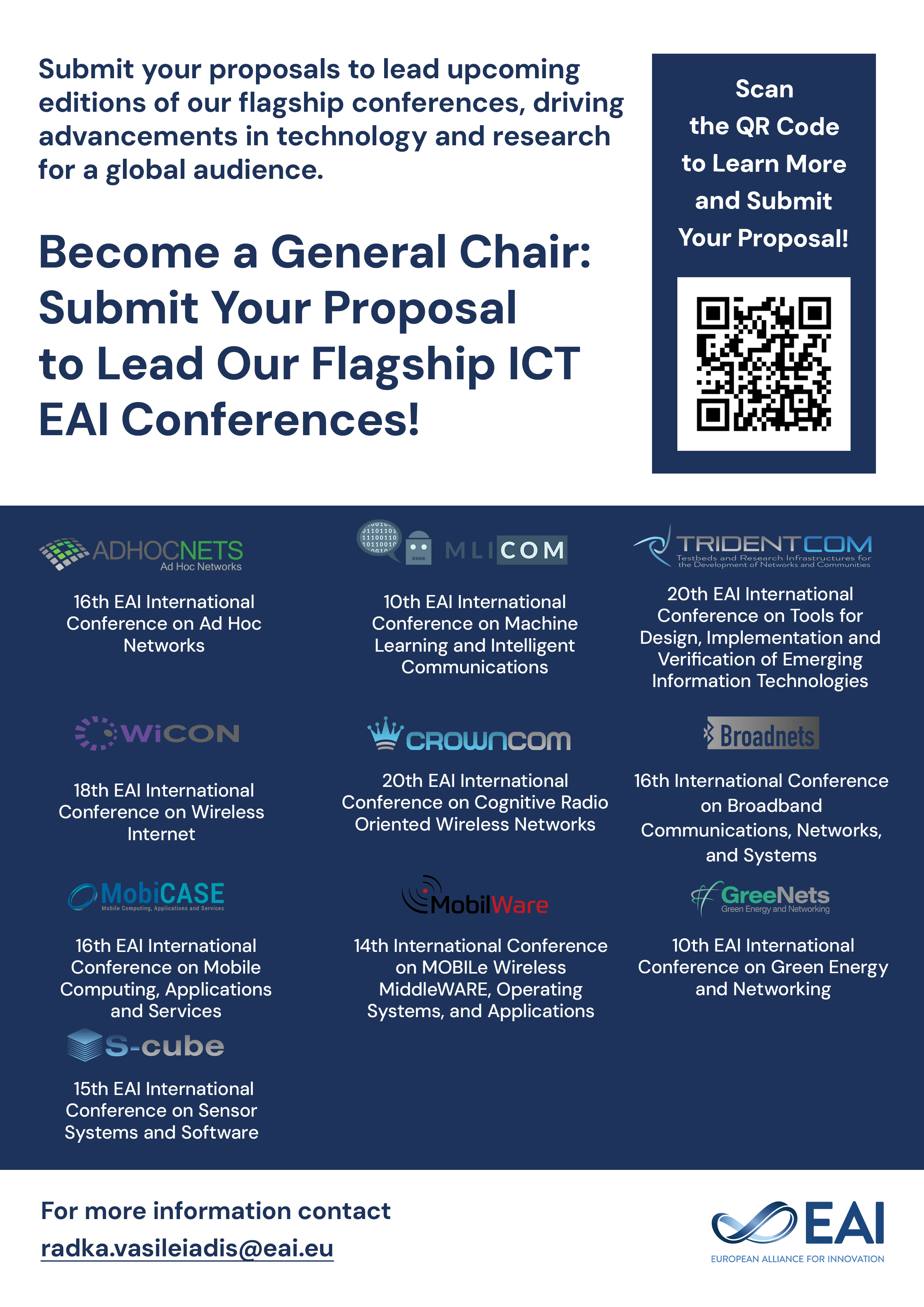
Research Article
Detecting Digital Photo Tampering with Deep Learning
@INPROCEEDINGS{10.1007/978-3-030-00557-3_47, author={Mitchell Singleton and Qingzhong Liu}, title={Detecting Digital Photo Tampering with Deep Learning}, proceedings={Machine Learning and Intelligent Communications. Third International Conference, MLICOM 2018, Hangzhou, China, July 6-8, 2018, Proceedings}, proceedings_a={MLICOM}, year={2018}, month={10}, keywords={Photography Detection of tampering Detection of modification Detection of falsification Deep learning}, doi={10.1007/978-3-030-00557-3_47} }
- Mitchell Singleton
Qingzhong Liu
Year: 2018
Detecting Digital Photo Tampering with Deep Learning
MLICOM
Springer
DOI: 10.1007/978-3-030-00557-3_47
Abstract
The tools to tamper with digital photographs have become easier to use by the lay person and techniques have evolved that make detection of tampering more difficult, there is a clear need to not only discover novel ways to distinguish the difference between real and fake, but also to make the detection quicker and easier. Using content-aware resizing, which is also known as image retargeting, seam carving, content-aware rescaling, liquid rescaling, or liquid resizing, allows for changing the resolution of an image while keeping the content unchanged in the image. In this paper, the retraining of Inception ResNet v2, one of the best object detection deep learning classifiers, is undertaken to look at classifications of untampered versus tampered photos via seam removal.