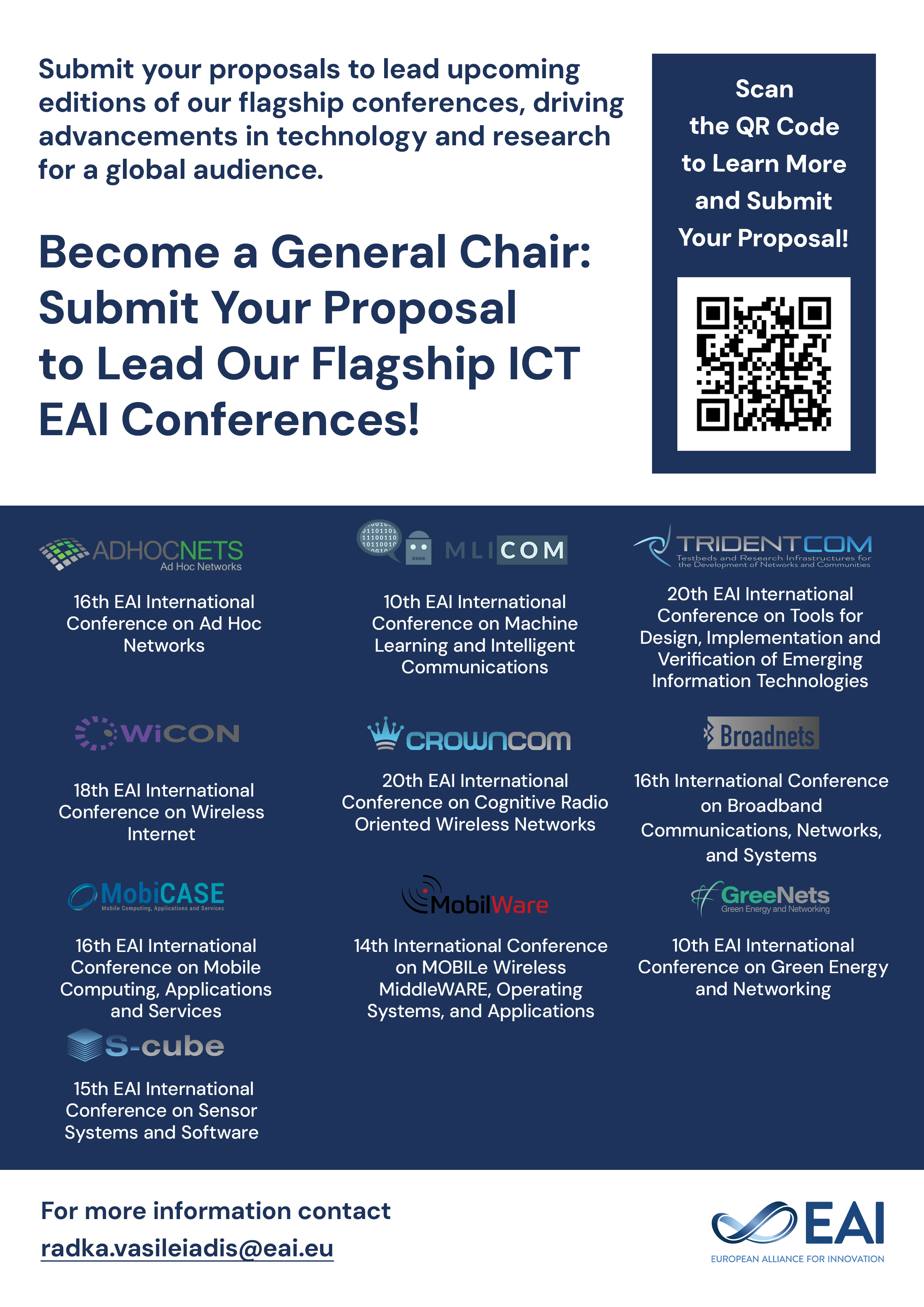
Research Article
Deep Reinforcement Learning-Based Task Offloading and Resource Allocation for Mobile Edge Computing
397 downloads
@INPROCEEDINGS{10.1007/978-3-030-00557-3_4, author={Liang Huang and Xu Feng and Liping Qian and Yuan Wu}, title={Deep Reinforcement Learning-Based Task Offloading and Resource Allocation for Mobile Edge Computing}, proceedings={Machine Learning and Intelligent Communications. Third International Conference, MLICOM 2018, Hangzhou, China, July 6-8, 2018, Proceedings}, proceedings_a={MLICOM}, year={2018}, month={10}, keywords={Mobile edge computing Deep reinforcement learning Task offloading Resource allocation Deep Q-learning}, doi={10.1007/978-3-030-00557-3_4} }
- Liang Huang
Xu Feng
Liping Qian
Yuan Wu
Year: 2018
Deep Reinforcement Learning-Based Task Offloading and Resource Allocation for Mobile Edge Computing
MLICOM
Springer
DOI: 10.1007/978-3-030-00557-3_4
Abstract
We consider a mobile edge computing system that every user has multiple tasks being offloaded to edge server via wireless networks. Our goal is to acquire a satisfactory task offloading and resource allocation decision for each user so as to minimize energy consumption and delay. In this paper, we propose a deep reinforcement learning-based approach to solve joint task offloading and resource allocation problems. Simulation results show that the proposed deep Q-learning-based algorithm can achieve near-optimal performance.
Copyright © 2018–2024 ICST