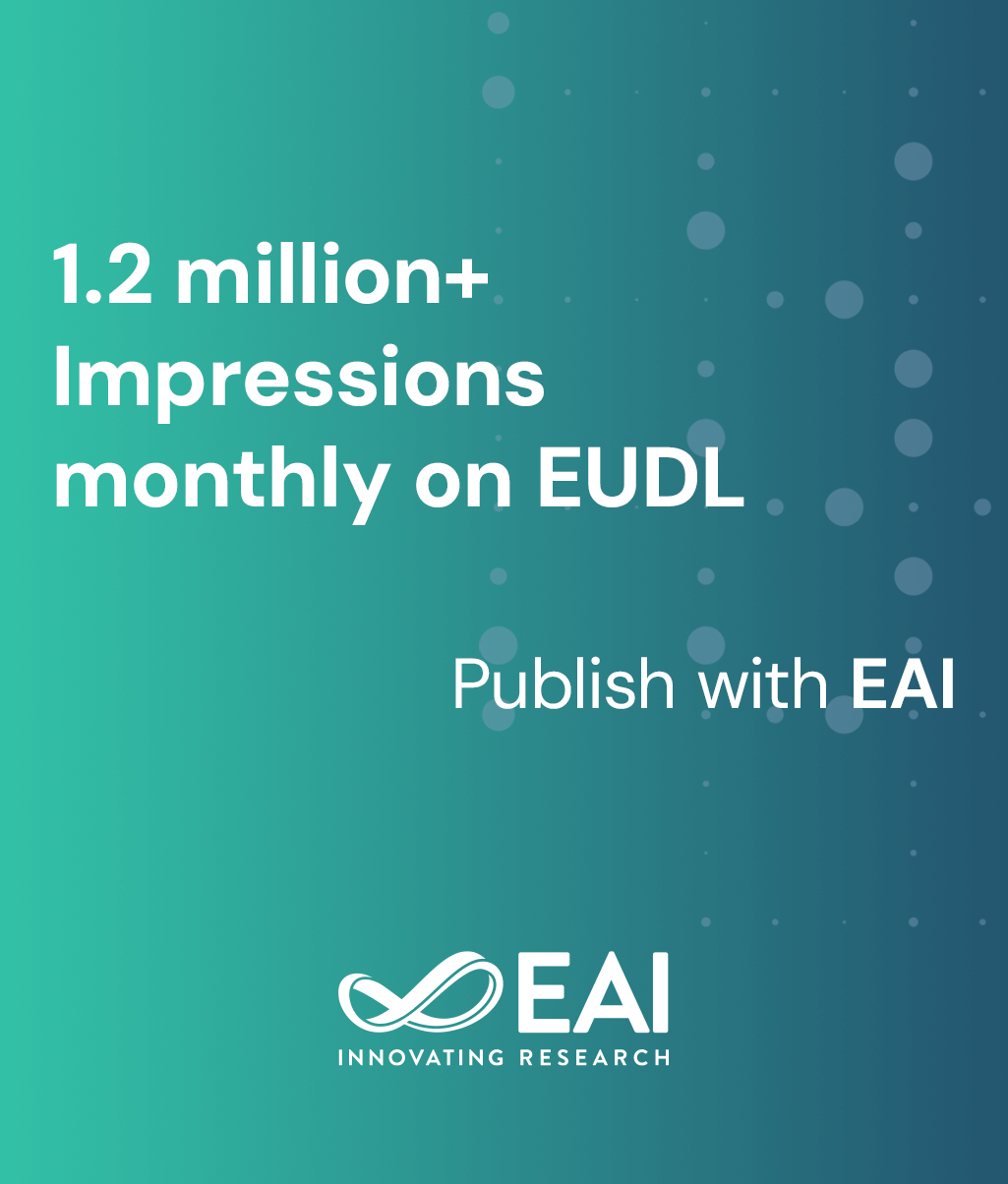
Research Article
Real-Time Drone Detection Using Deep Learning Approach
@INPROCEEDINGS{10.1007/978-3-030-00557-3_3, author={Manjia Wu and Weige Xie and Xiufang Shi and Panyu Shao and Zhiguo Shi}, title={Real-Time Drone Detection Using Deep Learning Approach}, proceedings={Machine Learning and Intelligent Communications. Third International Conference, MLICOM 2018, Hangzhou, China, July 6-8, 2018, Proceedings}, proceedings_a={MLICOM}, year={2018}, month={10}, keywords={Drone detection Deep learning Visual detection}, doi={10.1007/978-3-030-00557-3_3} }
- Manjia Wu
Weige Xie
Xiufang Shi
Panyu Shao
Zhiguo Shi
Year: 2018
Real-Time Drone Detection Using Deep Learning Approach
MLICOM
Springer
DOI: 10.1007/978-3-030-00557-3_3
Abstract
The arbitrary use of drones poses great threat to public safety and personal privacy. It is necessary to detect the intruding drones in sensitive areas in real time. In this paper, we design a real-time drone detector using deep learning approach. Specifically, we improve a well-performed deep learning model, i.e., You Only Look Once, by modifying its structure and tuning its parameters to better accommodate drone detection. Considering that a robust detector needs to be trained using a large amount of training images, we also propose a semi-automatically dataset labelling method based on Kernelized Correlation Filters tracker to speed up the pre-processing of the training images. At last, the performance of our detector is verified via extensive experiments.