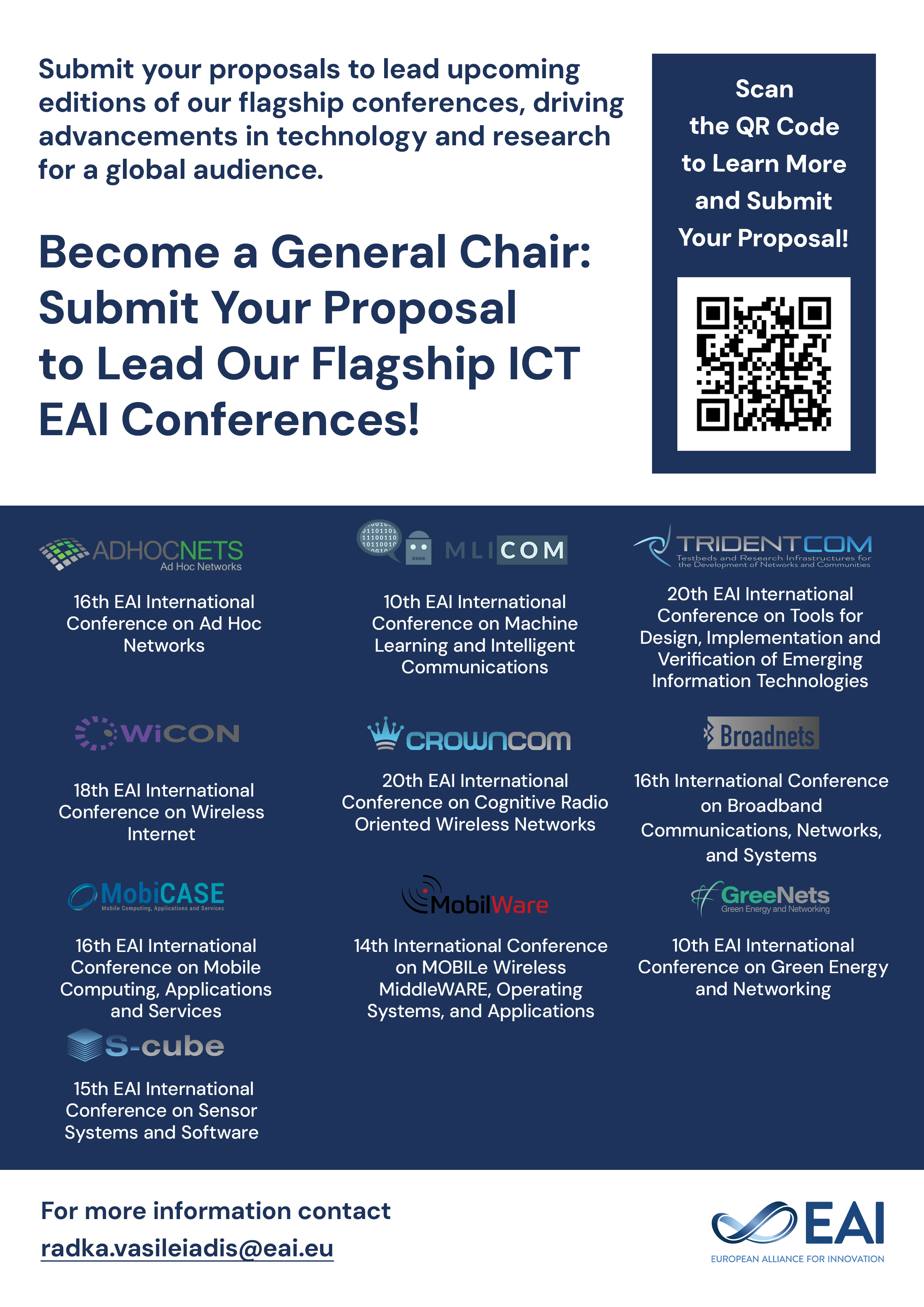
Research Article
Light-Weight Global Feature for Mobile Clothing Search
@INPROCEEDINGS{10.1007/978-3-030-00410-1_41, author={Guangshan Wen and Jing Wu and Chengnian Long and Yi-Bing Lin}, title={Light-Weight Global Feature for Mobile Clothing Search}, proceedings={IoT as a Service. Third International Conference, IoTaaS 2017, Taichung, Taiwan, September 20--22, 2017, Proceedings}, proceedings_a={IOTAAS}, year={2018}, month={10}, keywords={Smartphone Computer vision Flexible object Global feature Threshold-LBP Color histogram Incremental feature}, doi={10.1007/978-3-030-00410-1_41} }
- Guangshan Wen
Jing Wu
Chengnian Long
Yi-Bing Lin
Year: 2018
Light-Weight Global Feature for Mobile Clothing Search
IOTAAS
Springer
DOI: 10.1007/978-3-030-00410-1_41
Abstract
Mobile clothing search on smartphones is extraordinary challenging on both embedded computer vision and networking search system design due to the flexible object, constrained computing, networking resource, and quick response requirement. In this paper, we propose a light-weight global feature LGF – threshold-LBP and color histogram, for mobile clothing search, which is effective for feature extracting and networking communication on smartphone. In addition, an incremental feature is proposed in rerank module to promote the rerank computation and achieve the quick response requirement. Our approach is evaluated through extensive experiments on the smartphones. It reveals that mobile clothing search based on our global feature is more effective than other clothing retrieval system using prevalent local descriptors, such as SIFT, RGB-SIFT.